Generalized Radiative Transfer Emulation For Imaging Spectroscopy Reflectance Retrievals
REMOTE SENSING OF ENVIRONMENT(2021)
摘要
Estimates of surface reflectance from observed radiance are fundamentally tied to the accuracy of the radiative transfer models used to simulate the interaction of light with the atmosphere and surface. These radiative transfer models are parameterized by a wide range of quantities, ranging from observation and solar geometries to assumptions about atmospheric conditions, including vertical distributions aerosols and water vapor as well as the atmospheric composition. However, current retrieval approaches cannot represent this variability; they are limited by computational expense to a coarsely-spaced look up table (LUT) of just a few parameters. Here, we propose a new method called sRTMnet that facilitates the efficient creation of dense LUT grids that fully capture radiative transfer's inherent nonlinearity. sRTMnet uses a combination of fast, reduced-order radiative transfer modeling coupled with neural-network-based emulation to realize a computational speedup of over 3000x while maintaining the accuracy of a high-fidelity RTM. We demonstrate the accuracy of sRTMnet in multiple ways. We show that neural-network-based emulation delivers accurate atmospheric properties using robust testing sets. We then use acquisitions from the airborne visible/infrared imaging spectrometer - next generation (AVIRIS-NG) to show near identical surface reflectance estimates with sRTMnet and a high-fidelity radiative transfer model (MODTRAN). Finally, we show how distributions of mapped minerals remain consistent when using the sRTMnet-based reflectance. Given these results, sRTMnet will be utilized for the upcoming Earth Surface Mineral Dust Source Investigation (EMIT, an imaging spectrometer destined for the international space station in 2022). In addition to speed and accuracy, the fully open-source nature of sRTMnet (including simulation and emulation), provides a pathway for high-resolution imaging spectroscopy reflectance retrievals at the global scale.
更多查看译文
关键词
RTM, AVIRIS, Hyperspectral, Earth surface mineral dust source investigation (EMIT)
AI 理解论文
溯源树
样例
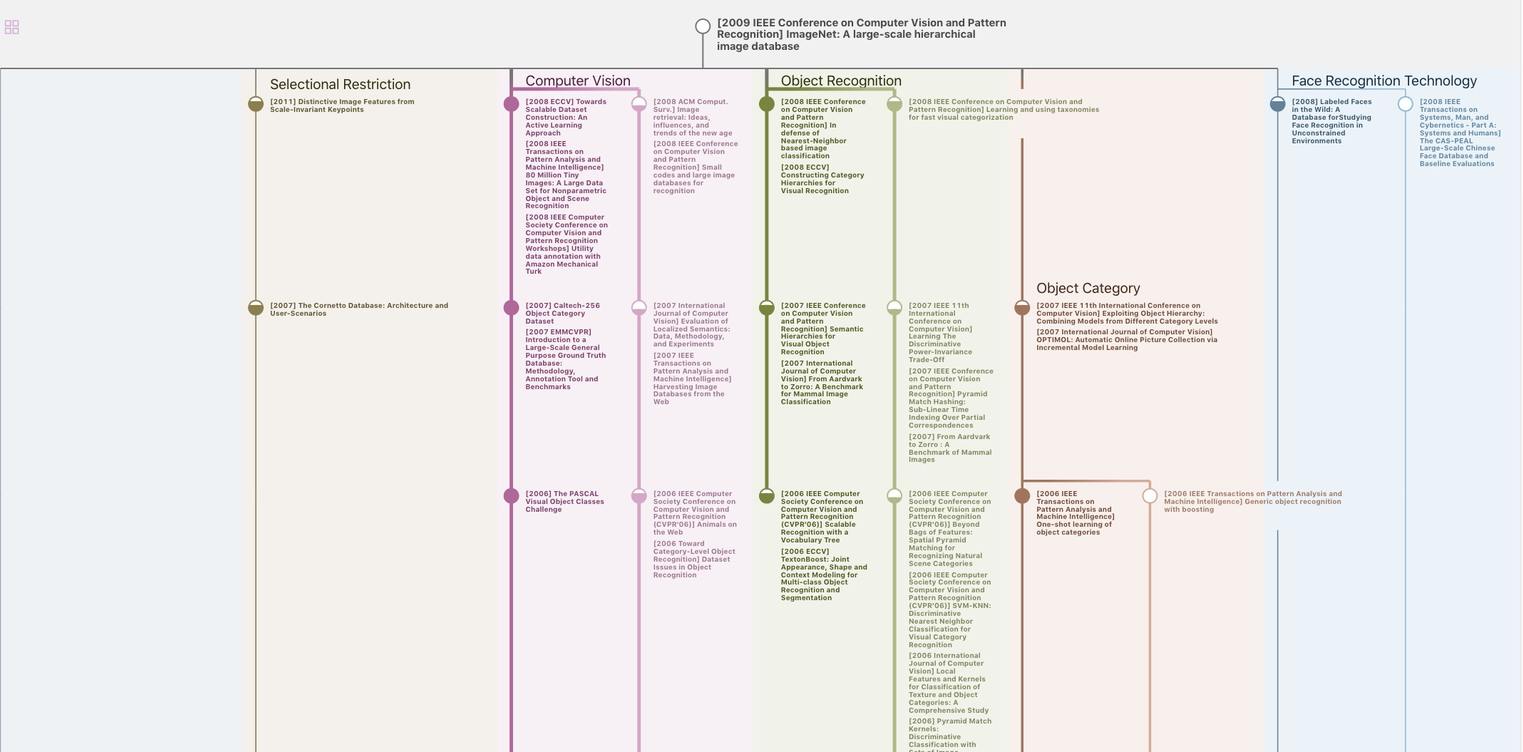
生成溯源树,研究论文发展脉络
Chat Paper
正在生成论文摘要