Discussion on Information Overlap in Hyperspectral Image Classification Based on Machine Learning.
International Conference on Image and Graphics Processing (ICIGP)(2021)
摘要
Principal component analysis(PCA) and spectral-spatial methods are usually used in hyperspectral images(HSI) classification based on machine learning. These methods have achieved high classification accuracy for several publicly hyperspectral datasets. However, we believe that high accuracy is mainly due to information overlap between the training and test set. In this paper, we have discussed the problem of information overlap caused by PCA and neighborhood region extraction. First, the PCA transformation matrix is fitted on the whole image, which leads to the spectral information overlap between the training and test samples. Second, there are pixels for testing in the neighborhood regions extracted by the training pixels, which cause spatial information overlap. In order to explore the influence of information overlap on classification results, we compare the classification results with or without information overlap using support vector machine (SVM) and convolutional neural network (CNN). Experiments indicate that classification results in above two information overlap cases are less reliable. In fact, in the method based on machine learning, the test set should be completely independent of the training set to ensure the accuracy, reliability and universality of the classification results. Therefore, the discussion on information overlap in HSIs classification method creates a new approach in future research and design for more reliable hyperspectral classification methods.
更多查看译文
AI 理解论文
溯源树
样例
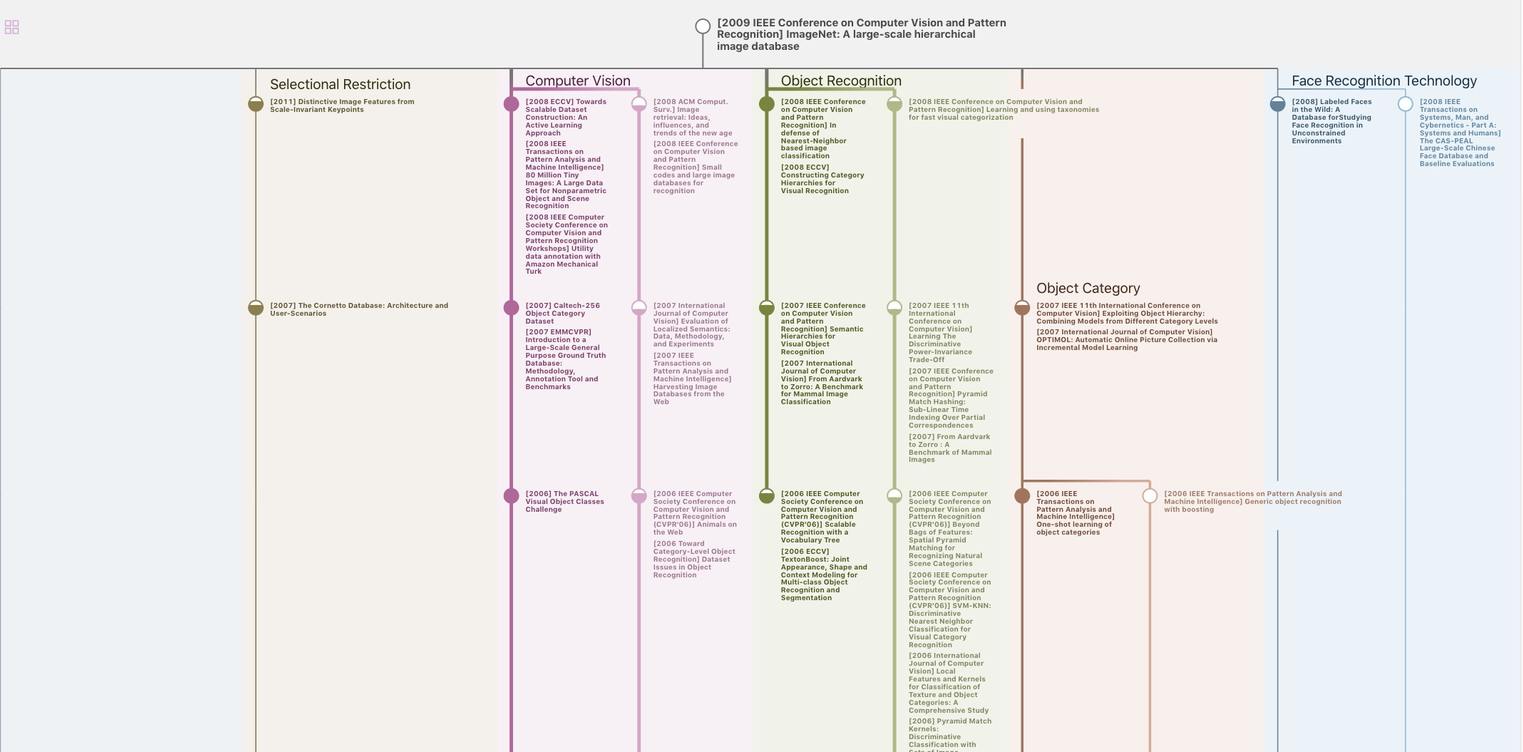
生成溯源树,研究论文发展脉络
Chat Paper
正在生成论文摘要