Discrepant collaborative training by Sinkhorn divergences
Image and Vision Computing(2021)
摘要
Deep Co-Training algorithms are typically comprised of two distinct and diverse feature extractors that simultaneously attempt to learn task-specific features from the same inputs. Achieving such an objective is, however, not trivial, despite its innocent look. This is because homogeneous networks tend to mimic each other under the collaborative training setup. Keeping this difficulty in mind, we make use of the newly proposed S∈ divergence to encourage diversity between homogeneous networks. The S∈ divergence encapsulates popular measures such as maximum mean discrepancy and the Wasserstein distance under the same umbrella and provides us with a principled, yet simple and straightforward mechanism. Our empirical results in two domains, classification in the presence of noisy labels and semi-supervised image classification, clearly demonstrate the benefits of the proposed framework in learning distinct and diverse features. We show that in these respective settings, we achieve impressive results by a notable margin.
更多查看译文
关键词
Co-training,Noisy labels,Weak-supervised learning
AI 理解论文
溯源树
样例
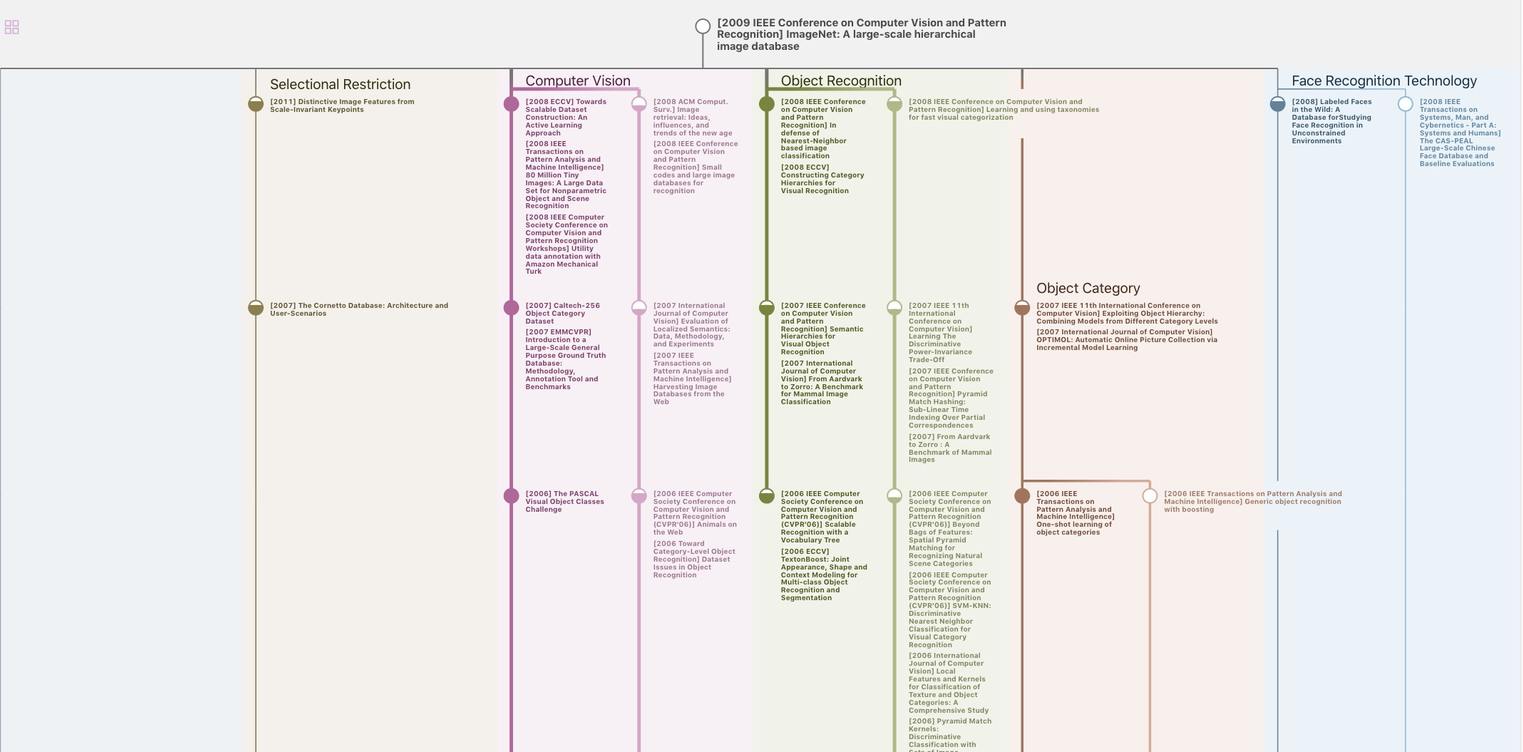
生成溯源树,研究论文发展脉络
Chat Paper
正在生成论文摘要