PhD Forum Abstract: Towards Reducing Labeling Efforts in IoT-based Machine Learning Systems
Cyber-physical Systems(2021)
摘要
The number of Internet-of-Things (IoT) and edge devices has exploded in recent years. Coupled with recent advances in learning methodologies, these can make the vision of smart building a reality and transform how people interact with their environment. Deep learning-driven systems have been already successful in many IoT application areas of pervasive sensing and ubiquitous computing including human activity monitoring and occupancy detection. However, the robustness of such systems at scale is dependent on the availability of a large amount of data labeled by a human expert. Acquiring human annotation involves high effort, high cost, and is often error-prone. Therefore, learning to execute tasks in a machine learning system without manual inspection is of prime interest as it can open up opportunities for continual learning in the long-term deployment of IoT systems. In this work, we propose deep neural network-based approaches for segmentation and classification tasks in sensor streams with no or limited labels. We focus on reducing human labeling efforts in machine learning systems by 1) leveraging temporal features learned through deep neural networks for downstream tasks and 2) learning robust features in dynamic environments by utilizing multiple sensing sources and semi-supervised learning (e.g. small amount of labeled data).
更多查看译文
关键词
Algorithms for sensing, Deep learning, Human-centered computing
AI 理解论文
溯源树
样例
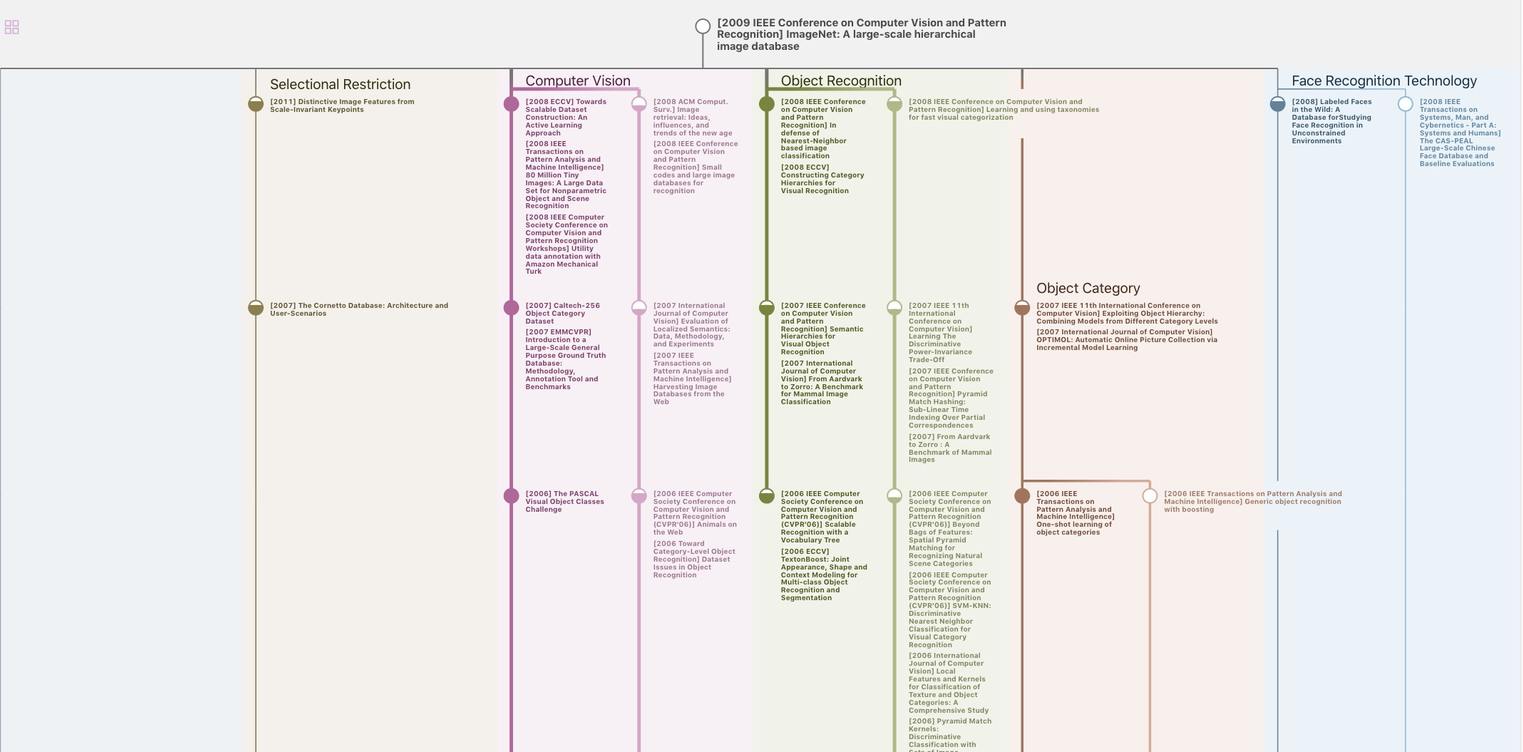
生成溯源树,研究论文发展脉络
Chat Paper
正在生成论文摘要