Principal Component Analysis of Dynamic [S-Methyl-11c]methionine PET Data Reveals Intratumor Heterogeneity in Gliomas
JOURNAL OF NUCLEAR MEDICINE(2021)
Abstract
1411 Objectives: Gliomas are the most common primary brain tumors whose diagnosis and follow-up often rely on MRI and amino acid (AA) PET. Recently, the added value of dynamic over static AA PET imaging has been demonstrated for biopsy targeting, classification, and recurrence diagnosis in gliomas. However, the analysis performed on such data is mostly qualitative or semi-quantitative and based on the mean time-activity curve (TAC) within a pre-delineated biological tumor volume (BTV). Nevertheless, gliomas are known to be highly heterogeneous tumors made of multiple genotypic populations. In this work, the ability of principal component analysis (PCA) to extract biologically relevant parametric maps from high-dimensional dynamic [S-methyl-11C]methionine ([11C]MET) PET data is investigated. The derived parametric map obtained are also compared to these provided by the classical one-tissue compartment pharmacokinetic model (1TCM). Methods: 33 glioma patients (20 males, median age 55 yr, 28 surgically treated, WHO grades II to IV, all histological types) were enrolled in this study. For each patient, a 30-minutes-and-30-seconds acquisition was started 30 seconds before the injection of 287 to 555 MBq of [11C]MET. 906 overlapping frames with duration 20 seconds and time spacing 2 seconds were reconstructed from the list file. The 33 reconstructed dynamic volumes were corrected for patient motion and inter-patient injection-to-carotid delay. In total, 6,420,534 voxel TACs were extracted from the segmented brain region of the 33 volumes, smoothed by averaging within 3x3x3 voxels neighborhood, and analyzed using PCA. To evaluate the robustness of our approach, the component definitions were determined on the brain TACs of 20 patients (3,974,466 TACs), then used to generate parametric maps from the brain TACs of the other 13 patients (2,446,068 TACs). Kinetic parameter maps were also generated from each patient’s smoothed TACs using the 1TCM and an image-derived function with PVE and population-based metabolite corrections. Results: Four of the first 6 principal component maps were found to be of clinical interest and are depicted in Figure 1e-h. The first component map (PC1, Figure 1e) is similar to the static PET image (Figure 1a) but has a higher tumor-to-background ratio and does not depend on an arbitrarily chosen injection-to-acquisition delay. PC2 (Figure 1f) reflects the vascular component of the TACs. PC3 (Figure 1g) distinguishes fast increasing then decreasing TACs from progressively increasing TACs within the BTV. Such behaviors were previously reported at the whole tumor level for high-grade and low-grade gliomas, respectively. PC3 would thus reflect intra-tumor heterogeneity of the proliferation potential possibly related to the local over-expression of the LAT1 trans-membrane amino-acid transporter. PC5 (Figure 1h) is related to the carotid-to-voxel delay and could reflect the effectiveness of the micro-vasculature. These maps also strongly to very strongly correlate with the 1TCM kinetic parameter maps depicted in Figure 1i-l. However, the different dynamic uptake behaviors are more clearly reflected by the PC3 map than the homologous 1/K2 map, as highlighted by TAC A, B, and C at voxels pointed by the red, blue, and green arrows in Figure 1. Conclusion: We proposed a novel methodology for the analysis of high-dimensional dynamic PET data based on PCA which does not require invasive arterial sampling. Principal components are determined from the whole brain TACs of multiple patients, which make them robust to inter-patient variations. We applied this methodology to dynamic [11C]MET PET data of glioma patients. One of the derived component maps highlighted intra-tumor heterogeneity in the uptake dynamics potentially linked to the local aggressiveness of the tumor. Such a parametric map could be of interest for biopsy targeting, surgery and radiotherapy planning, and recurrence diagnosis in gliomas.
MoreTranslated text
AI Read Science
Must-Reading Tree
Example
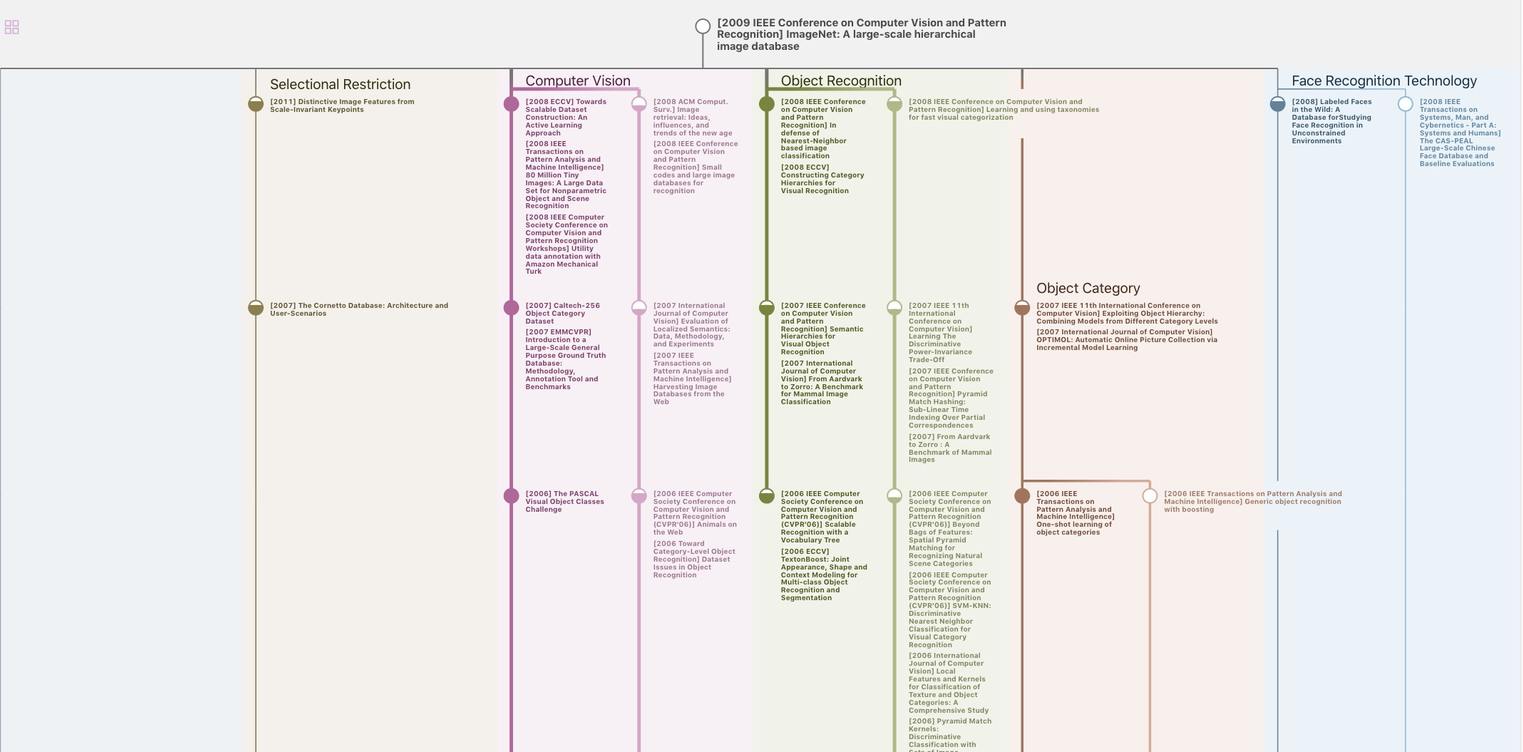
Generate MRT to find the research sequence of this paper
Chat Paper
Summary is being generated by the instructions you defined