An Efficient Preprocessing-Based Approach to Mitigate Advanced Adversarial Attacks
IEEE TRANSACTIONS ON COMPUTERS(2024)
摘要
Deep Neural Networks are well-known to be vulnerable to Adversarial Examples. Recently, advanced gradient-based attacks were proposed (e.g., BPDA and EOT), which can significantly increase the difficulty and complexity of designing effective defenses. In this paper, we present a study towards the opportunity of mitigating those powerful attacks with only pre-processing operations. We make the following two contributions. First, we perform an in-depth analysis of those attacks and summarize three fundamental properties that a good defense solution should have. Second, we design a lightweight preprocessing function with these properties and the capability of preserving the model's usability and robustness against these threats. Extensive evaluations indicate that our solutions can effectively mitigate all existing standard and advanced attack techniques, and beat 11 state-of-the-art defense solutions published in top-tier conferences over the past 2 years.
更多查看译文
关键词
Perturbation methods,Training,Computational modeling,Robustness,Predictive models,Neural networks,Mathematical model,Adversarial examples,deep learning,adversarial attacks,BPDA
AI 理解论文
溯源树
样例
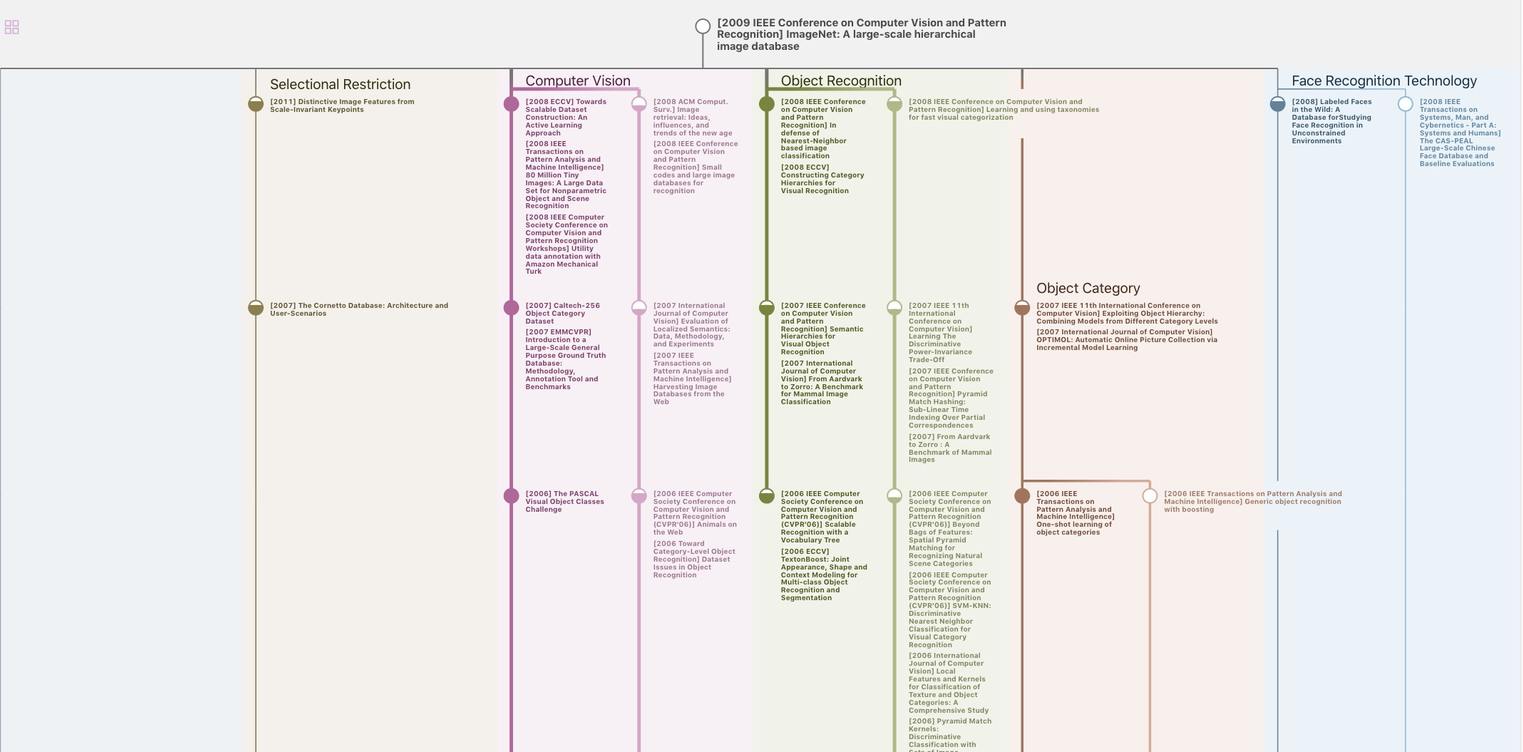
生成溯源树,研究论文发展脉络
Chat Paper
正在生成论文摘要