Runtime Replacement of Machine Learning Modules in FPGA-Based Systems.
MECO(2021)
摘要
Field Programmable Gate Arrays (FPGAs) are often used for accelerating machine learning algorithms. These algorithms can be implemented by various architectures which may differ in performance, required area, power consumption and reliability. This paper proposes the use of the Dynamic Partial Reconfiguration (DPR) capability of FPGAs to download the appropriate architecture depending on changing operating conditions (e.g., harsh environments, low battery). Since multipliers are usually an important component of machine learning circuits, three different multiplier implementations are studied in this paper along with two fault-tolerant architectures for each of them. Several scenarios are then described to illustrate the advantage of changing multiplier architecture on the fly, when the need arises. The concept presented in this research can be extended to other machine learning building blocks.
更多查看译文
关键词
FPGA,DPR,machine learning,multiplier,fault tolerance
AI 理解论文
溯源树
样例
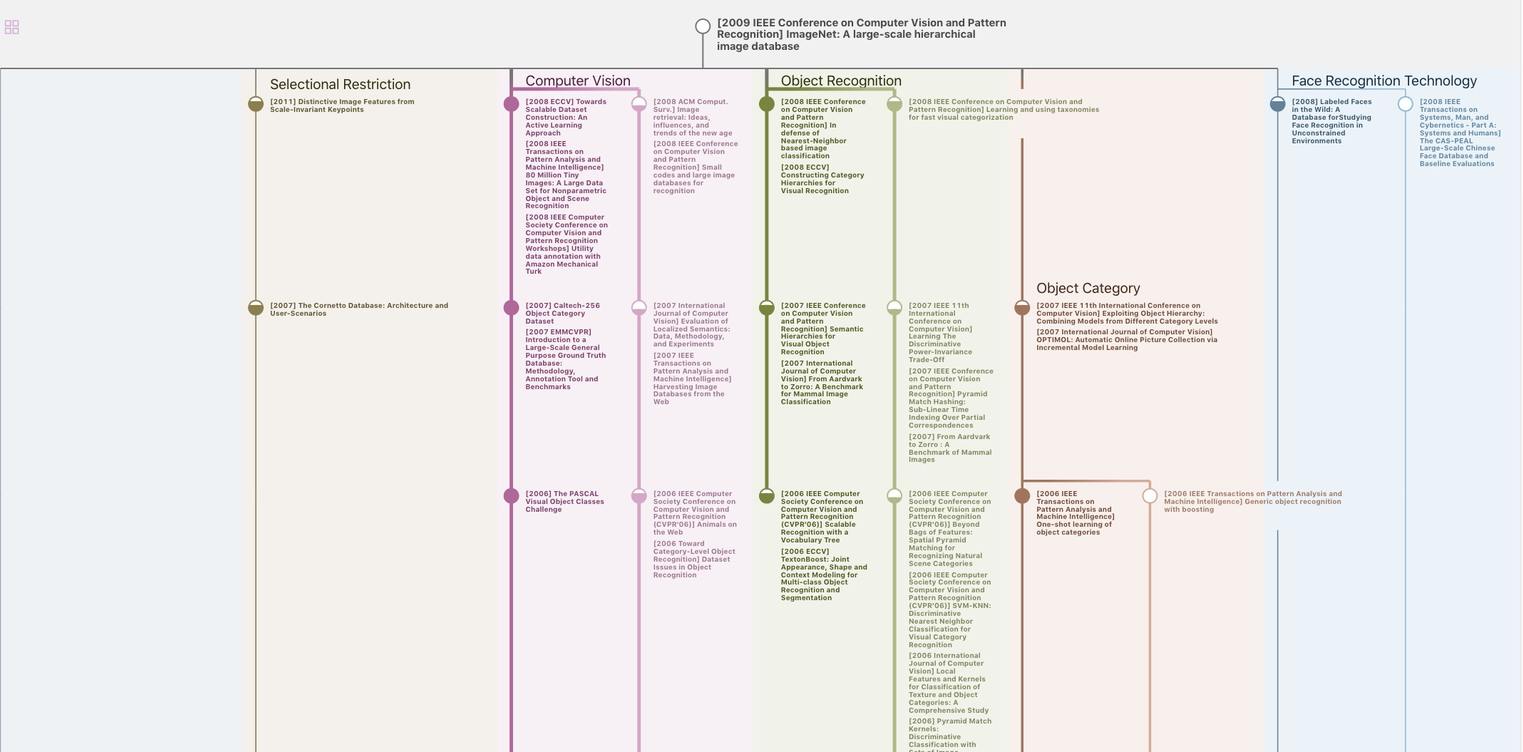
生成溯源树,研究论文发展脉络
Chat Paper
正在生成论文摘要