Res2-UNeXt: a novel deep learning framework for few-shot cell image segmentation
Multimedia Tools and Applications(2021)
摘要
Recently, developing more accurate and more efficient deep learning algorithms for medical images segmentation attracts more and more attentions of researchers. Most of methods increase the depth of the network to replace with acquiring multi-information. The costs of training images annotation are too expensive to label by hand. In this paper, we propose a multi-scale and better performance deep architecture for medical image segmentation, named Res2-UNeXt. Our architecture is an encoder-decoder network with Res2XBlocks. The Res2XBlocks aim at acquiring multi-scale information better in images. To cooperate with Res2-UNeXt, we put forward a simple and efficient method of data augmentation. The data augmentation method, based on the process of cell movement and deformation, has biological implications in away. We evaluate Res2-UNeXt in comparison with recent variants of U-Net: UNet++, CE-Net and LadderNet and the method that different from U-Net architecture: FCN and DFANet on the dataset of ISBI cell tracking challenge 2019 via four different cell images. The experimental results demonstrate that Res2-UNeXt can achieve better performance than both recent variants of U-Net and non-U-Net architecture methods. Besides, the proposed architecture and the data augmentation method have been proven efficiently by the ablation experiments.
更多查看译文
关键词
Segmentation of medical images,Data augmentation,Deep learning,Image processing
AI 理解论文
溯源树
样例
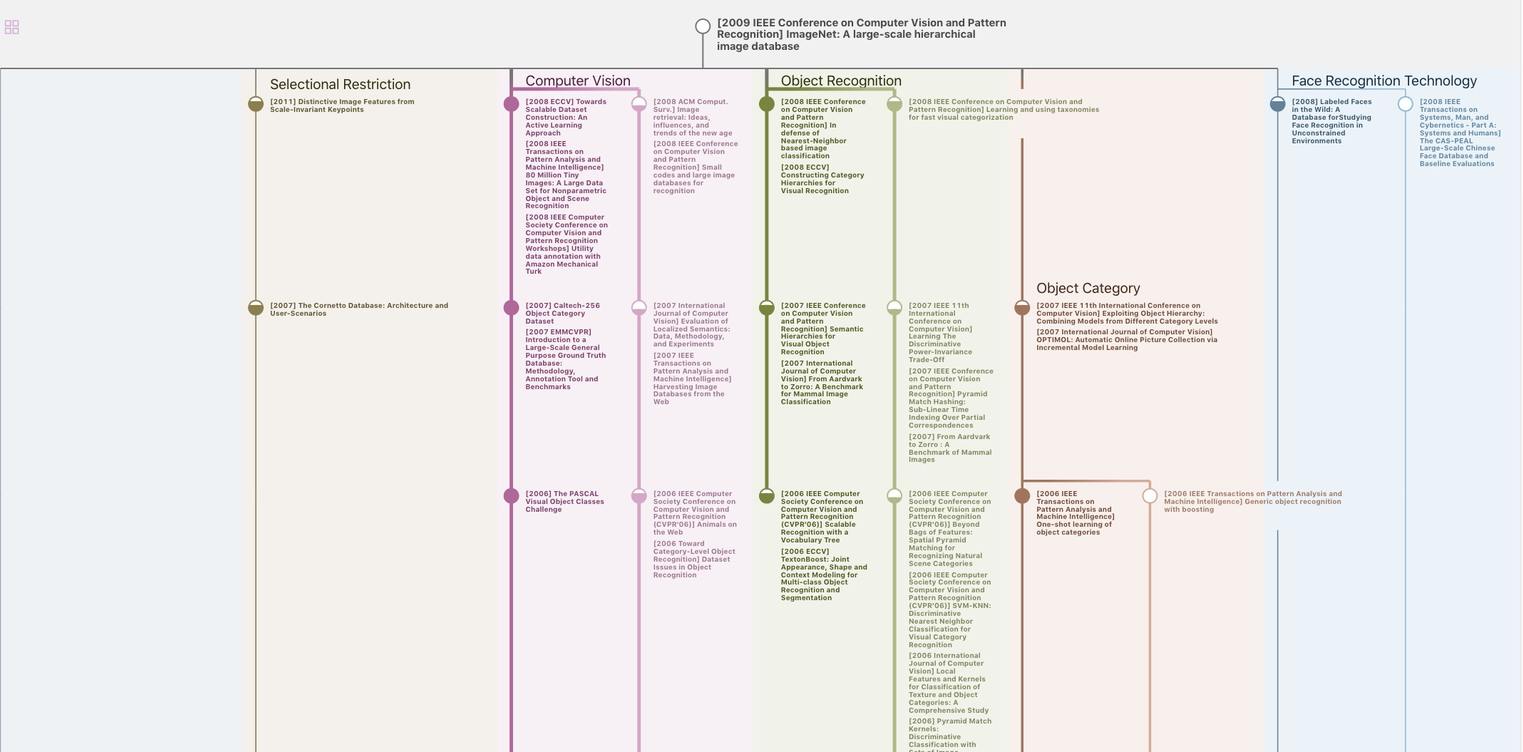
生成溯源树,研究论文发展脉络
Chat Paper
正在生成论文摘要