Label Noise in Segmentation Networks - Mitigation Must Deal with Bias.
DGM4MICCAI/DALI@MICCAI(2021)
摘要
Imperfect labels limit the quality of predictions learned by deep neural networks. This is particularly relevant in medical image segmentation, where reference annotations are difficult to collect and vary significantly even across expert annotators. Prior work on mitigating label noise focused on simple models of mostly uniform noise. In this work, we explore biased and unbiased errors artificially introduced to brain tumour annotations on MRI data. We found that supervised and semi-supervised segmentation methods are robust or fairly robust to unbiased errors but sensitive to biased errors. It is therefore important to identify the sorts of errors expected in medical image labels and especially mitigate the biased errors.
更多查看译文
关键词
Label noise,Segmentation,Neural networks
AI 理解论文
溯源树
样例
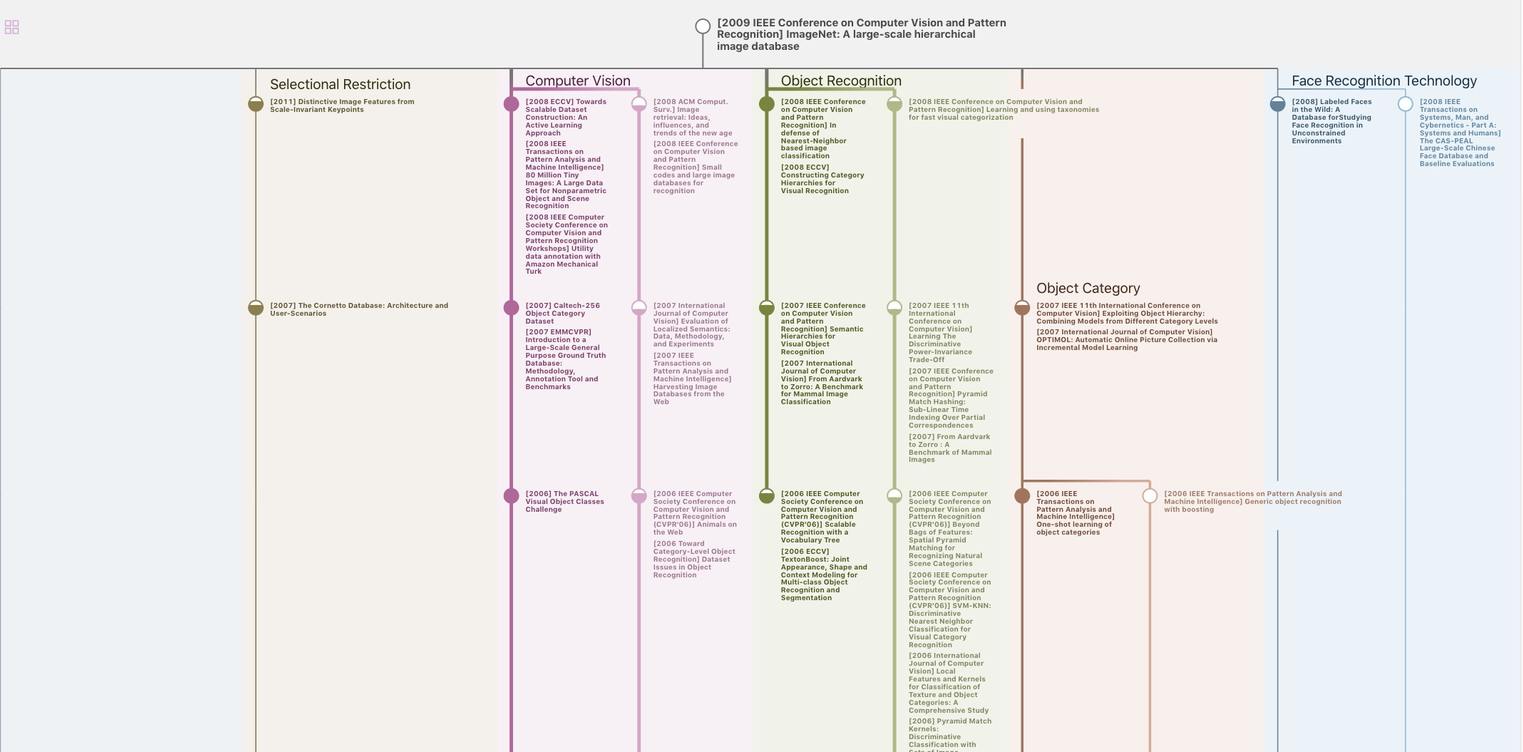
生成溯源树,研究论文发展脉络
Chat Paper
正在生成论文摘要