TRAINING NEURAL NETWORKS WITH DOMAIN PATTERN-AWARE AUXILIARY TASK FOR SLEEP STAGING
2021 IEEE INTERNATIONAL CONFERENCE ON ACOUSTICS, SPEECH AND SIGNAL PROCESSING (ICASSP 2021)(2021)
摘要
Recent studies on deep learning for biomedical data analysis have shown that guiding neural networks (NNs) to incorporate appropriate domain knowledge can enhance model performance. Accordingly, we present an auxiliary classification task for sleep staging to enable NNs to exploit clinically significant EEG patterns in data. Specifically, representations constructed from a global max pooling operator, promoting the NNs to extract distinctive signal patterns for each sleep stage, are adopted herein. While previous studies required additional training processes or parameter tuning phases for correct formulation of the domain knowledge during training, our method operates in an end-to-end manner without further calibration. During the experiments, the proposed method improved the classification accuracies compared to the original model. Moreover, intermediate representations in the proposed method were highly interpretable, wherein medically-significant features were correctly addressed with the activation values. Overall, the proposed method successfully augmented model qualities in two important aspects for adoption of AI in medical tasks: good model performance and clinically sound interpretation of models.
更多查看译文
关键词
neural networks, auxiliary task, sleep staging, EEG analysis, model interpretation
AI 理解论文
溯源树
样例
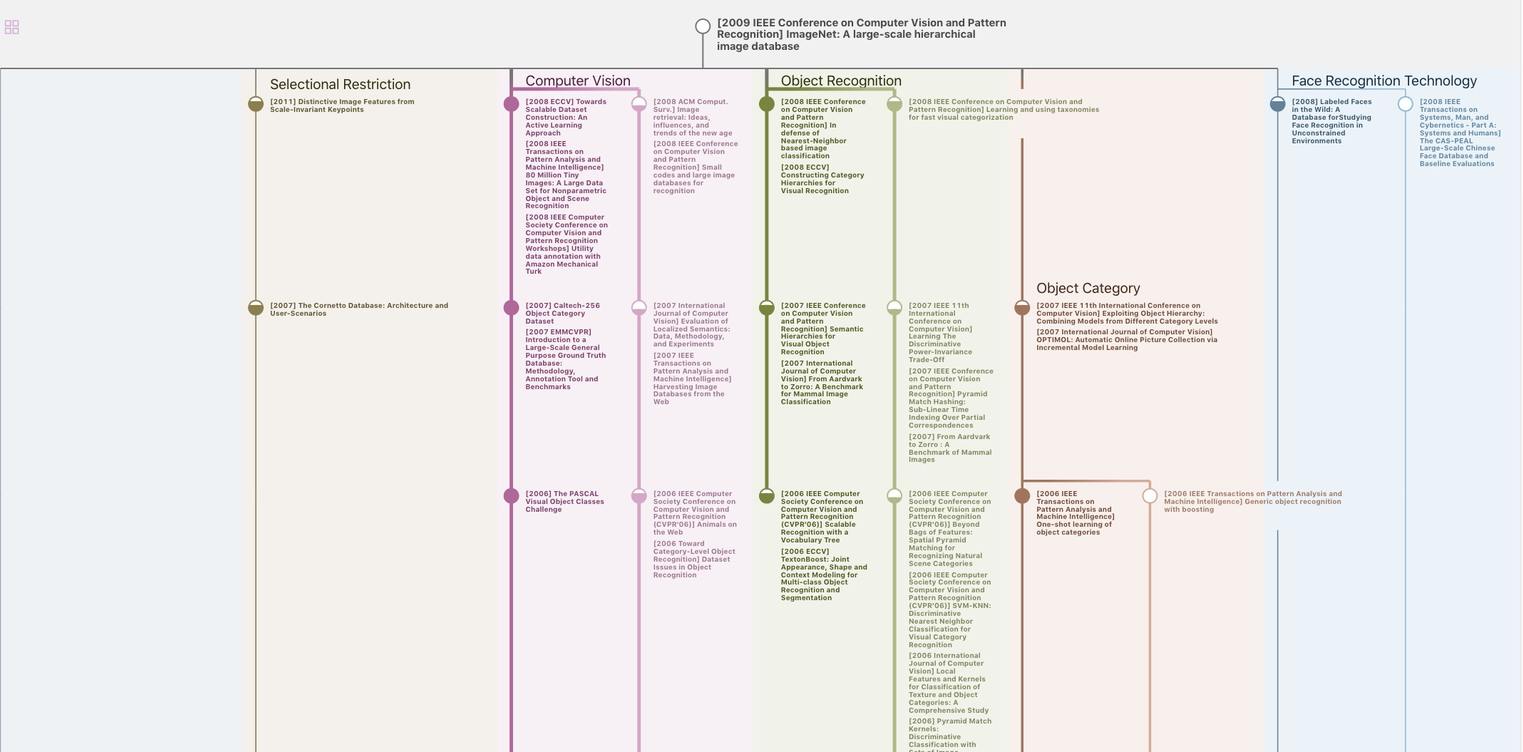
生成溯源树,研究论文发展脉络
Chat Paper
正在生成论文摘要