Perfect Sampling for (Atomic) Lovász Local Lemma
arxiv(2021)
摘要
We give a Markov chain based perfect sampler for uniform sampling solutions of constraint satisfaction problems (CSP). Under some mild Lovász local lemma conditions where each constraint of the CSP has a small number of forbidden local configurations, our algorithm is accurate and efficient: it outputs a perfect uniform random solution and its expected running time is quasilinear in the number of variables. Prior to our work, perfect samplers are only shown to exist for CSPs under much more restrictive conditions (Guo, Jerrum, and Liu, JACM’19). Our algorithm has two components: • A simple perfect sampling algorithm using bounding chains (Huber, STOC’98; Haggstrom and Nelander, Scandinavian Journal of Statistics’99). This sampler is efficient if each variable domain is small. • A simple but powerful state tensorization trick to reduce large domains to smaller ones. This trick is a generalization of state compression (Feng, He, and Yin, STOC’21). The crux of our analysis is a simple information percolation argument which allows us to achieve bounds even beyond current best approximate samplers (Jain, Pham, and Vuong, ArXiv’21). Previous related works either use intricate algorithms or need sophisticated analysis or even both. Thus we view the simplicity of both our algorithm and analysis as a strength of our work.
更多查看译文
AI 理解论文
溯源树
样例
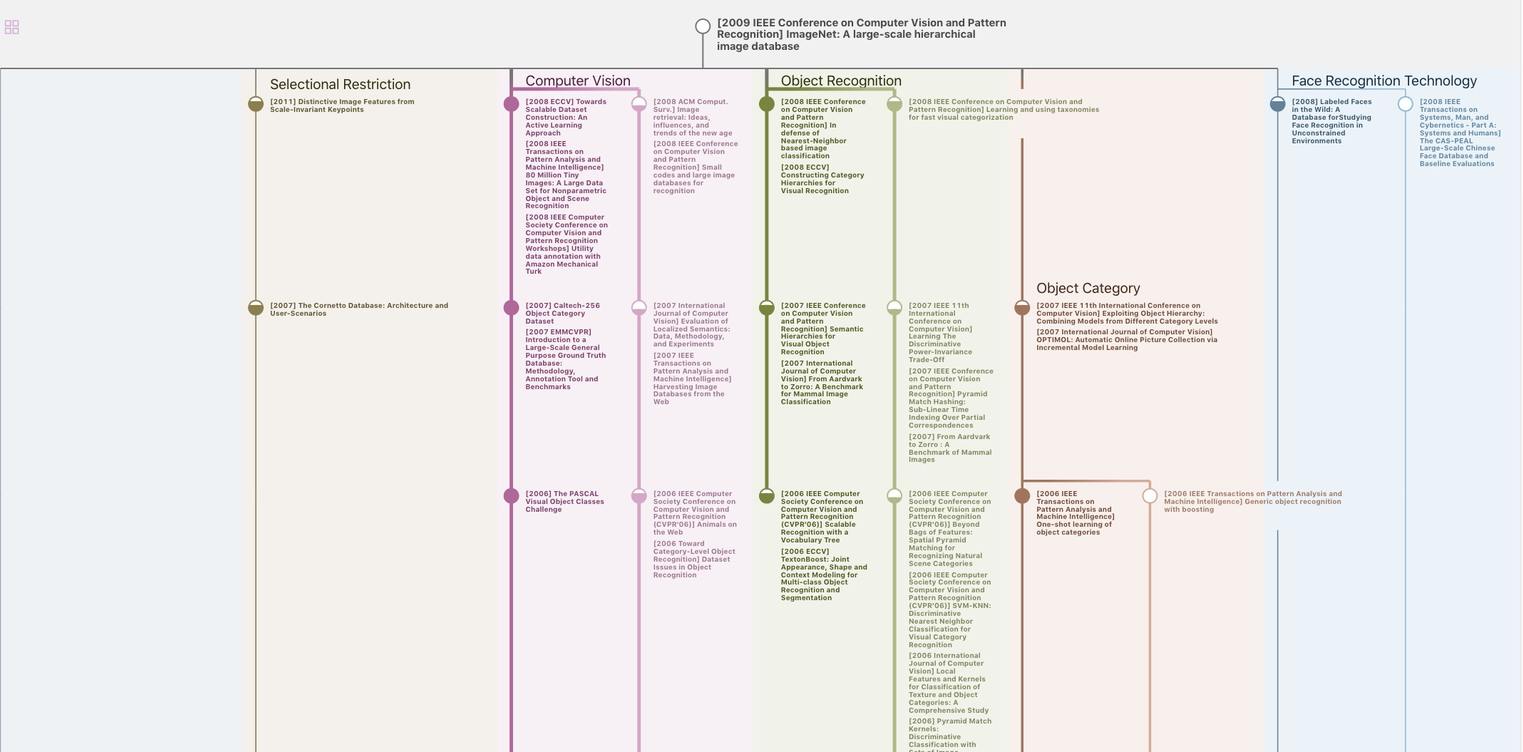
生成溯源树,研究论文发展脉络
Chat Paper
正在生成论文摘要