Efficient Design Of A Dielectric Metasurface With Transfer Learning And Genetic Algorithm
OPTICAL MATERIALS EXPRESS(2021)
摘要
Machine learning has been widely adopted in various disciplines as they offer low-computational cost solutions to complex problems. Recently, deep lear ning-enabled methods for metasurface design have received increasing attention in the field of subwavelength electromagnetics. However, the previous metasurface design methods based on deep learning usually use huge datasets or complex networks to make deep neural networks achieve high prediction accuracy which results in more time for dataset establishment and network training. Here, we propose an expeditious and accurate scheme for designing phase-modulating dielectric metasurface through employing the transfer learning technology and genetic algorithm. The performance of the neural network is improved distinctly by migrating knowledge between real part and imaginary part spectrum-prediction tasks. Furthermore, the target meta-atoms can be optimized readily without increasing a large dataset through transfer learning. Finally, we design two deflectors and two metalenses as a proof-of-concept demonstration to validate the ability of our proposed approach. The scheme provides an efficient and promising design method for phase-modulating metasurface. (c) 2021 Optical Society of America under the terms of the OSA Open Access Publishing AgreementMetasurface, a two-dimension artificial subwavelength structure, can arbitrarily manipulate the amplitude, phase, and polarization of the electromagnetic (EM) wave. It has tremendous potential to replace traditional optical components due to their advantages of low profile and ease of integration [1-6]. The traditional design method of metasurface is a one-time and trial-and-error process with complicated electromagnetic simulations. The general design philosophy is that the optimization algorithm combines with the electromagnetic simulation tool (e.g. finite-element method (FEM) and rigorous coupled-wave analysis (RCWA)) to search for the best result under the guidance of the gradient or fitness [7,8]. For example, the topology optimization method obtains the optimal result by updating the parameters along the deepest-gradient direction, which is calculated through the corresponding adjoint problem [9-11]. Nevertheless, most traditional
更多查看译文
AI 理解论文
溯源树
样例
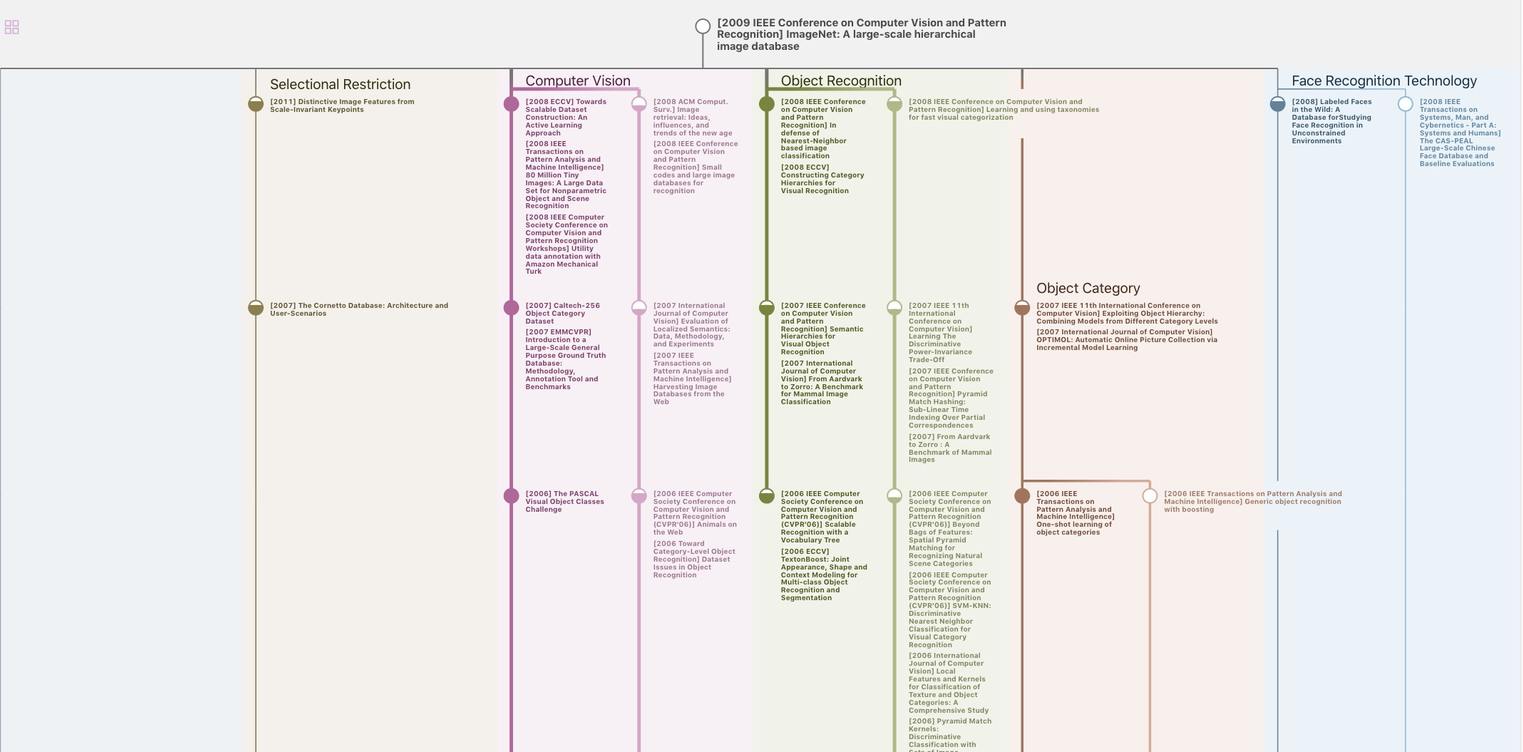
生成溯源树,研究论文发展脉络
Chat Paper
正在生成论文摘要