Impact of model parameterization on predictive uncertainty of regional groundwater models in the context of environmental impact assessment
Environmental Impact Assessment Review(2021)
摘要
Parameterization strategy impacts models' outputs and the associated uncertainty. This is particularly true for transient regional groundwater models where parameters can only be weakly constrained by insufficient observations. However, this is rarely investigated under any particular model structure. This study bridges this gap using a regional groundwater model developed to understand the impact of coal seam gas extraction on groundwater systems in a probabilistic framework. Two different parameterization schemes were implemented for hydraulic conductivity and specific storage. The first method solely relies on the relationship between hydraulic properties and burial depths. The second more complex strategy allows more spatial variations of hydraulic parameters using pilot points. The study provides new insights and practical guidance on the application of groundwater modelling for environmental impact assessment. The results suggest that the choice of model parameterization has a significant influence on predictive uncertainty. The model using the simple parameterization provides predictions with a much wider range than the model with a more sophisticated parameterization. This is because that the lowly parameterized model tends to generate more extreme effective hydraulic parameter fields unless the parameterization simplification converts the inverse problem to a (close to) well-posed problem that rarely exists for applied regional groundwater modelling. The potential impact of model parameterization should be discussed explicitly in groundwater modelling applications to support decision making to avoid misinterpretation of the modelling results.
更多查看译文
关键词
Groundwater modelling,Uncertainty analysis,Model complexity,Model simplification,Model parameterization
AI 理解论文
溯源树
样例
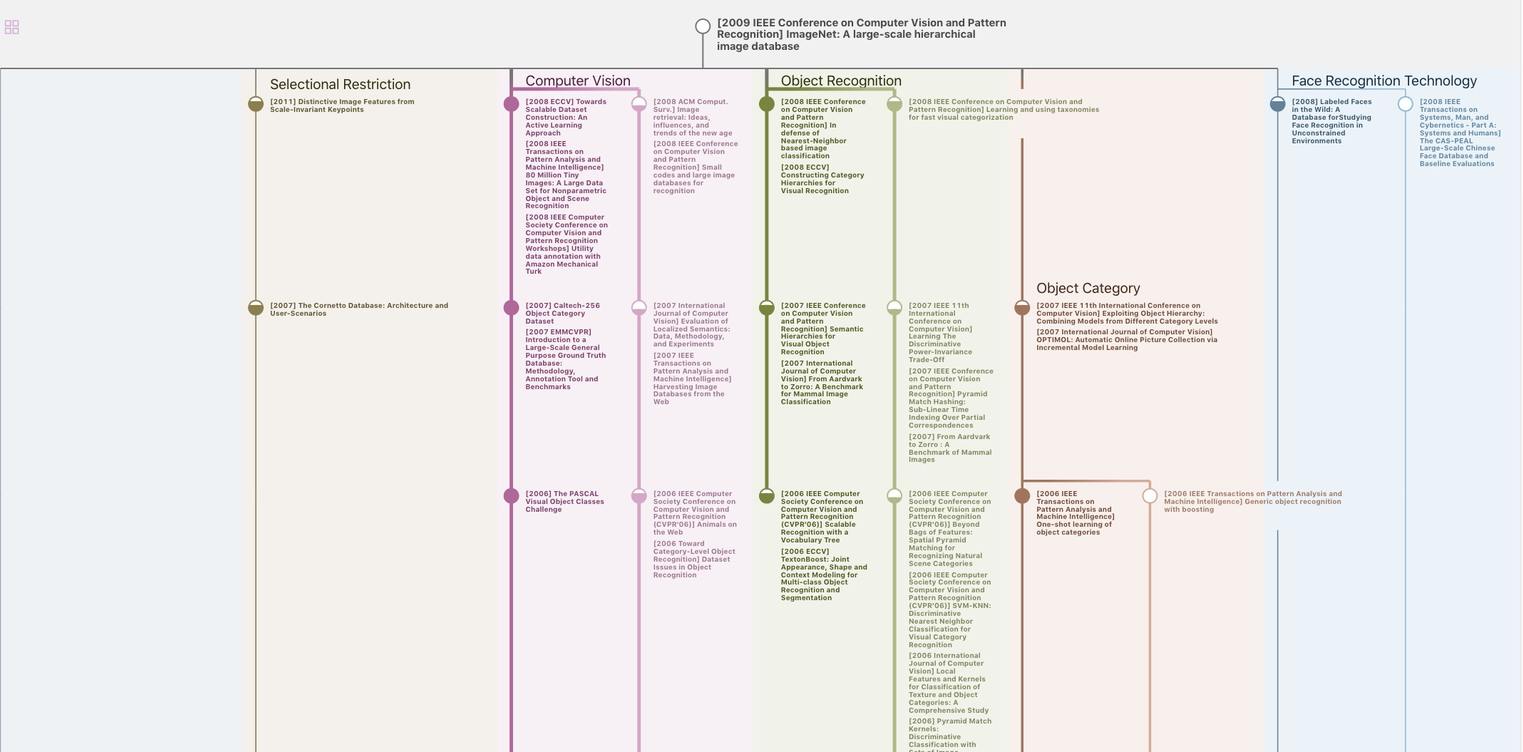
生成溯源树,研究论文发展脉络
Chat Paper
正在生成论文摘要