ANDREAS HOLZINGER – FIVE PUBLICATIONS AND WHY THEY ARE RELEVANT The interactive machine learning concept and four experimental proofs-of-concept
semanticscholar(2018)
摘要
Concept Paper #1: Andreas Holzinger 2016. Interactive Machine Learning for Health Informatics: When do we need the human-in-theloop? Springer/Nature Brain Informatics, 3, 2, 119-131, doi:10.1007/s40708-016-0042-6. https://link.springer.com/article/10.1007/s40708-016-0042-6 This is the concept paper behind the interactive machine learning approach; along with it there are four proof-of-concepts. Since its release two years ago it received 145 citations and 15,000 downloads! The topic was selected as “Aktuelles Schlagwort” by the GI Proof-of-concept #2: Michael Hund, Dominic Boehm, Werner Sturm, Michael Sedlmair, Tobias Schreck, Torsten Ullrich, Daniel A. Keim, Ljiljana Majnaric & Andreas Holzinger 2016. Visual analytics for concept exploration in subspaces of patient groups: Making sense of complex datasets with the Doctor-in-the-loop. Springer/Nature Brain Informatics, 3, 4, 233-247, doi:10.1007/s40708-016-0043-5. https://link.springer.com/article/10.1007/s40708-016-0043-5 In machine learning we are often confronted with data in arbitrarily high dimensional spaces and we must map it down to dimensions perceivable to the human. Irrelevant, redundant, and conflicting dimensions can negatively affect effectiveness and efficiency of the analytic process (curse of dimensionality). In this paper we present a new method to visually explore subspace clusters from different perspectives and introduce a novel analysis workflow. Proof-of-concept #3: Peter Kieseberg, Bernd Malle, Peter Fruehwirt, Edgar Weippl & Andreas Holzinger 2016. A tamper-proof audit and control system for the doctor in the loop. Springer/Nature Brain Informatics, 3, 4, 269– 279, doi:10.1007/s40708-016-0046-2. https://link.springer.com/article/10.1007%2Fs40708-016-0046-2 While the interactive machine learning approach can be beneficial for solving various problems in different domains, it relies heavily on the authenticity of the data, thus there is urgent need for secure and reliable databases. Here, we provide a novel solution to protect a doctor in the loop against manipulated data. Proof-of-concept #4: Seid Muhie Yimam, Chris Biemann, Ljiljana Majnaric, Šefket Šabanović & Andreas Holzinger 2016. An adaptive annotation approach for biomedical entity and relation recognition. Brain Informatics, 3, (3), 157-168, doi:10.1007/s40708-016-0036-4. https://link.springer.com/article/10.1007%2Fs40708-016-0036-4 In three experiments we explore the annotation of semantic relations with relation instance learning across documents. Our experiments validated the interactive machine learning method both qualitatively and quantitatively, and give rise to a personalized, responsive information extraction technology. Proof-of-concept #5: Andreas Holzinger, Markus Plass, Katharina Holzinger, Gloria Cerasela Crisan, Camelia-M. Pintea & Vasile Palade 2016. Towards interactive Machine Learning: Applying Ant Colony Algorithms to solve the Traveling Salesman Problem with the Human-in-the-Loop approach. Springer Lecture Notes in Computer Science LNCS 9817. Heidelberg, Berlin, New York: Springer, pp. 8195, doi:10.1007/978-3-319-45507-56. https://link.springer.com/chapter/10.1007/978-3-319-45507-5_6 Here we show on a gamification experiment how to open a black box, thus enabling a human directly and indirectly interacting with an algorithm. We selected the Ant Colony Optimization (ACO) framework and use it on the Traveling Salesman Problem (TSP) which is of high importance in solving many practical problems in practice, e.g. in health informatics, in the study of proteins. I have a good track record in gamification, e.g. my first gamification paper received 606 citations!
更多查看译文
AI 理解论文
溯源树
样例
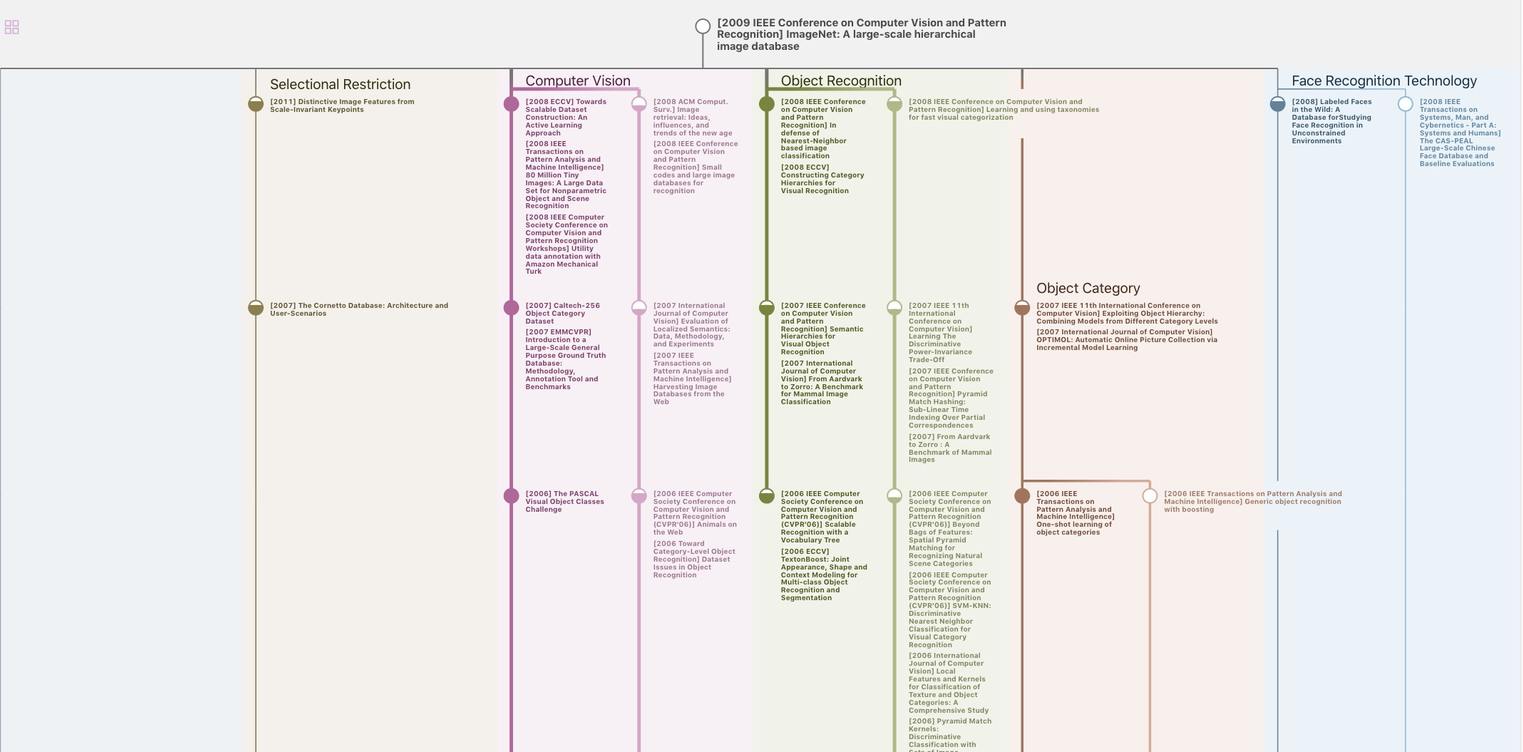
生成溯源树,研究论文发展脉络
Chat Paper
正在生成论文摘要