Multiplicative Weights Algorithms For Parallel Automated Software Repair
2021 IEEE 35TH INTERNATIONAL PARALLEL AND DISTRIBUTED PROCESSING SYMPOSIUM (IPDPS)(2021)
摘要
Multiplicative Weights Update (MWU) algorithms are a form of online learning that is applied to multi-armed bandit problems. Such problems involve allocating a fixed number of trials among multiple options to maximize cumulative payoff. MWU is a popular and effective method for dynamically balancing the trade-off between exploring the value of new options and exploiting the information already gained. However, no clear strategy exists to help practitioners choose which of the several algorithmic designs within this family to deploy. In this paper, three variants of parallel MWU algorithms are considered: Two parallel variants that rely on global memory, and one variant that uses distributed memory. The three variants are first analyzed theoretically, and then their effectiveness is assessed empirically on the task of estimating distributions in the context of stochastic search for repairs to bugs in software. Earlier work on APR suffers from various inefficiencies, and the paper shows how to decompose the problem into two stages: one that is embarrassingly parallel and one that is amenable to MWU. We then model the cast of each MWU variant and derive the conditions under which it is likely to be preferred in practice. We find that all three MWU algorithms achieve accuracy above 90% but that there are significant differences in runtime and total cost. When 90% accuracy is sufficient and evaluating options is expensive, such as in our use case, we find that the algorithm that uses global memory and has high communication cast outperforms the other two. We analyze the reasons for this surprising result.
更多查看译文
关键词
Automated Program Repair,Online Learning,Multiplicative Weights Update
AI 理解论文
溯源树
样例
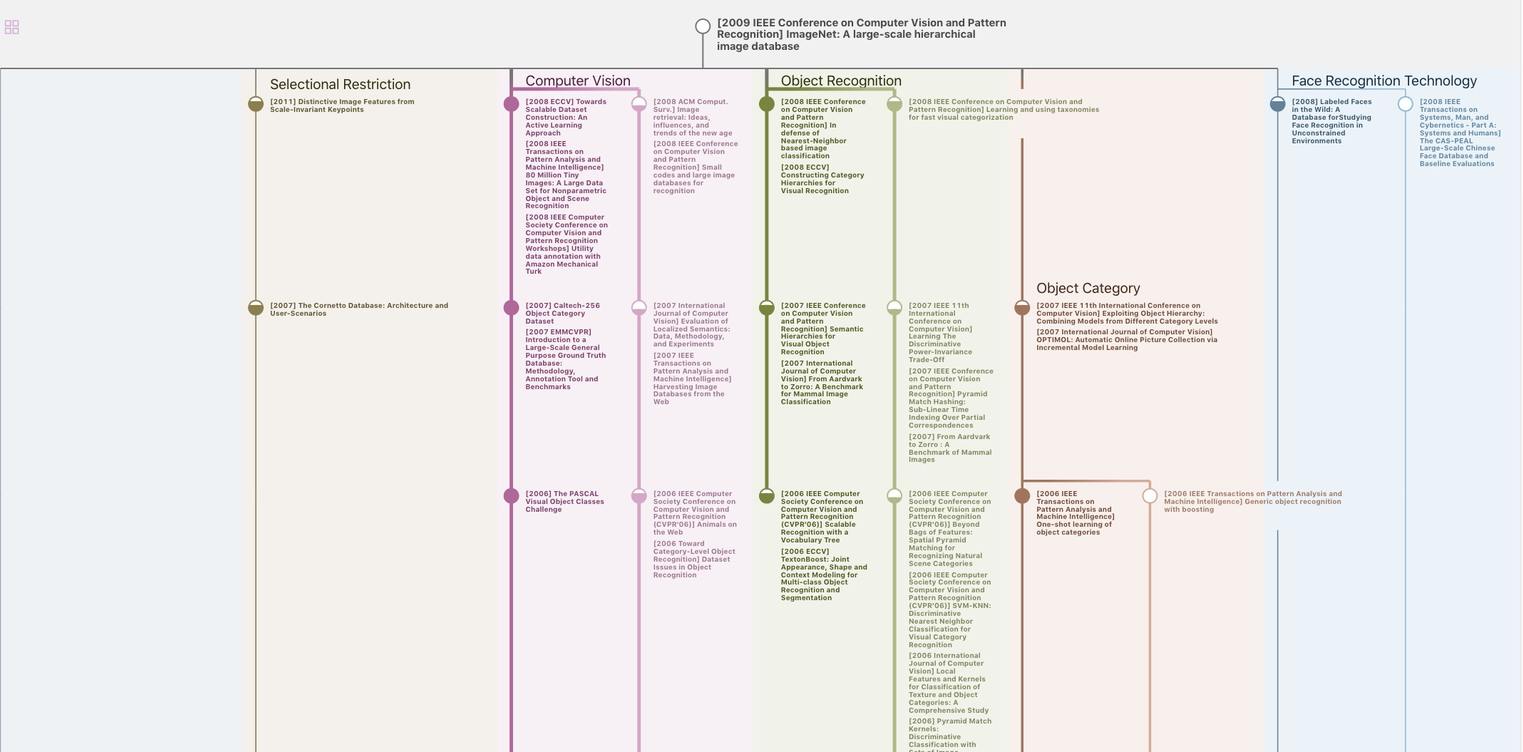
生成溯源树,研究论文发展脉络
Chat Paper
正在生成论文摘要