Transfer Learning And Performance Enhancement Techniques For Deep Semantic Segmentation Of Built Heritage Point Clouds
VIRTUAL ARCHAEOLOGY REVIEW(2021)
摘要
The growing availability of three-dimensional (3D) data, such as point clouds, coming from Light Detection and Ranging (LiDAR), Mobile Mapping Systems (MMSs) or Unmanned Aerial Vehicles (UAVs), provides the opportunity to rapidly generate 3D models to support the restoration, conservation, and safeguarding activities of cultural heritage (CH). The so-called scan-to-BIM process can, in fact, benefit from such data, and they can themselves be a source for further analyses or activities on the archaeological and built heritage. There are several ways to exploit this type of data, such as Historic Building Information Modelling (HBIM), mesh creation, rasterisation, classification, and semantic segmentation. The latter, referring to point clouds, is a trending topic not only in the CH domain but also in other fields like autonomous navigation, medicine or retail. Precisely in these sectors, the task of semantic segmentation has been mainly exploited and developed with artificial intelligence techniques. In particular, machine learning (ML) algorithms, and their deep learning (DL) subset, are increasingly applied and have established a solid state-of-the-art in the last half-decade. However, applications of DL techniques on heritage point clouds are still scarce; therefore, we propose to tackle this framework within the built heritage field. Starting from some previous tests with the Dynamic Graph Convolutional Neural Network (DGCNN), in this research close attention is paid to: i) the investigation of fine-tuned models, used as a transfer learning technique, ii) the combination of external classifiers, such as Random Forest (RF), with the artificial neural network, and iii) data augmentation results evaluation for the domain-specific ArCH dataset. Finally, after analysing the main advantages and critical aspects, a proposal is made evaluating the extent to which this methodology can also be useful for non-programming or domain experts.
更多查看译文
关键词
cultural heritage, semantic segmentation, deep learning, deep neural networks, point clouds
AI 理解论文
溯源树
样例
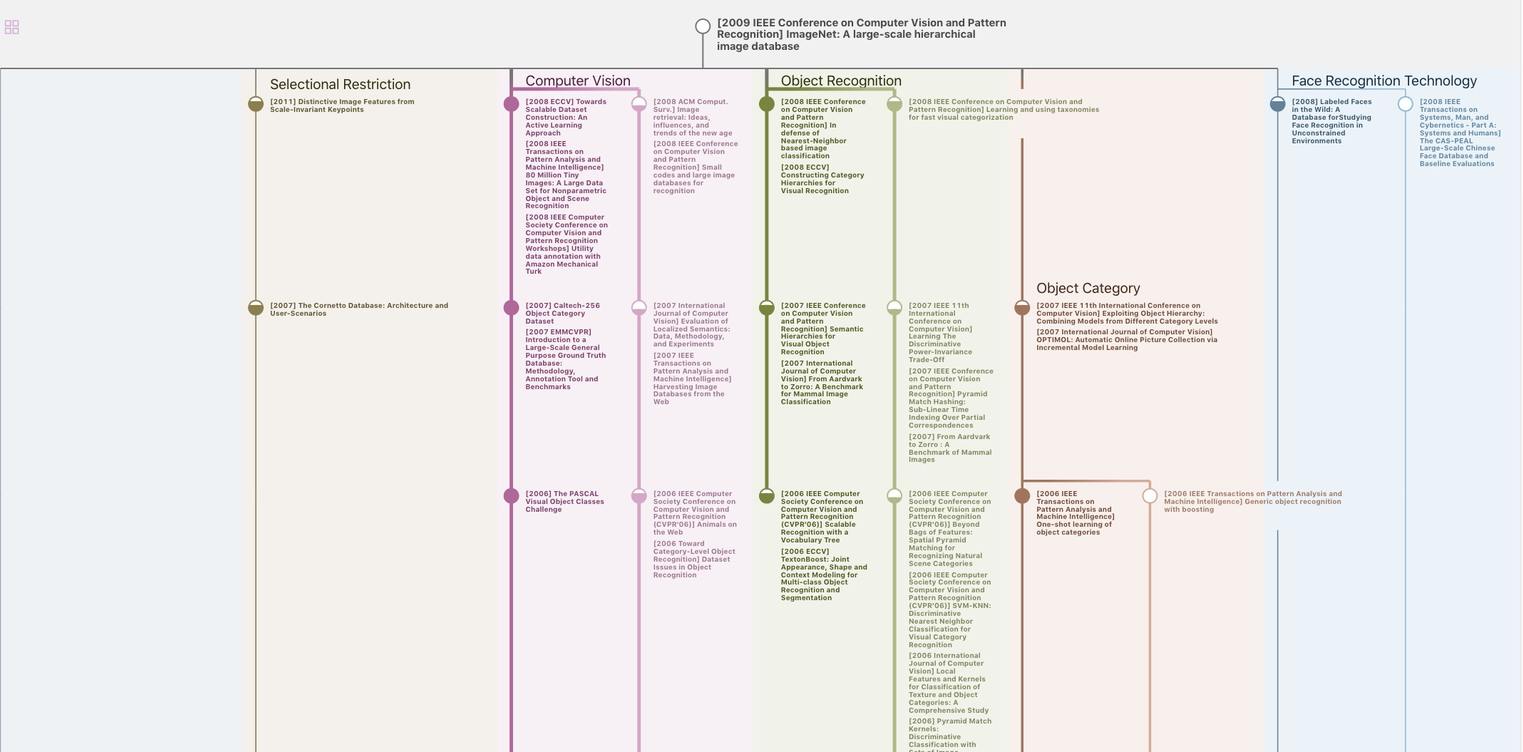
生成溯源树,研究论文发展脉络
Chat Paper
正在生成论文摘要