A Reinforcement Learning Approach For Placement Of Stateful Virtualized Network Functions
2021 IFIP/IEEE INTERNATIONAL SYMPOSIUM ON INTEGRATED NETWORK MANAGEMENT (IM 2021)(2021)
摘要
Network softwarization increases network flexibility by supporting the implementation of network functions such as firewalls as software modules. However, this creates new concerns on service reliability due to failures at both software and hardware level. The survivability of critical applications is commonly assured by deploying stand-by Virtual Network Functions (VNFs) to which the service is migrated upon failure of the primary VNFs. However, it is challenging to identify the optimal Data Centers (DCs) for hosting the active and stand-by VNF instances, not only to minimize their placement cost, but also the cost of a continuous state transfer between active and stand-by instances, since a number of VNFs are stateful. This paper proposes a reinforcement learning (RL) approach for the placement of stateful VNFs that considers a joint reservation of primary and backup resources with the objective of minimizing the overall placement cost. Simulation results show that the proposed algorithm is optimized in terms of both acceptance ratio and cost, resulting in up to 27% and 30% improvements in terms of accepted requests and placement cost compared to a state-of-the art algorithm.
更多查看译文
关键词
Resilient Embedding, Reinforcement Learning, Stateful VNF, Service Function Chainning
AI 理解论文
溯源树
样例
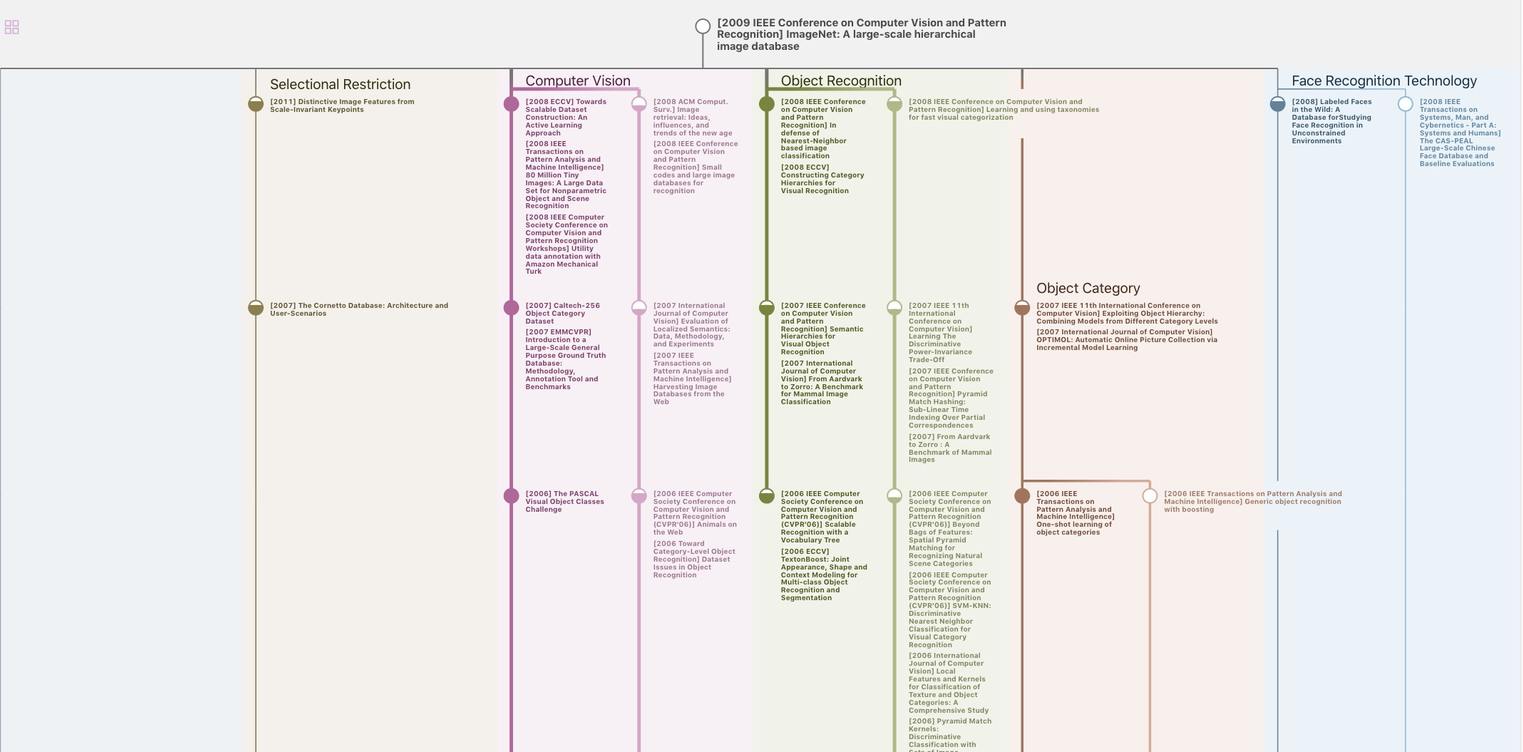
生成溯源树,研究论文发展脉络
Chat Paper
正在生成论文摘要