The Last-Iterate Convergence Rate of Optimistic Mirror Descent in Stochastic Variational Inequalities.
COLT(2021)
摘要
In this paper, we analyze the local convergence rate of optimistic mirror descent methods in stochastic variational inequalities, a class of optimization problems with important applications to learning theory and machine learning. Our analysis reveals an intricate relation between the algorithm's rate of convergence and the local geometry induced by the method's underlying Bregman function. We quantify this relation by means of the Legendre exponent, a notion that we introduce to measure the growth rate of the Bregman divergence relative to the ambient norm near a solution. We show that this exponent determines both the optimal step-size policy of the algorithm and the optimal rates attained, explaining in this way the differences observed for some popular Bregman functions (Euclidean projection, negative entropy, fractional power, etc.).
更多查看译文
关键词
optimistic mirror descent,stochastic variational inequalities,variational inequalities,last-iterate
AI 理解论文
溯源树
样例
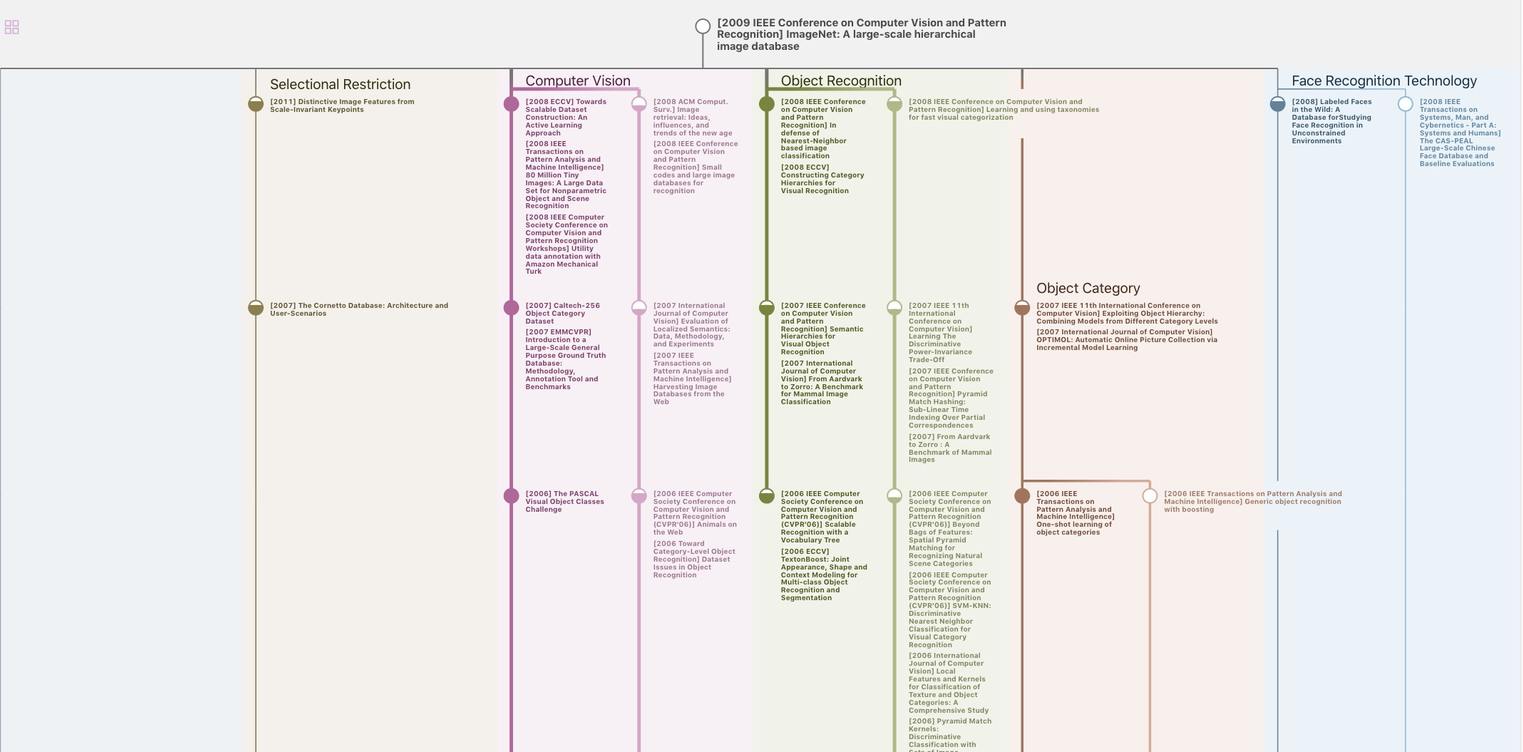
生成溯源树,研究论文发展脉络
Chat Paper
正在生成论文摘要