Rubik: A Hierarchical Architecture for Efficient Graph Neural Network Training
IEEE Transactions on Computer-Aided Design of Integrated Circuits and Systems(2022)
摘要
The graph convolutional network (GCN) emerges as a promising direction to learn the inductive representation in graph data commonly used in widespread applications, such as E-commerce, social networks, and knowledge graphs. However, learning from graphs is nontrivial because of its mixed computation model involving both graph analytics and neural network computing. To this end, we decompose the GC...
更多查看译文
关键词
Task analysis,Computational modeling,Hardware,Aggregates,Training,Optimization,Topology
AI 理解论文
溯源树
样例
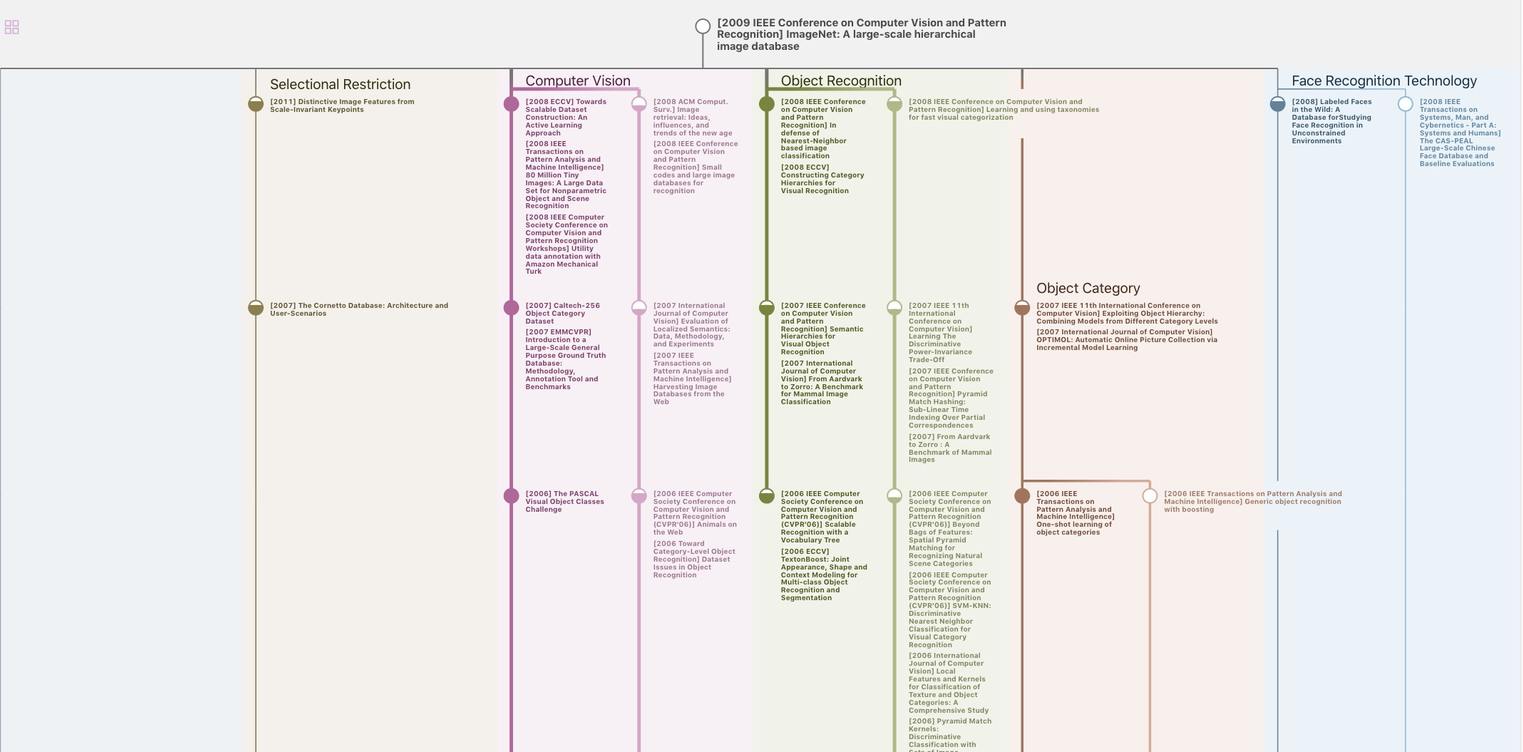
生成溯源树,研究论文发展脉络
Chat Paper
正在生成论文摘要