POMP++: Pomcp-based Active Visual Search in unknown indoor environments.
2021 IEEE/RSJ INTERNATIONAL CONFERENCE ON INTELLIGENT ROBOTS AND SYSTEMS (IROS)(2021)
摘要
In this paper, we focus on the problem of learning online an optimal policy for Active Visual Search (AVS) of objects in unknown indoor environments. We propose POMP++, a planning strategy that introduces a novel formulation on top of the classic Partially Observable Monte Carlo Planning (POMCP) framework, to allow training-free online policy learning in unknown environments. We present a new belief reinvigoration strategy that enables the use of POMCP with a dynamically growing state space to address the online generation of the floor map. We evaluate our method on two public benchmark datasets, AVD that is acquired by real robotic platforms and Habitat ObjectNav that is rendered from real 3D scene scans, achieving the best success rate with an improvement of >10% over the state-of-the-art methods.
更多查看译文
关键词
active visual search,pomcp-based
AI 理解论文
溯源树
样例
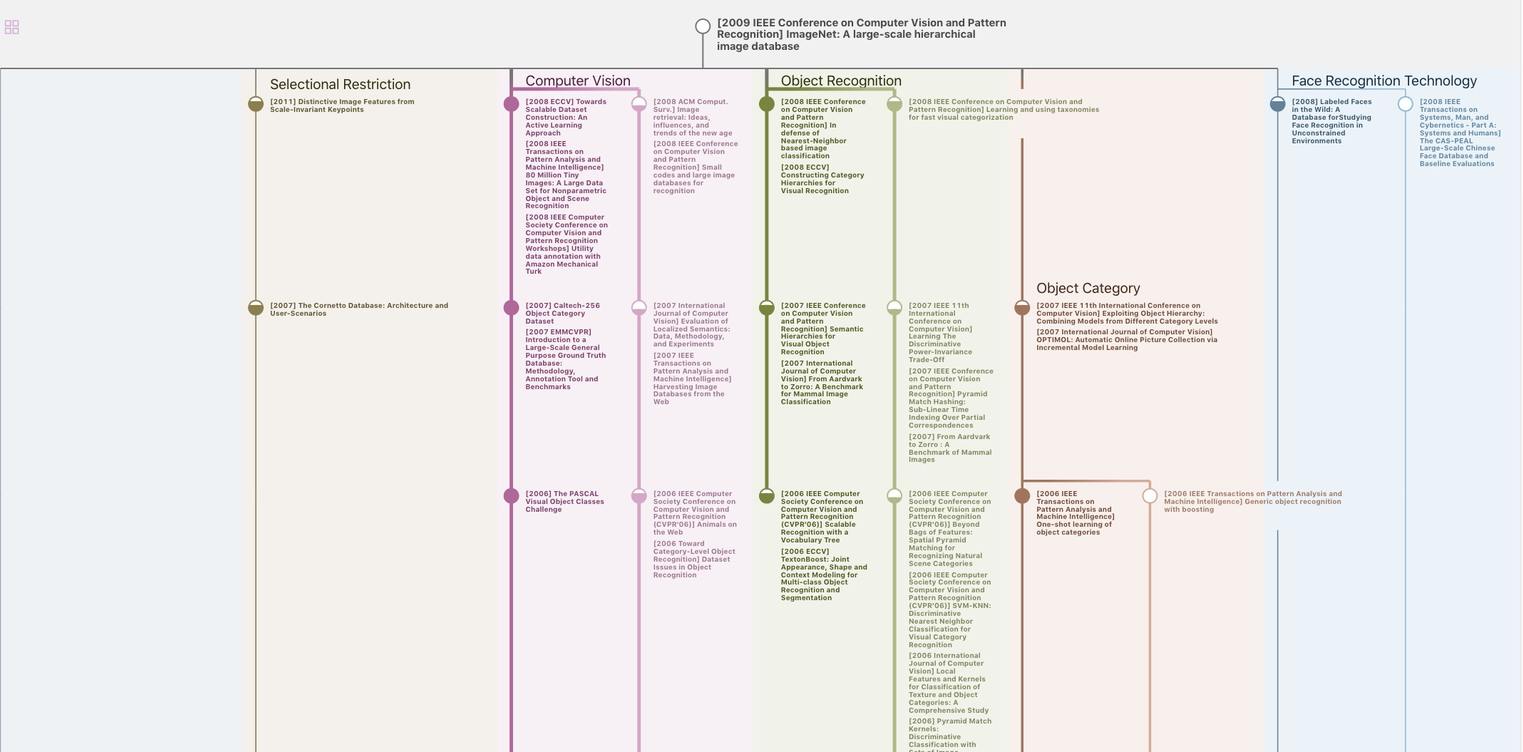
生成溯源树,研究论文发展脉络
Chat Paper
正在生成论文摘要