Dimension Selected Subspace Clustering
2021 IEEE INTERNATIONAL CONFERENCE ON ACOUSTICS, SPEECH AND SIGNAL PROCESSING (ICASSP 2021)(2021)
摘要
Subspace clustering is a popular method for clustering unlabelled data. However, the computational cost of the subspace clustering algorithm can be unaffordable when dealing with a large data set. Using a set of dimension sketched data instead of the original data set can be helpful for mitigating the computational burden. Thus, finding a way for dimension sketching becomes an important problem. In this paper, a new dimension sketching algorithm is proposed, which aims to select informative dimensions that have significant effects on the clustering results. Experimental results reveal that this method can significantly improve subspace clustering performance on both synthetic and real-world datasets, in comparison with two baseline methods.
更多查看译文
关键词
unlabelled data,computational cost,dimension sketched data,original data set,computational burden,dimension sketching algorithm,informative dimensions,subspace clustering performance,real-world datasets,baseline methods
AI 理解论文
溯源树
样例
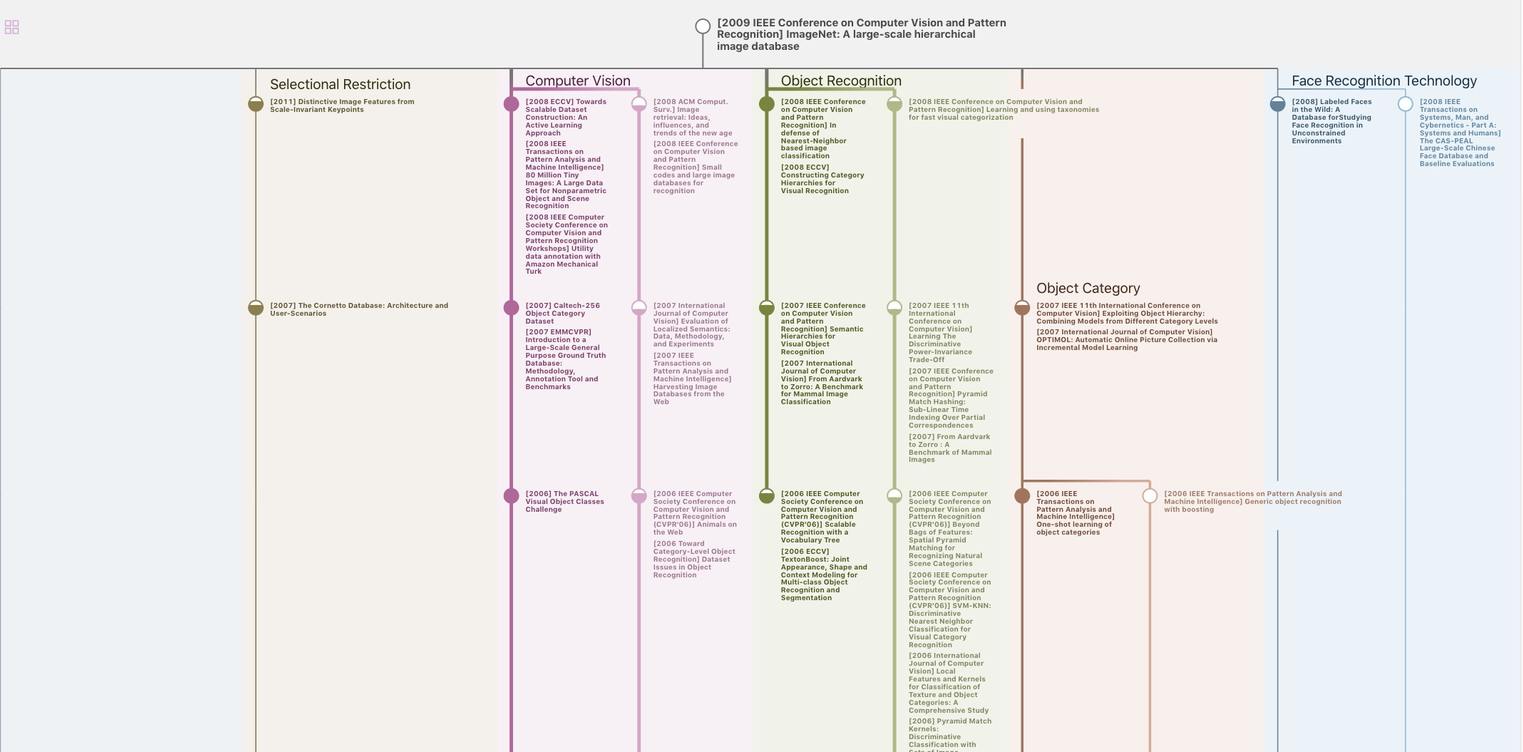
生成溯源树,研究论文发展脉络
Chat Paper
正在生成论文摘要