A JOINT TRAINING FRAMEWORK OF MULTI-LOOK SEPARATOR AND SPEAKER EMBEDDING EXTRACTOR FOR OVERLAPPED SPEECH
2021 IEEE INTERNATIONAL CONFERENCE ON ACOUSTICS, SPEECH AND SIGNAL PROCESSING (ICASSP 2021)(2021)
摘要
In multi-talker cases, overlapped speech degrades the speaker verification (SV) performance dramatically. To tackle this challenging problem, speech separation with multi-channel techniques can be adopted to extract each speaker's signals to improve the SV performance. In this paper, a joint training framework of the front-end multi-look speech separator and the back-end speaker embedding extractor is proposed for multi-channel overlapped speech. To better leverage the complementarity between the speech separator and the speaker embedding extractor, several training strategies are proposed to jointly optimize the two modules. Experimental results show that the proposed joint training framework significantly outperforms the individual SV system by around 52% relative EER reduction. Additionally, the robustness of the proposed framework is further evaluated under different conditions.
更多查看译文
关键词
Speaker verification, multi-channel, multi-look, overlapped speech, speech separation
AI 理解论文
溯源树
样例
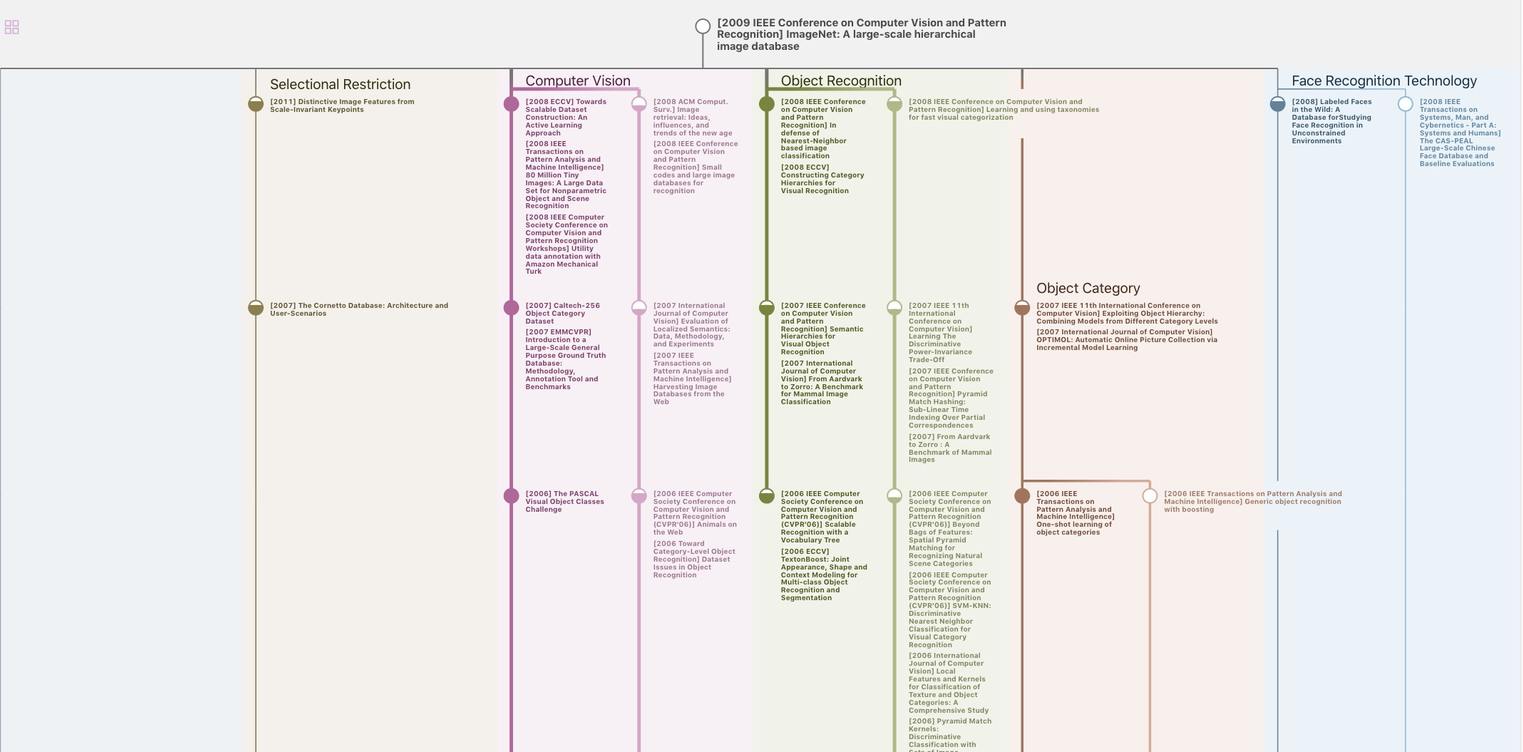
生成溯源树,研究论文发展脉络
Chat Paper
正在生成论文摘要