Transport-based pattern recognition versus deep neural networks in underwater OAM communications
JOURNAL OF THE OPTICAL SOCIETY OF AMERICA A-OPTICS IMAGE SCIENCE AND VISION(2021)
Abstract
Comparisons between machine learning and optimal transport-based approaches in classifying images are made in underwater orbital angular momentum (OAM) communications. A model is derived that justifies optimal transport for use in attenuated water environments. OAM pattern demultiplexing is performed using optimal transport and deep neural networks and compared to each other. Additionally, some of the complications introduced by signal attenuation are highlighted. The Radon cumulative distribution transform (R-CDT) is applied to OAM patterns to transform them to a linear subspace. The original OAM images and the R-CDT transformed patterns are used in several classification algorithms, and results are compared. The selected classification algorithms are the nearest subspace algorithm, a shallow convolutional neural network (CNN), and a deep neural network. It is shown that the R-CDT transformed images are more accurate than the original OAM images in pattern classification. Also, the nearest subspace algorithm performs better than the selected CNNs in OAM pattern classification in underwater environments. (c) 2021 Optical Society of America under the terms of the OSA Open Access Publishing Agreement
MoreTranslated text
Key words
deep neural networks,communications,pattern recognition,neural networks,transport-based
AI Read Science
Must-Reading Tree
Example
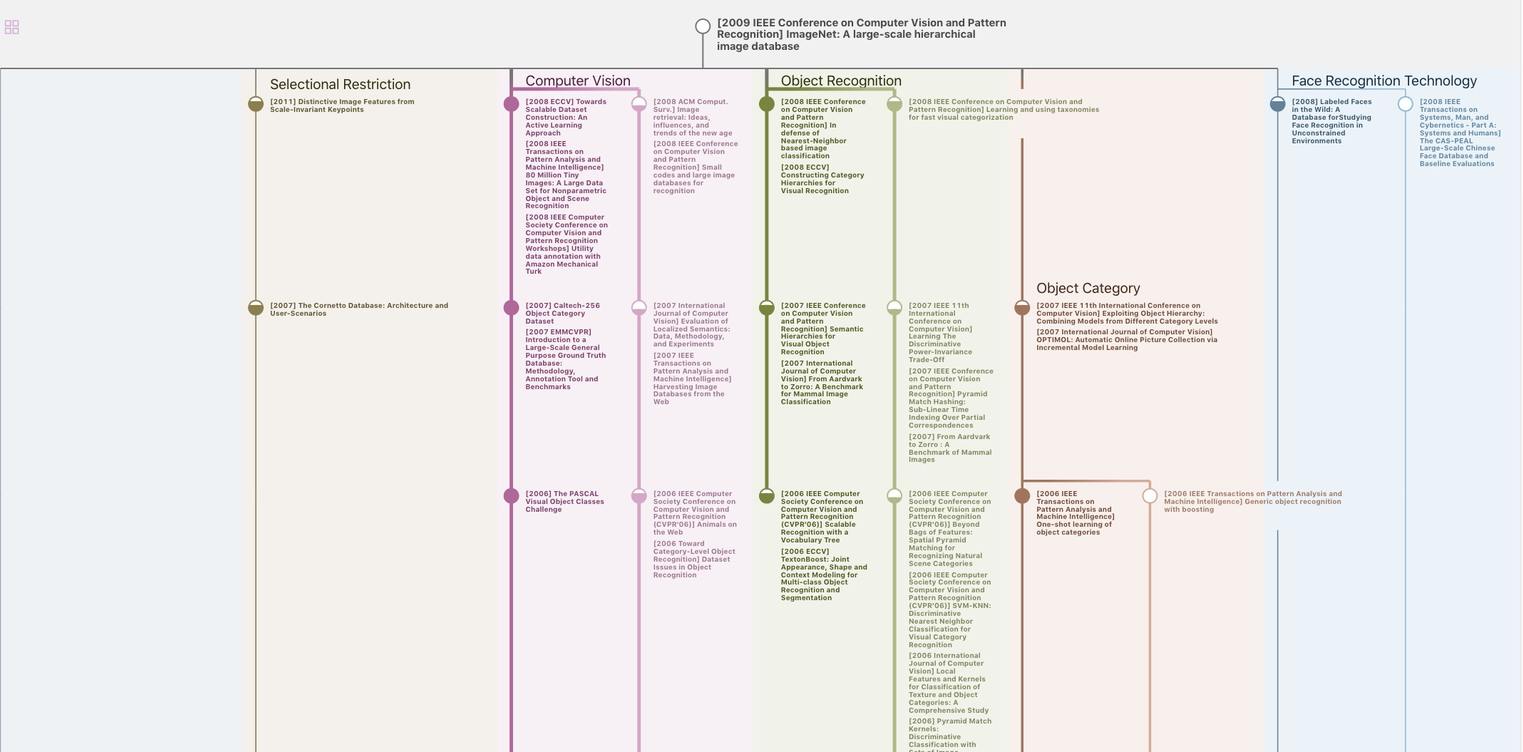
Generate MRT to find the research sequence of this paper
Chat Paper
Summary is being generated by the instructions you defined