Online multi-object tracking using multi-function integration and tracking simulation training
APPLIED INTELLIGENCE(2021)
摘要
Recently, with the development of deep-learning, the performance of multi-object tracking algorithms based on deep neural networks has been greatly improved. However, most methods separate different functional modules into multiple networks and train them independently on specific tasks. When these network modules are used directly, they are not compatible with each other effectively, nor can they be better adapted to the multi-object tracking task, which leads to a poor tracking effect. Therefore, a network structure is designed to aggregate the regression of objects between frames and the extraction of appearance features into one model to improve the harmony between various functional modules of multi-object tracking. To improve the support for the multi-object tracking task, an end-to-end training method is also proposed to simulate the multi-object tracking process during the training and expand the training data by using the historical position of the target combined with the prediction of the motion model. A metric loss that can take advantage of the historical appearance features of the target is also used to train the extraction module of appearance features to improve the temporal correlation of extracted appearance features. Evaluation results on the MOTChallenge benchmark datasets show that the proposed approach achieves state-of-the-art performance.
更多查看译文
关键词
Deep learning,Neural network,Computer vision,Multi-object tracking,Object detection
AI 理解论文
溯源树
样例
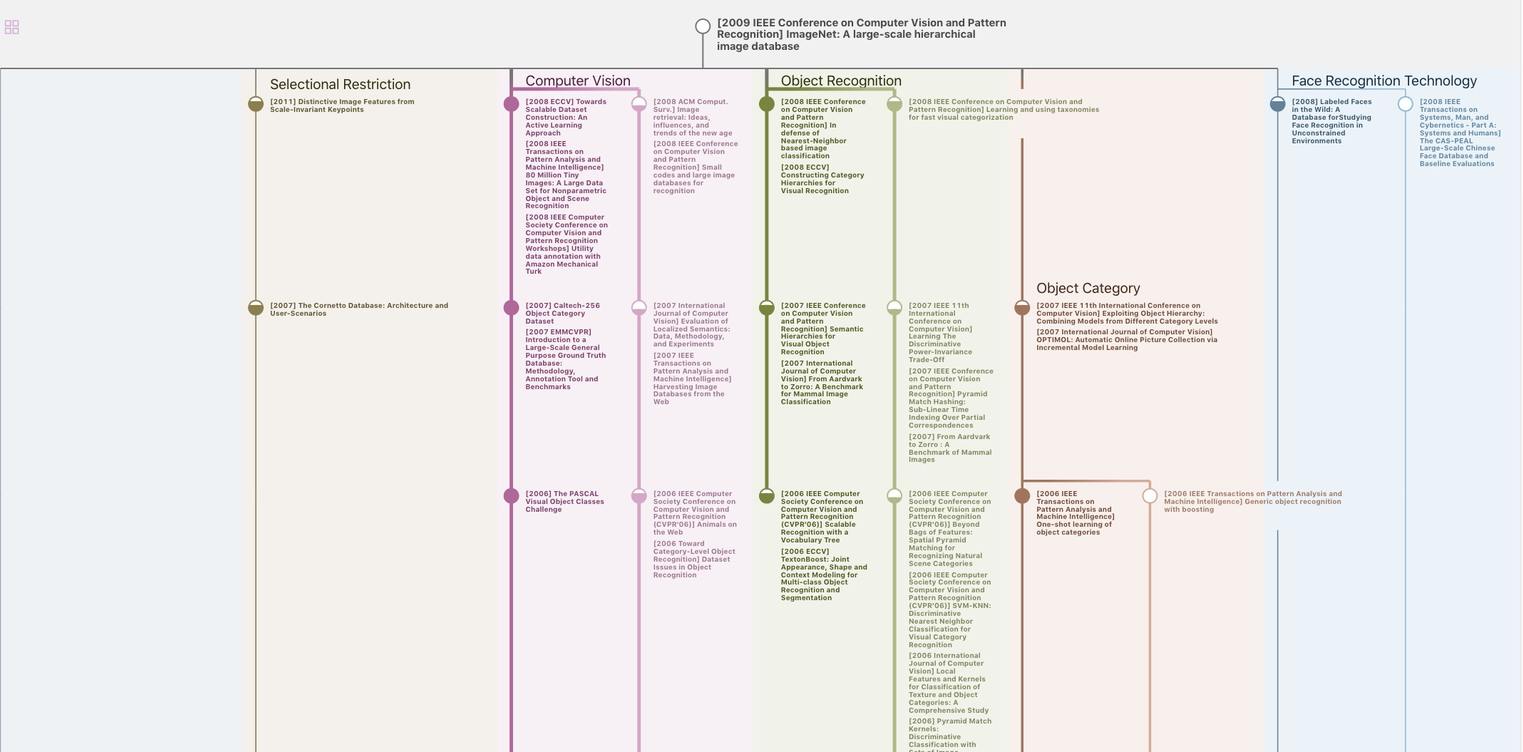
生成溯源树,研究论文发展脉络
Chat Paper
正在生成论文摘要