Maximizing Explainability with SF-Lasso and Selective Inference for Video and Picture Ads
ADVANCES IN KNOWLEDGE DISCOVERY AND DATA MINING, PAKDD 2021, PT I(2021)
摘要
There is a growing interest in explainable machine learning methods. In our investigation, we have collected heterogeneous features from two series of YouTube video ads and seven series of Instagram picture ads to form our datasets. There are two main challenges that we found in analysing such data: i) multicollinearity and ii) infrequent common features. Due to these issues, standard estimation methods, such as OLS, Lasso, and Elastic-net, are only able to find a small number of significant features. This paper proposes a method called Significant Feature Lasso (SF-Lasso) to maximize model explainability by identifying most of the significant features that affect a target outcome (such as online video and picture ad popularity). Experiments show that SF-Lasso is able to identify much more significant features while maintaining similar prediction accuracy as what Lasso and Elastic-net can obtain. The human evaluation shows that SF-Lasso is better at identifying true features that appeal to ad viewers. We also find that the number of significant features is mainly affected by the model size (i.e., the number of active variables) and the correlations among explanatory variables.
更多查看译文
关键词
Explainable models, Lasso, Significance test
AI 理解论文
溯源树
样例
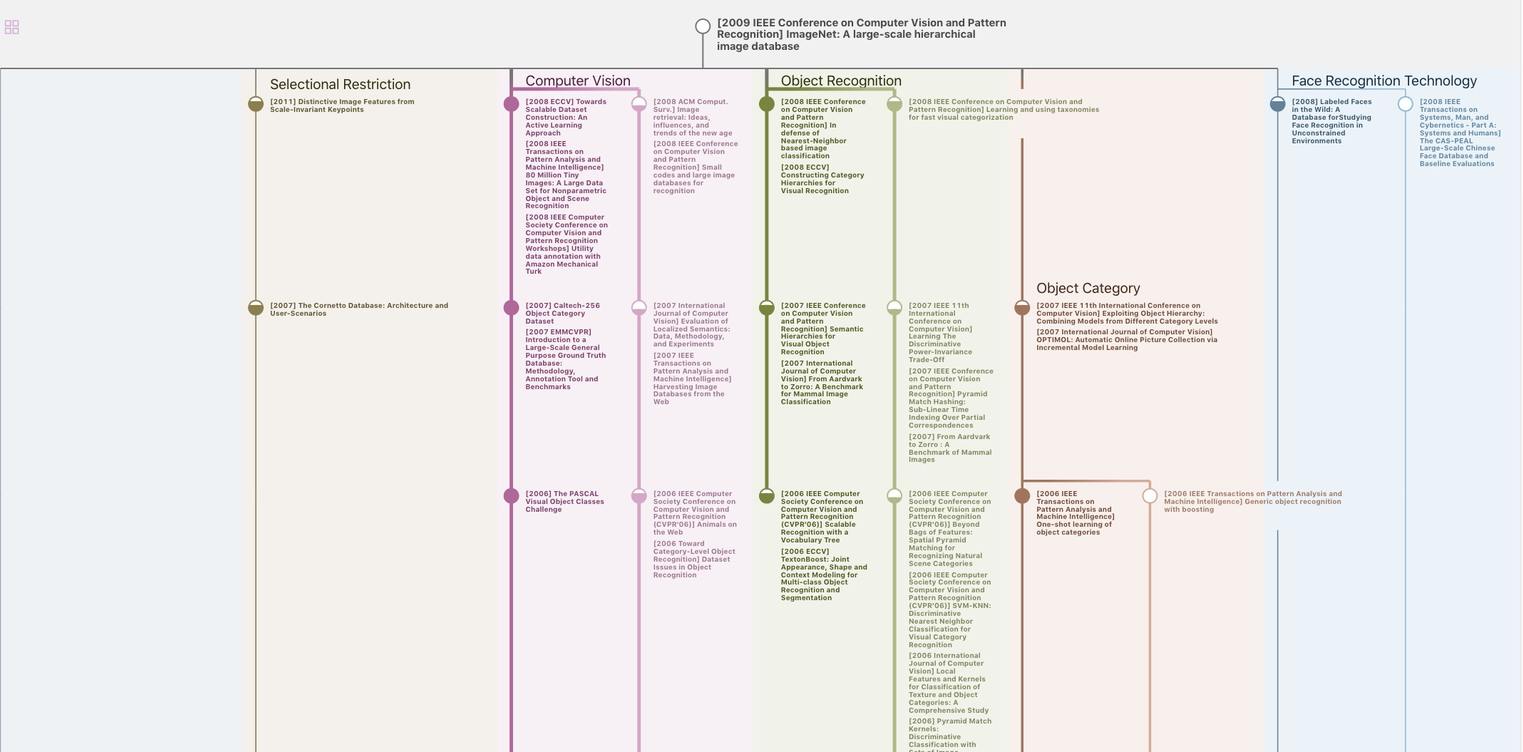
生成溯源树,研究论文发展脉络
Chat Paper
正在生成论文摘要