Towards Gaze-Based Prediction of the Intent to Interact in Virtual Reality
ACM Symposium on Eye Tracking Research and Applications(2021)
Facebook Reality Labs Facebook
Abstract
With the increasing frequency of eye tracking in consumer products, including head-mounted augmented and virtual reality displays, gaze-based models have the potential to predict user intent and unlock intuitive new interaction schemes. In the present work, we explored whether gaze dynamics can predict when a user intends to interact with the real or digital world, which could be used to develop predictive interfaces for low-effort input. Eye-tracking data were collected from 15 participants performing an item-selection task in virtual reality. Using logistic regression, we demonstrated successful prediction of the onset of item selection. The most prevalent predictive features in the model were gaze velocity, ambient/focal attention, and saccade dynamics, demonstrating that gaze features typically used to characterize visual attention can be applied to model interaction intent. In the future, these types of models can be used to infer user’s near-term interaction goals and drive ultra-low-friction predictive interfaces.
MoreTranslated text
PDF
View via Publisher
AI Read Science
AI Summary
AI Summary is the key point extracted automatically understanding the full text of the paper, including the background, methods, results, conclusions, icons and other key content, so that you can get the outline of the paper at a glance.
Example
Background
Key content
Introduction
Methods
Results
Related work
Fund
Key content
- Pretraining has recently greatly promoted the development of natural language processing (NLP)
- We show that M6 outperforms the baselines in multimodal downstream tasks, and the large M6 with 10 parameters can reach a better performance
- We propose a method called M6 that is able to process information of multiple modalities and perform both single-modal and cross-modal understanding and generation
- The model is scaled to large model with 10 billion parameters with sophisticated deployment, and the 10 -parameter M6-large is the largest pretrained model in Chinese
- Experimental results show that our proposed M6 outperforms the baseline in a number of downstream tasks concerning both single modality and multiple modalities We will continue the pretraining of extremely large models by increasing data to explore the limit of its performance
Try using models to generate summary,it takes about 60s
Must-Reading Tree
Example
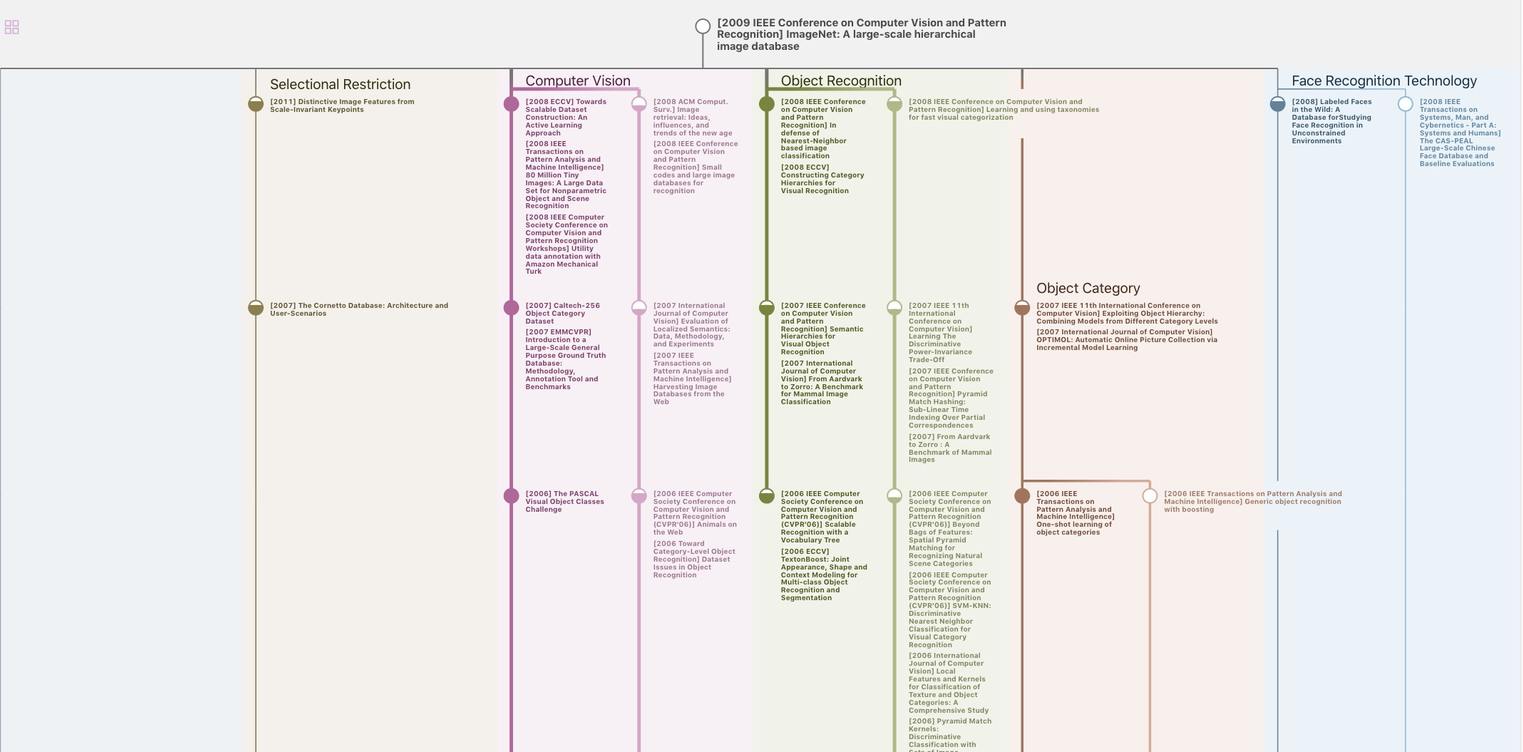
Generate MRT to find the research sequence of this paper
Related Papers
1997
被引用8736 | 浏览
2010
被引用1 | 浏览
2015
被引用39 | 浏览
2013
被引用46 | 浏览
2018
被引用22 | 浏览
2019
被引用5 | 浏览
2020
被引用62 | 浏览
2020
被引用81 | 浏览
2020
被引用28 | 浏览
Data Disclaimer
The page data are from open Internet sources, cooperative publishers and automatic analysis results through AI technology. We do not make any commitments and guarantees for the validity, accuracy, correctness, reliability, completeness and timeliness of the page data. If you have any questions, please contact us by email: report@aminer.cn
Chat Paper
去 AI 文献库 对话