DM-pruning CADJ graphs for SOM clustering
NEURAL COMPUTING & APPLICATIONS(2021)
摘要
topology representing networks, the Cumulative ADJacency graph CADJ and its symmetric version CONN = CADJ + CADJ^T Tasdemir and Merenyi (IEEE Trans Neural Netw 20(4): 549–562, 2009), can be utilized as inputs to graph-based clustering (GBC) paradigms for partitioning the learned prototypes of a vector quantizer. To express complex data faithfully, CADJ must typically be pruned (thresholded, or made sparse) to be most effective as an input to GBC routines, whether they be algorithmic or driven by human assessment. This work, given in two parts, develops a formal framework for CADJ pruning as a preprocessing (sparsifying) step to improve CADJ’s use in any GBC routine. That is, rather than advocating a particular GBC method, our goal is development of sensible logic for creating sparse CADJ inputs to the entire family of GBC methods. Part 1 defines an overall quality measure for each CADJ edge by extending lines of reasoning used successfully in the past to prune CONN graphs. Part 2 introduces a Bayesian Dirichlet-multinomial (DM) model of CADJ edge weights with an intelligent prior constructed through analysis of the Voronoi tessellation generated by the vector quantization. The DM likelihood offers an internal assessment of information loss resulting from iterative CADJ edge removal, which is used to determine an optimal stopping criterion for the pruning process. We show that DM-Pruned CADJ graphs lead to GBCs comparable to the best previously achieved on highly structured real data.
更多查看译文
关键词
SOM clustering,Topology representing graph,Graph sparsity
AI 理解论文
溯源树
样例
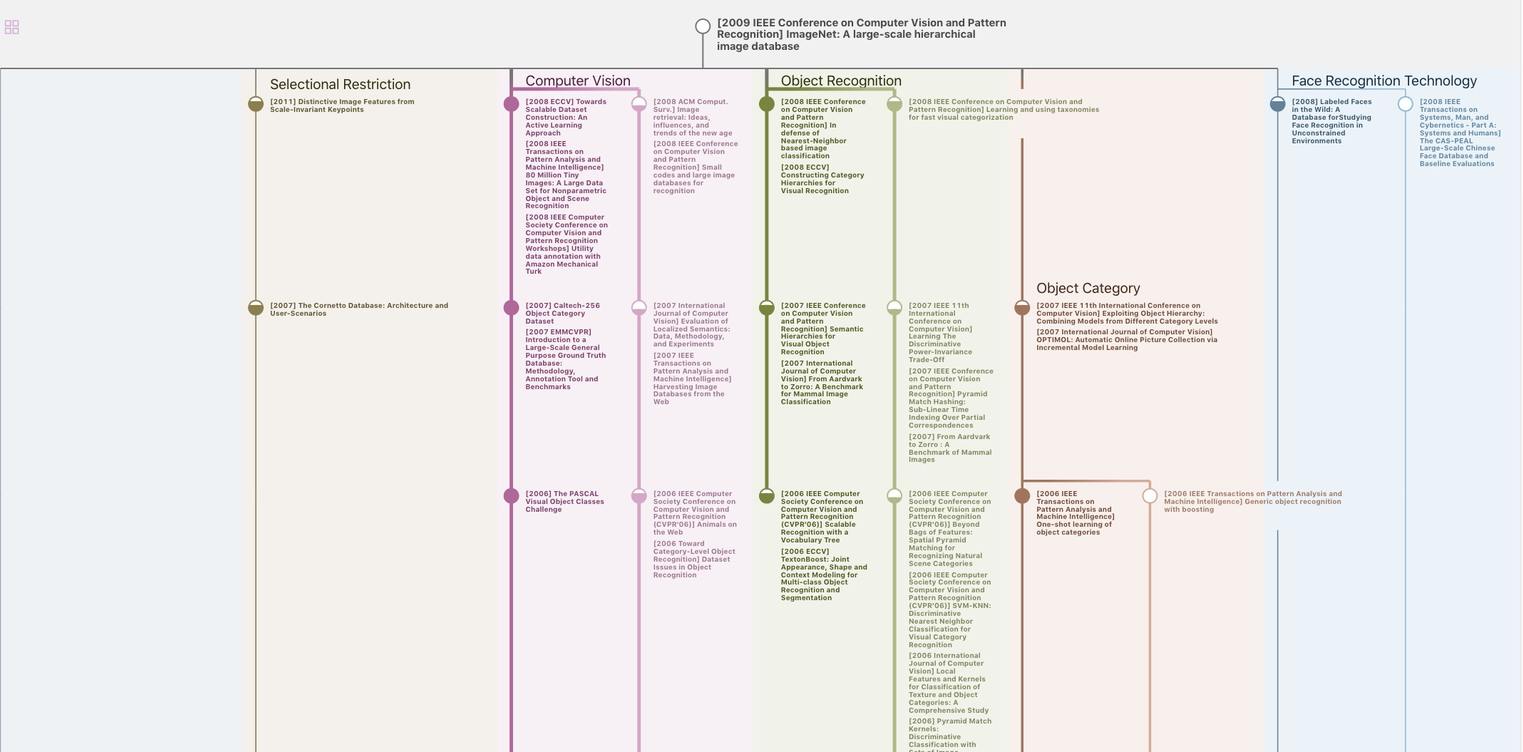
生成溯源树,研究论文发展脉络
Chat Paper
正在生成论文摘要