Unsupervised RGB-T saliency detection by node classification distance and sparse constrained graph learning
APPLIED INTELLIGENCE(2021)
摘要
Saliency detection methods which center on RGB images are sensitive to surrounding environments. Fusing complementary RGB and thermal infrared (RGB-T) images is an effective way to promote the final saliency performance. However, there are relatively few datasets and algorithms for RGB-T saliency detection, which is the prominent problem in this research field. Therefore, an unsupervised method that does not require a large amount of labeled data is proposed for RGB-T image saliency detection in this paper. At first, we construct the graph model in which the superpixels are treated as graph nodes. Instead of utilizing the Euclidean distance to construct initial affinity matrix, a novel node classification distance is designed to explore the local relationship and graph geometrical structure of nodes. Additionally, the advantageous constraint is proposed to increase the sparsity of the image, which not only makes the initial affinity matrix sparse and accurate but also enhances the foreground or background consistency during the graph learning. Furthermore, an adaptive ranking algorithm fusing classification distance and sparse constraint is used to unify the graph affinity learning and the computation of saliency values, which helps to generate more accurate saliency results. Experiments on two public RGB-T datasets demonstrate that the applied method performs desirably against the state-of-the-art algorithms.
更多查看译文
关键词
RGB-T images,Unsupervised saliency detection,Node classification distance,Sparse constrained graph learning
AI 理解论文
溯源树
样例
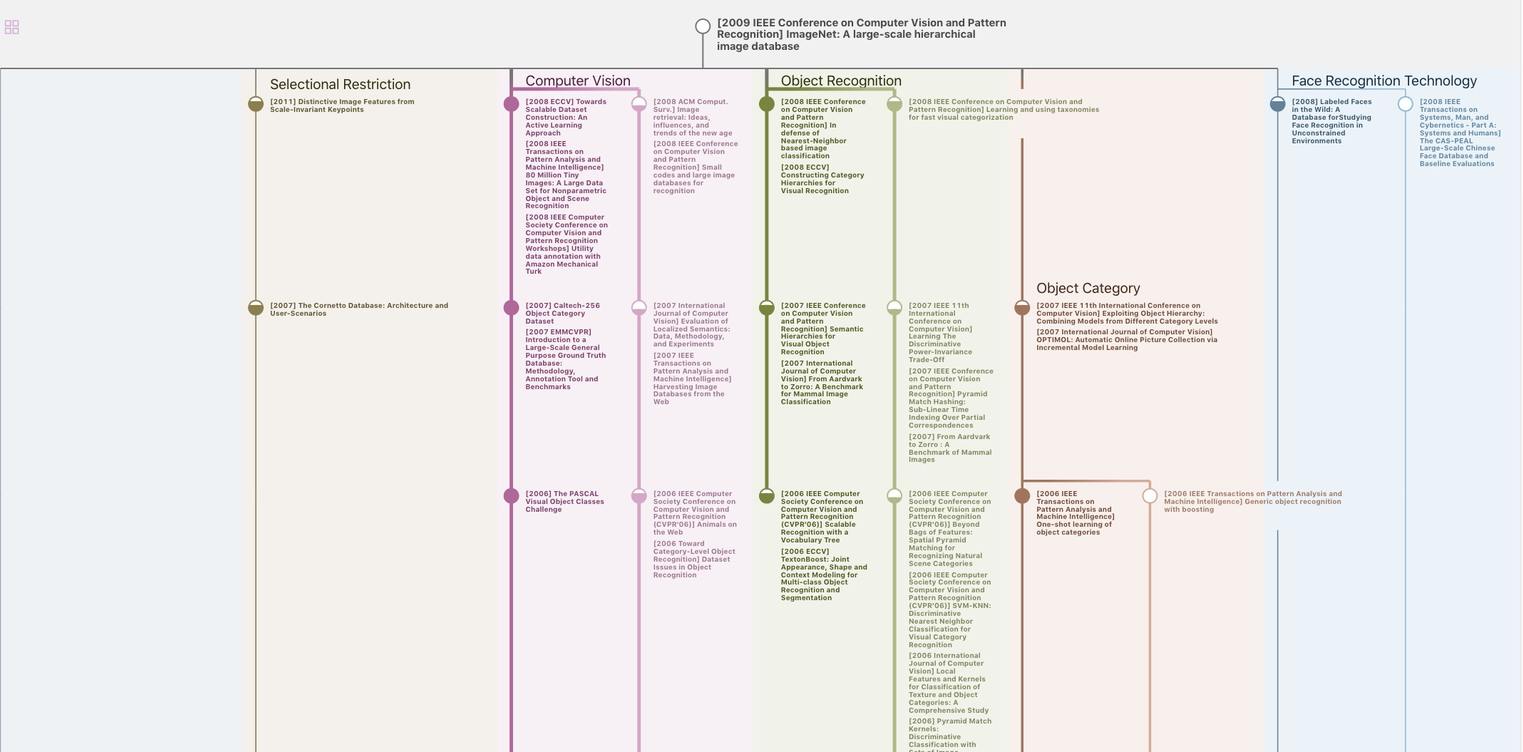
生成溯源树,研究论文发展脉络
Chat Paper
正在生成论文摘要