Machine Learning Model Predicts Short-Term Mortality among Prehospital Patients: A Prospective Development Study from Finland.
Resuscitation plus(2021)
摘要
AIM:To show whether adding blood glucose to the National Early Warning Score (NEWS) parameters in a machine learning model predicts 30-day mortality more precisely than the standard NEWS in a prehospital setting.METHODS:In this study, vital sign data prospectively collected from 3632 unselected prehospital patients in June 2015 were used to compare the standard NEWS to random forest models for predicting 30-day mortality. The NEWS parameters and blood glucose levels were used to develop the random forest models. Predictive performance on an unknown patient population was estimated with a ten-fold stratified cross-validation method.RESULTS:All NEWS parameters and blood glucose levels were reported in 2853 (79%) eligible patients. Within 30 days after contact with ambulance staff, 97 (3.4%) of the analysed patients had died. The area under the receiver operating characteristic curve for the 30-day mortality of the evaluated models was 0.682 (95% confidence interval [CI], 0.619-0.744) for the standard NEWS, 0.735 (95% CI, 0.679-0.787) for the random forest-trained NEWS parameters only and 0.758 (95% CI, 0.705-0.807) for the random forest-trained NEWS parameters and blood glucose. The models predicted secondary outcomes similarly, but adding blood glucose into the random forest model slightly improved its performance in predicting short-term mortality.CONCLUSIONS:Among unselected prehospital patients, a machine learning model including blood glucose and NEWS parameters had a fair performance in predicting 30-day mortality.
更多查看译文
关键词
Machine learning,Prehospital,Risk stratification,NEWS
AI 理解论文
溯源树
样例
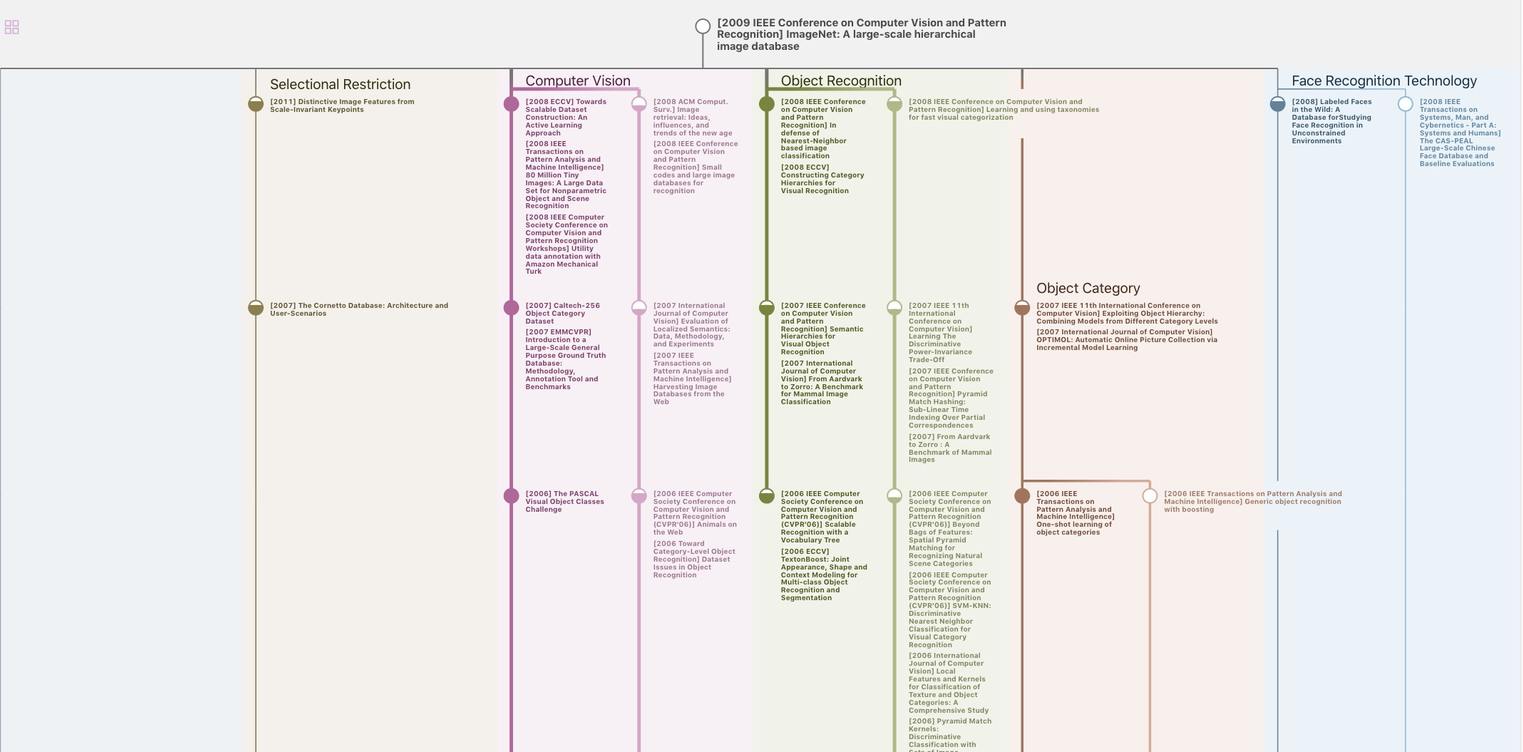
生成溯源树,研究论文发展脉络
Chat Paper
正在生成论文摘要