Transformer with peak suppression and knowledge guidance for fine-grained image recognition
Neurocomputing(2022)
摘要
Fine-grained image recognition is challenging because discriminative clues are usually fragmented, whether from a single image or multiple images. Despite their significant improvements, the majority of existing methods still focus on the most discriminative parts from a single image, ignoring informative details in other regions and lacking consideration of clues from other associated images. In this paper, we analyze the difficulties of fine-grained image recognition from a new perspective and propose a transformer architecture with the peak suppression module and knowledge guidance module, which respects the diversification of discriminative features in a single image and the aggregation of discriminative clues among multiple images. Specifically, the peak suppression module first utilizes a linear projection to convert the input image into sequential tokens. It then blocks the token based on the attention response generated by the transformer encoder. This module penalizes the attention to the most discriminative parts in the feature learning process, therefore, enhancing the information exploitation of the neglected regions. The knowledge guidance module compares the image-based representation generated from the peak suppression module with the learnable knowledge embedding set to obtain the knowledge response coefficients. Afterwards, it formalizes the knowledge learning as a classification problem using response coefficients as the classification scores. Knowledge embeddings and image-based representations are updated during training simultaneously so that the knowledge embedding includes a large number of discriminative clues for different images of the same category. Finally, we incorporate the acquired knowledge embeddings into the image-based representations as comprehensive representations, leading to significantly higher recognition performance. Extensive evaluations on the six popular datasets demonstrate the advantage of the proposed method in performance. The source code and models will be available online after the acceptance of the paper.
更多查看译文
关键词
Fine-grained image recognition,Food recognition,Knowledge guidance,Peak suppression,Vision transformer
AI 理解论文
溯源树
样例
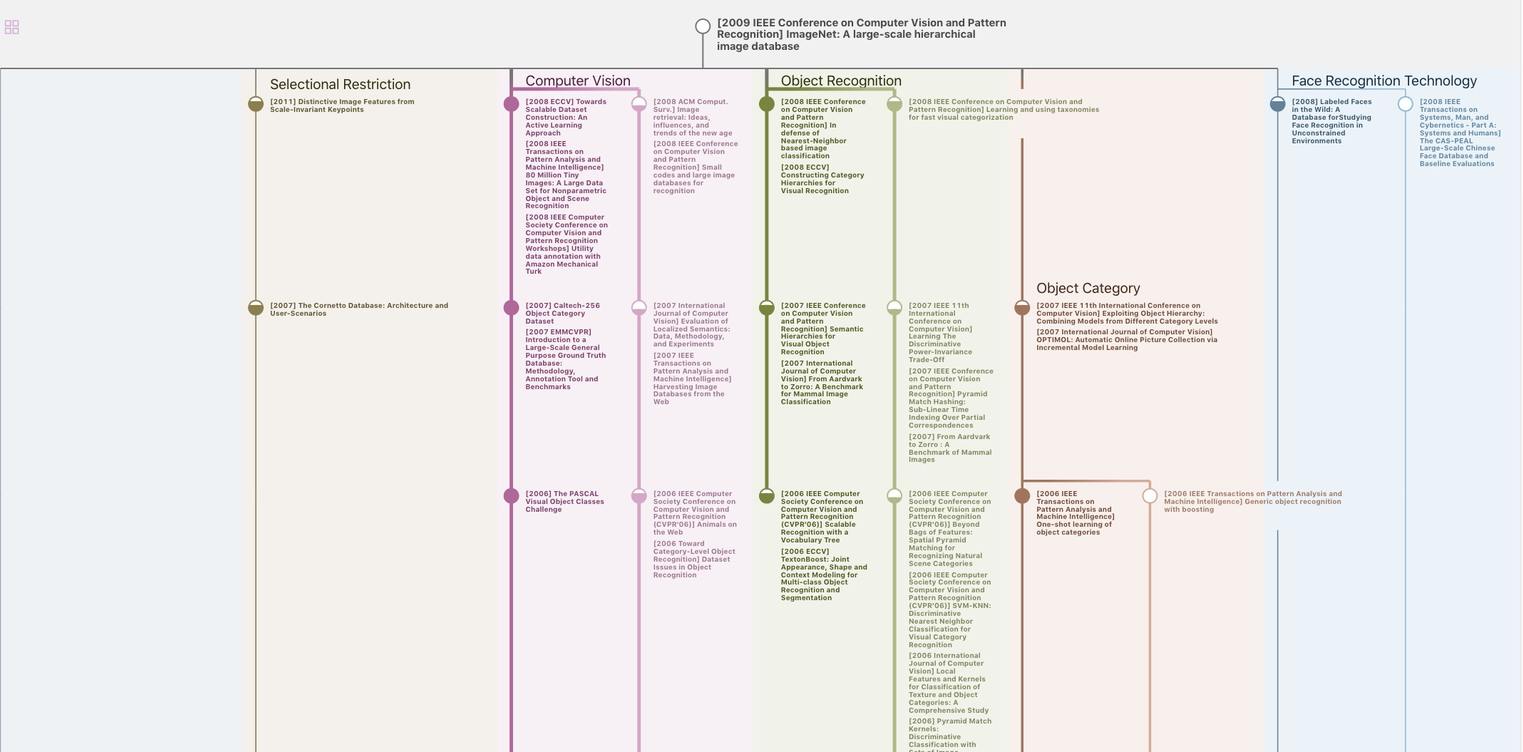
生成溯源树,研究论文发展脉络
Chat Paper
正在生成论文摘要