Robot Navigation Based on Predicting of Human Interaction and Its Reproducible Evaluation in a Densely Crowded Environment
International journal of social robotics(2021)
摘要
To achieve robot navigation in crowded environments having high densities of moving people, it is insufficient to simply consider humans as moving obstacles and avoid collisions with them. That is, the impact of an approaching robot on human movements must be considered as well. Moreover, various navigation methods have been tested in their own environments in the literature, which made them difficult to compare with one another. Thus, we propose an autonomous robot navigation method in densely crowded environments for data-based predictions of robot-human interactions, together with a reproducible experimental test under controlled conditions. Based on localized positional relationships with humans, this method extracts multiple alternative paths, which can implement either following or avoidance, and selects an optimal path based on time efficiency. Each path is selected using neural networks, and the various paths are evaluated by predicting the position after a given amount of time has elapsed. These positions are then used to calculate the time required to reach a certain target position to ensure that the optimal path can be determined. We trained the predictor using simulated data and conducted experiments using an actual mobile robot in an environment where humans were walking around. Using our proposed method, collisions were avoided more effectively than when conventional navigation methods were used, and navigation was achieved with good time efficiency, resulting in an overall reduction in interference with humans. Thus, the proposed method enables an effective navigation in a densely crowded environment, while collecting human-interaction experience for further improvement of its performance in the future.
更多查看译文
关键词
Mobile robot navigation,Crowded environment,Human interaction,Planning
AI 理解论文
溯源树
样例
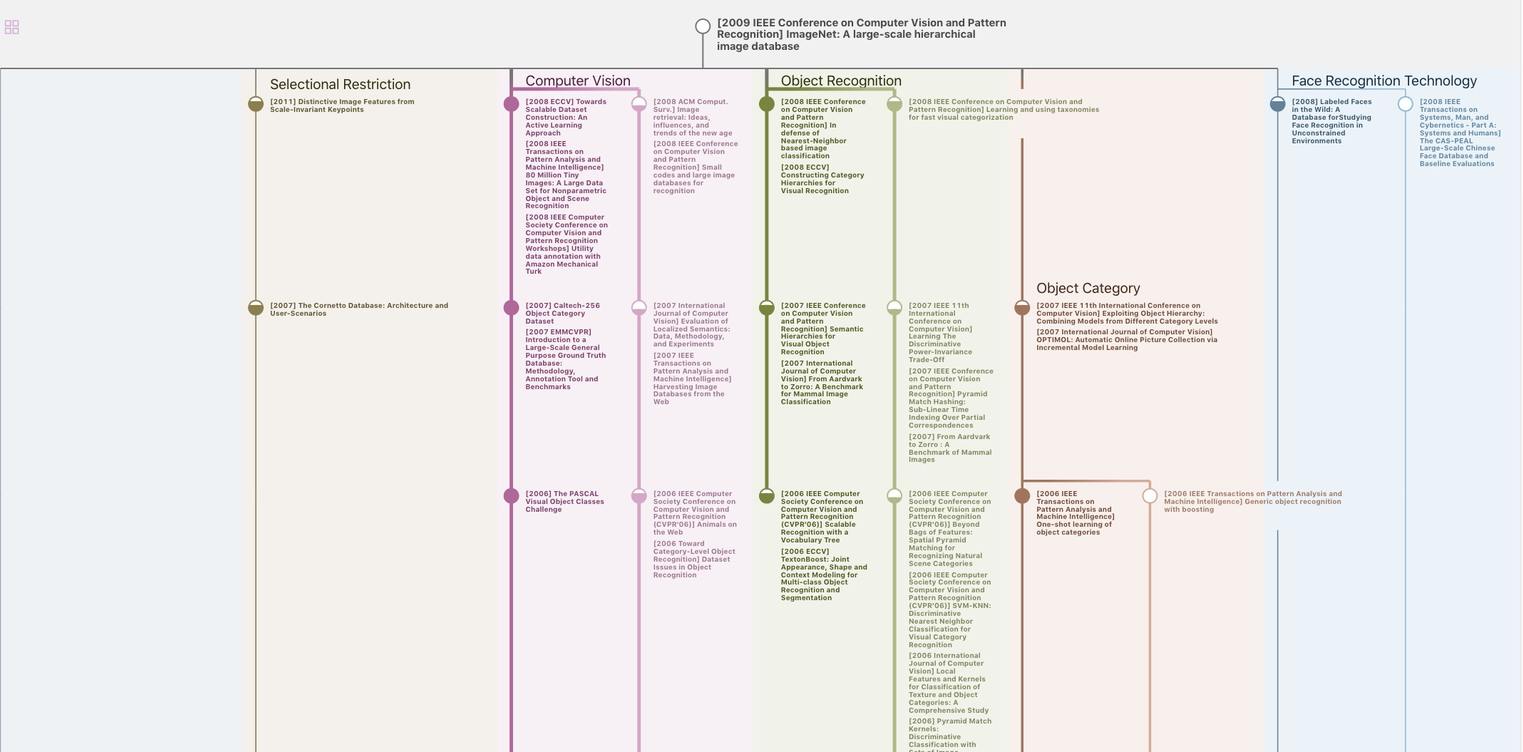
生成溯源树,研究论文发展脉络
Chat Paper
正在生成论文摘要