On the Convergence of Randomized Bregman Coordinate Descent for Non-Lipschitz Composite Problems
2021 IEEE INTERNATIONAL CONFERENCE ON ACOUSTICS, SPEECH AND SIGNAL PROCESSING (ICASSP 2021)(2021)
摘要
We propose a new randomized Bregman (block) coordinate descent (RBCD) method for minimizing a composite problem, where the objective function could be either convex or nonconvex, and the smooth part are freed from the global Lipschitz-continuous (partial) gradient assumption. Under the notion of relative smoothness based on the Bregman distance, we prove that every limit point of the generated sequence is a stationary point. Further, we show that the iteration complexity of the proposed method is $\mathcal{O}\left( {n{\varepsilon ^{ - 2}}} \right)$ to achieve ϵ-stationary point, where n is the number of blocks of coordinates. If the objective is assumed to be convex, the iteration complexity is improved to $\mathcal{O}\left( {n{ \in ^{ - 1}}} \right)$. If, in addition, the objective is strongly convex (relative to the reference function), the global linear convergence rate is recovered. We also present the accelerated version of the RBCD method, which attains an $\mathcal{O}\left( {n{\varepsilon ^{ - 1/\gamma }}} \right)$ iteration complexity for the convex case, where the scalar $\gamma \in [1,2]$ is determined by the generalized translation variant of the Bregman distance. Convergence analysis without assuming the global Lipschitz-continuous (partial) gradient sets our results apart from the existing works in the composite problems.
更多查看译文
关键词
Bregman distance, Non-Lipschitz, Coordinate Descent, Convex and Nonconvex Optimization
AI 理解论文
溯源树
样例
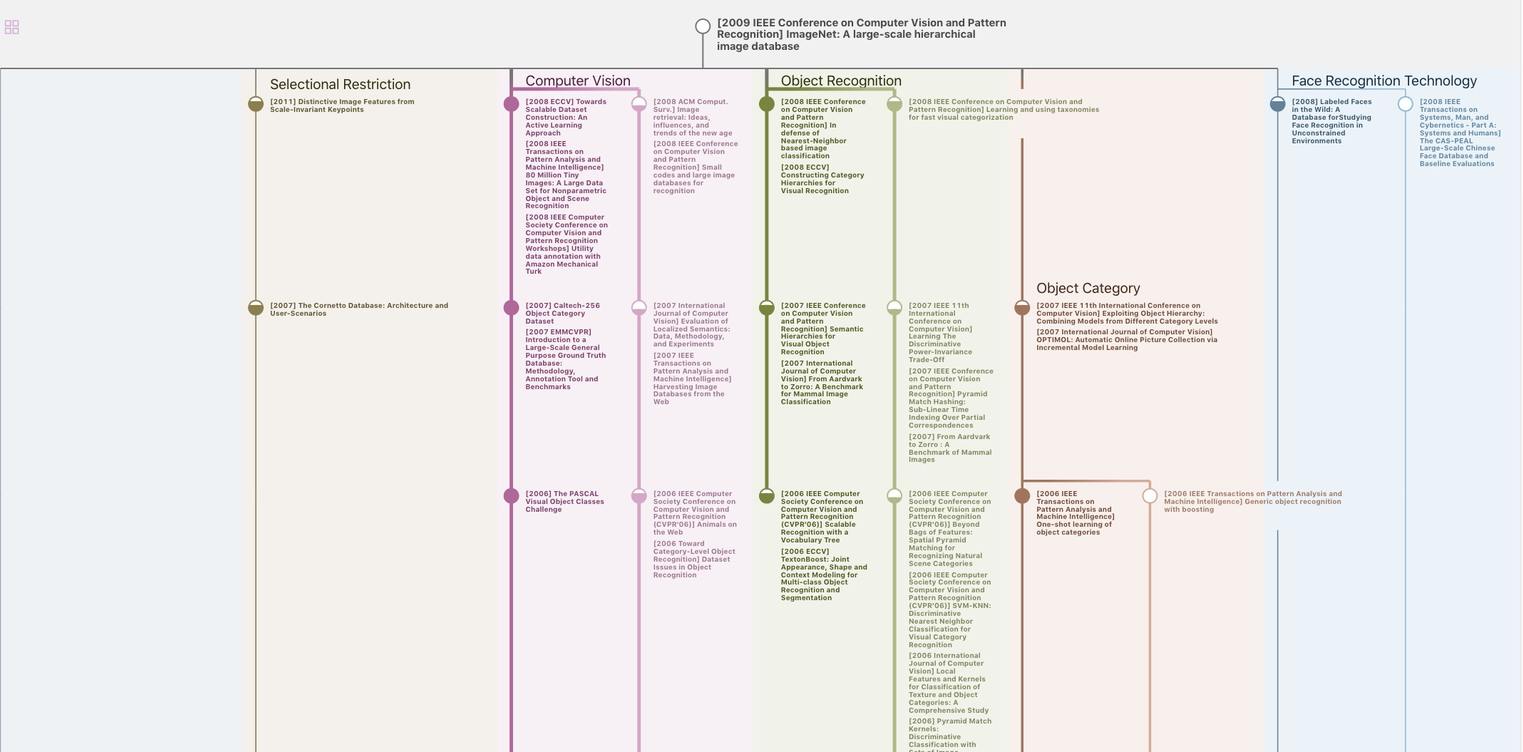
生成溯源树,研究论文发展脉络
Chat Paper
正在生成论文摘要