Adaptive Importance Sampling Via Auto-Regressive Generative Models and Gaussian Processes
2021 IEEE INTERNATIONAL CONFERENCE ON ACOUSTICS, SPEECH AND SIGNAL PROCESSING (ICASSP 2021)(2021)
摘要
The quality of importance distribution is vital to adaptive importance sampling, especially in high dimensional sampling spaces where the target distributions are sparse and hard to approximate. This requires that the proposal distributions are expressive and easily adaptable. Because of the need for weight calculation, point evaluation of the proposal distributions is also needed. The Gaussian process has been proven to be a highly expressive non-parametric model for conditional density estimation whose training process is also straightforward. In this paper, we introduce a class of adaptive importance sampling methods where the proposal distribution is constructed in a way that Gaussian processes are combined autoregressively. By numerical experiments of sampling from a high dimensional target distribution, we demonstrate that the method is accurate and efficient compared to existing methods.
更多查看译文
关键词
adaptive importance sampling,generative model,Gaussian Process,population Monte Carlo
AI 理解论文
溯源树
样例
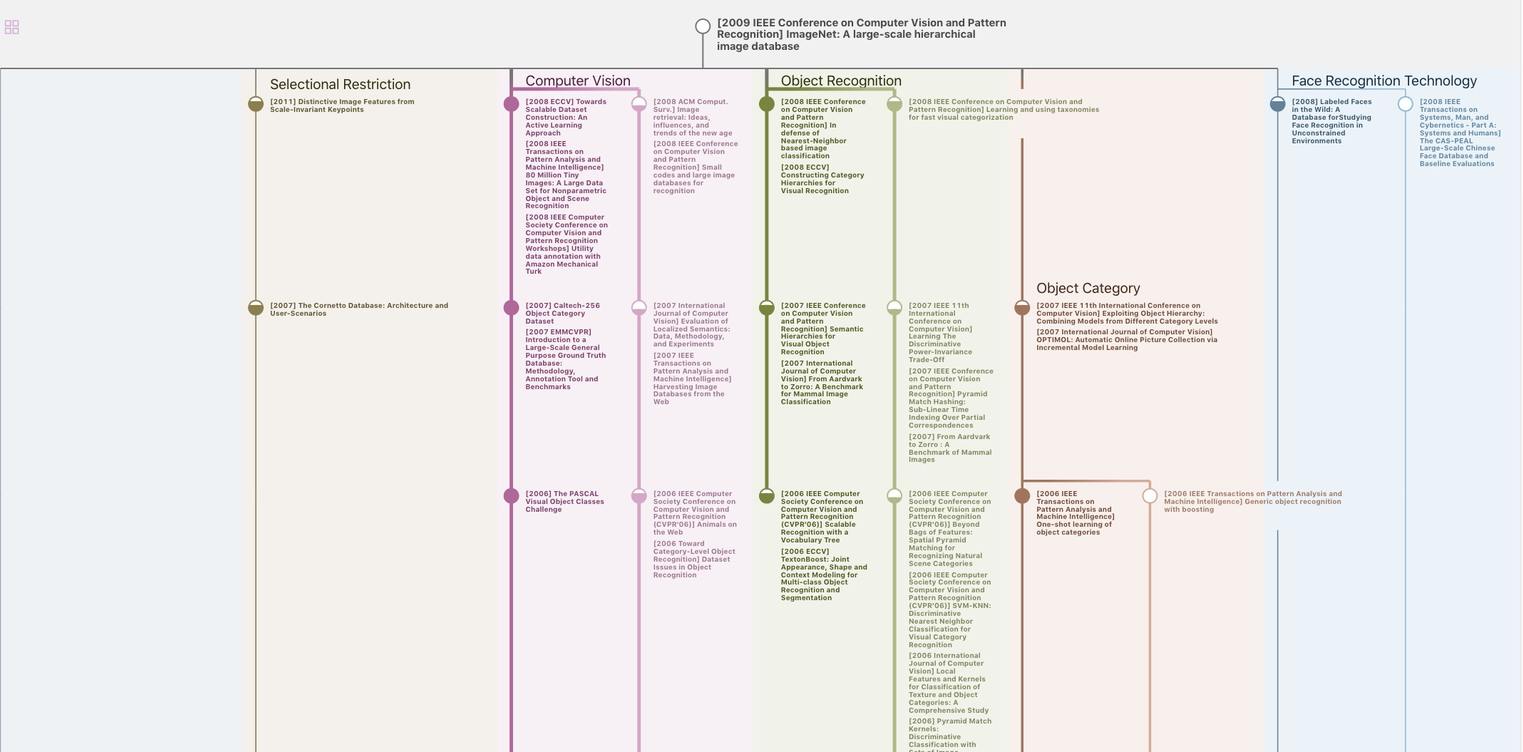
生成溯源树,研究论文发展脉络
Chat Paper
正在生成论文摘要