Extensions of ADMM for Separable Convex Optimization Problems with Linear Equality or Inequality Constraints
arxiv(2021)
摘要
The alternating direction method of multipliers (ADMM) proposed by Glowinski and Marrocco is a benchmark algorithm for two-block separable convex optimization problems with linear equality constraints. It has been modified, specified, and generalized from various perspectives to tackle more concrete or complicated application problems. Despite its versatility and phenomenal popularity, it remains unknown whether or not the ADMM can be extended to separable convex optimization problems with linear inequality constraints. In this paper, we lay down the foundation of how to extend the ADMM to two-block and multiple-block (more than two blocks) separable convex optimization problems with linear inequality constraints. From a high-level and methodological perspective, we propose a unified framework of algorithmic design and a roadmap for convergence analysis in the context of variational inequalities, based on which it is possible to design a series of concrete ADMM-based algorithms with provable convergence in the prediction-correction structure. The proposed algorithmic framework and roadmap for convergence analysis are eligible to both the two-block and multiple-block separable convex optimization problems, in which both linear equality and inequality constraints can be included. The analysis is comprehensive yet can be presented by elementary mathematics, and hence generically understandable.
更多查看译文
关键词
separable convex optimization problems,admm,inequality constraints
AI 理解论文
溯源树
样例
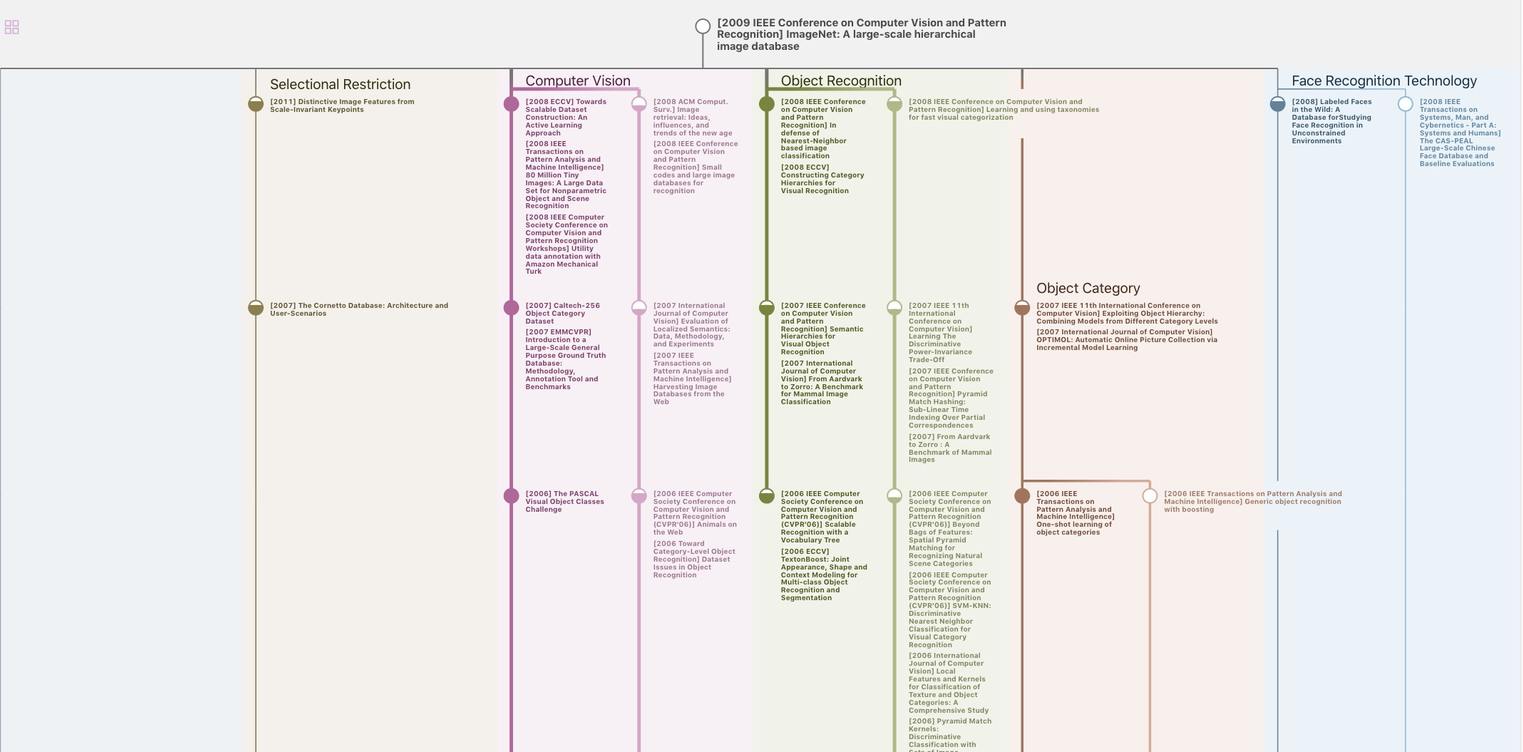
生成溯源树,研究论文发展脉络
Chat Paper
正在生成论文摘要