Computed Tomography Super-Resolution Using a Generative Adversarial Network in Bronchoscopy: A Clinical Feasibility Study
JOURNAL OF MEDICAL AND BIOLOGICAL ENGINEERING(2021)
摘要
Purpose To evaluate the usefulness of applying computed tomography (CT) images reconstructed by a deep learning super-resolution method to the clinical scenario of planning a real bronchoscopy procedure. Methods We trained a super-resolution generative adversarial network (SRGAN) to reconstruct CT images to high-resolution (SRGANrc). We tasked three pulmonologists with evaluating the quality of the CT images and the derived virtual bronchoscopies. We also compared the number of bronchi that were segmented by an automatic commercial program with the number of bronchi segmented in different processed thin-sectioned CT images. Results Regarding the human visual score, the original thin-sectioned CT images received more votes than the reconstructed CT images (SRGANrc) (29 votes versus eight votes). As for the human classification of four high-resolution CT images, the majority of images (83.7%) were classified correctly. Four out of 23 virtual bronchoscopies derived from super-resolution CT images were considered superior. The number of automatically segmented bronchi in super-resolution CT images was on average 1.5 less than that in the original thin-sliced CT images (mean bronchi: 15.1 vs. 16.6). Conclusion The reconstruction of super-resolution CT images through the SRGAN may have limited applications in the clinical scenarios of our study. In addition to improving the deep-learning algorithm, we need more clinical implementation tests to discover its value.
更多查看译文
关键词
Super-resolution, Bronchoscopy, Super-resolution generative adversarial network, Virtual bronchoscopy
AI 理解论文
溯源树
样例
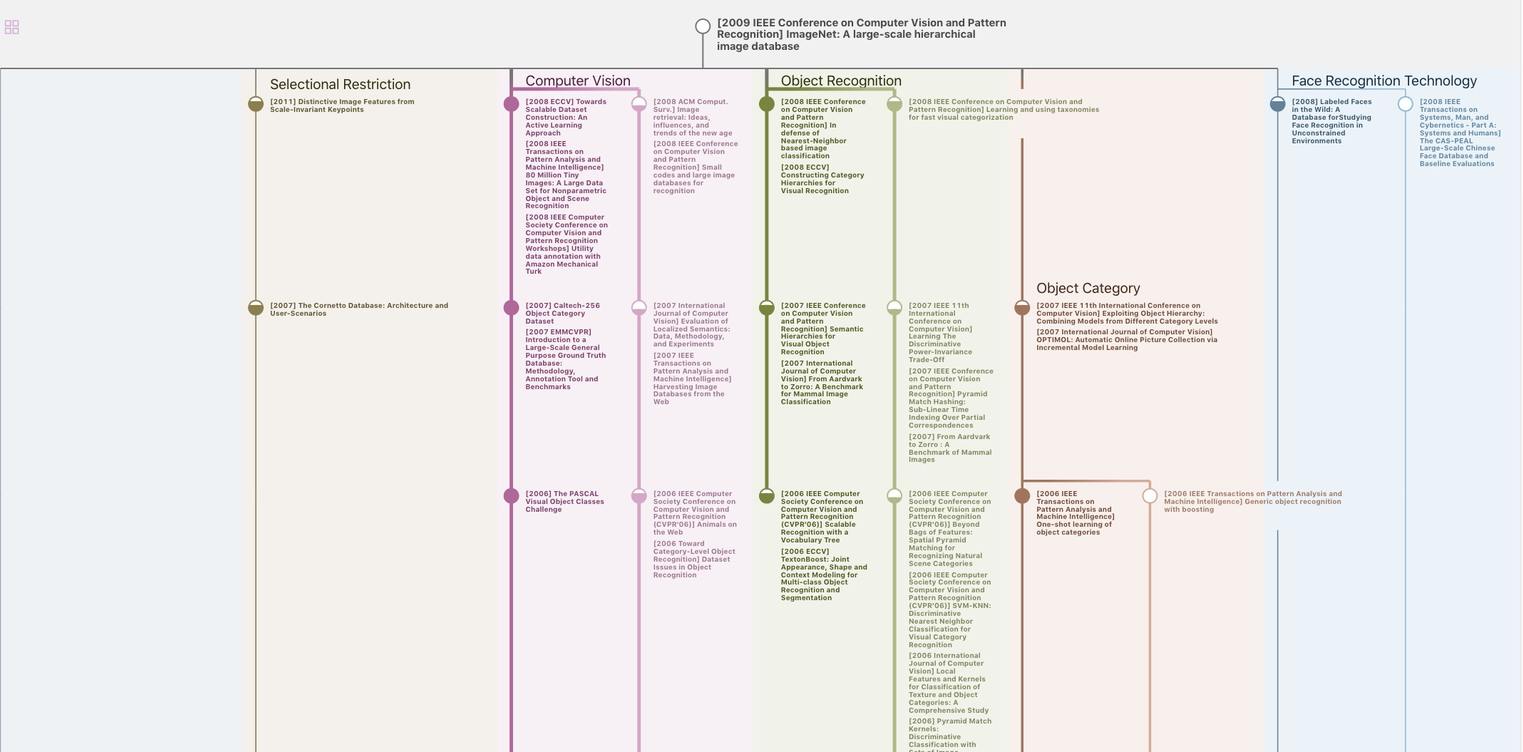
生成溯源树,研究论文发展脉络
Chat Paper
正在生成论文摘要