MSSET: A high-performance time-frequency analysis method for sparse-spectrum biomedical signal.
Computers in biology and medicine(2021)
摘要
When processing sparse-spectrum biomedical signals, traditional time-frequency (TF) analysis methods are faced with the defects of blurry energy concentration and low TF resolution caused by the Heisenberg uncertainty principle. The synchrosqueezing-based methods have demonstrated advanced TF performances in recent studies. However, these methods contain at least three drawbacks: (1) existence of non-reassigned points (NRPs), (2) low noise robustness, and (3) low amplitude accuracy. In this study, the novel TF method, termed multi-synchrosqueezing extracting transform (MSSET), is proposed to address these limitations. The proposed MSSET is divided into three steps. First, multisynchrosqueezing transform (MSST) is performed with specific iterations. Second, a synch-extracting is applied to retain the TF distribution of MSST results that relate most to time-varying information of the raw signal; meanwhile, the other smeared TF energy is discarded. Finally, the MSSET result is obtained by rounding the adjacent results at the frequency plane. Numerical verification results show that the proposed MSSET method can effectively solve the NRPs problem and enhance noise robustness. Furthermore, while retaining superior energy concentration and signal reconstruction capability, the MSSET's amplitude accuracy reaches about 90%, significantly higher than other methods. In the same conditions, the MSSET even consumes less time than MSST and IMSST. It also achieves the best composite performance with the least amplitude accuracy-time cost ratio (ATCR) index. Actual application examples in the Bat signal and the electrocardiograph (ECG) signal also validate the excellent performances of our method. To conclude, our proposed MSSET is superior to state-of-the-art methods and is expected to be widely used in the sparse-spectrum biomedical signal.
更多查看译文
AI 理解论文
溯源树
样例
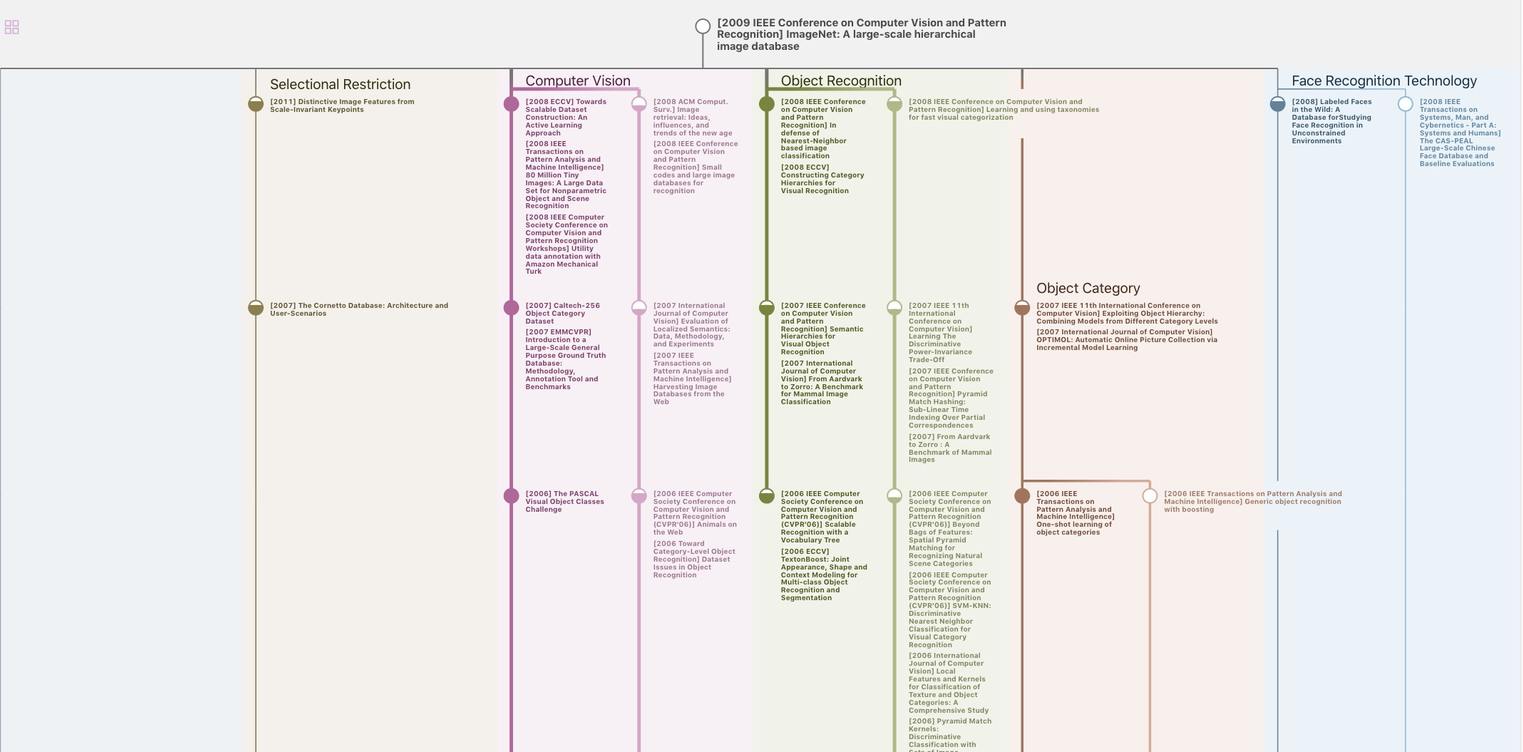
生成溯源树,研究论文发展脉络
Chat Paper
正在生成论文摘要