Learning Saliency-Aware Correlation Filters for Visual Tracking
COMPUTER JOURNAL(2022)
摘要
Recently, visual object tracking has become a hot topic in computer vision community owing to its extensive applications and fundamental research significance. Many researchers have paid attention to the discriminative correlation filter (DCF) due to its excellent tracking performance. The background-aware CF (BACF) is developed to handle the inevitable boundary effects of DCFs that shows superior performance compared with other CF-based trackers. However, BACF has a poor occlusion handling ability. To overcome this defect, we propose a saliency-aware CF tracking (SACF) model. In SACF, a saliency map of the target is introduced on CFs to strengthen the ability to extract the target from a complex background. Meanwhile, to adjust to the rapid variation of the target appearance, we adaptively modify the learning rate of the filter template using adaptive learning rate. By incorporating the saliency map into the BACF objective function and adaptive learning rate adjustment, the robustness and accuracy of the tracker are boosted significantly. Extensive experiments validate that our method can efficaciously solve the occlusion problem and achieves state-of-the-art performance in terms of accuracy and speed on standard benchmarks, i.e. OTB-2013, OTB-2015 and LaSOT. Compared with other state-of-the-art CF tracking methods, the proposed method is competitive considering accuracy and success rate.
更多查看译文
关键词
visual object tracking, correlation filters, saliency map, learning rate
AI 理解论文
溯源树
样例
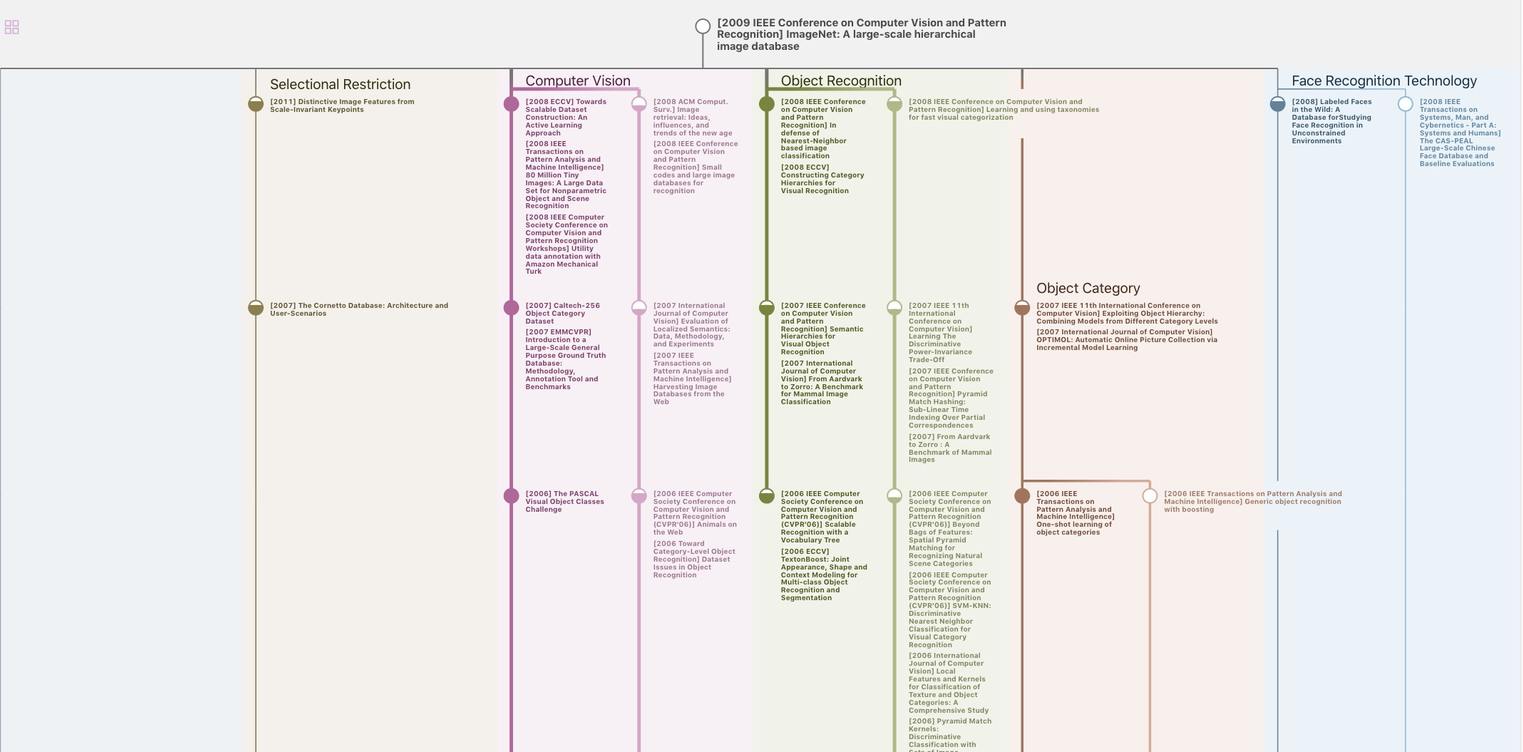
生成溯源树,研究论文发展脉络
Chat Paper
正在生成论文摘要