An Exploration of Machine Learning Methods for Predicting Post-stroke Aphasia Recovery.
PETRA(2021)
摘要
Predicting the potential recovery outcome of post-stroke aphasia remains a challenging task. Our previous work[10] applied machine learning algorithms to predict participant response to therapy using a complex set of brain and behavioral data in individuals with post-stroke aphasia. The present work explores the additional predictive value of cognitive composite scores (CS), which measure visuo-spatial processing and verbal working memory; high-dimensional resting-state (RS) functional magnetic resonance imaging (fMRI) data, which measures the functional connectivity between brain regions; and diffusion tensor imaging (DTI) data, which provides information related to microstructural integrity via fractional anisotropy (FA) values. We first perform feature selection on the RS data as it has about 5 times more features than than all the other feature-sets combined. Next, we append these RS features, CS scores, and FA values to our existing data set. Finally, we train Support Vector Machine (SVM) and Random Forest (RF) classifiers for various combinations of feature-sets and compare their performance in terms of accuracy, F1-score, sensitivity and selectivity. Results show that combinations of feature-sets outperform most individual feature-sets and whereas each feature-set is present among the top 20 combinations, many of them contain RS.
更多查看译文
关键词
machine learning methods,recovery,post-stroke
AI 理解论文
溯源树
样例
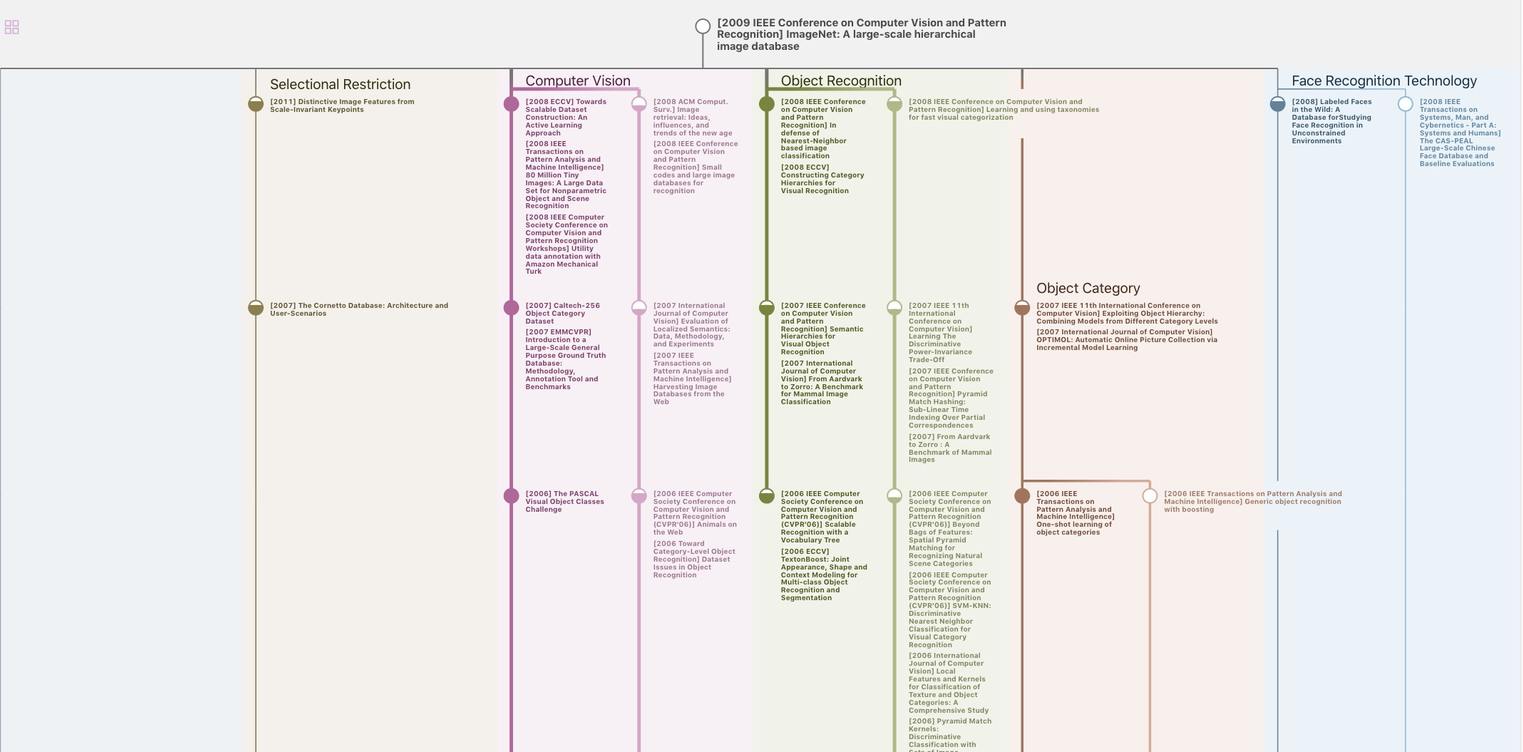
生成溯源树,研究论文发展脉络
Chat Paper
正在生成论文摘要