Reducing manual operation time to obtain a segmentation learning model for volume electron microscopy using stepwise deep learning with manual correction
MICROSCOPY(2021)
Abstract
Three-dimensional (3D) observation of a biological sample using serial-section electron microscopy is widely used. However, organelle segmentation requires a significant amount of manual time. Therefore, several studies have been conducted to improve organelle segmentation's efficiency. One such promising method is 3D deep learning (DL), which is highly accurate. However, the creation of training data for 3D DL still requires manual time and effort. In this study, we developed a highly efficient integrated image segmentation tool that includes stepwise DL with manual correction. The tool has four functions: efficient tracers for annotation, model training/inference for organelle segmentation using a lightweight convolutional neural network, efficient proofreading and model refinement. We applied this tool to increase the training data step by step (stepwise annotation method) to segment the mitochondria in the cells of the cerebral cortex. We found that the stepwise annotation method reduced the manual operation time by one-third compared with the fully manual method, where all the training data were created manually. Moreover, we demonstrated that the F1 score, the metric of segmentation accuracy, was 0.9 by training the 3D DL model with these training data. The stepwise annotation method using this tool and the 3D DL model improved the segmentation efficiency of various organelles.
MoreTranslated text
Key words
image segmentation,mouse cerebral cortex,deep convolutional neural network,electron microscopy image stack,machine learning
AI Read Science
Must-Reading Tree
Example
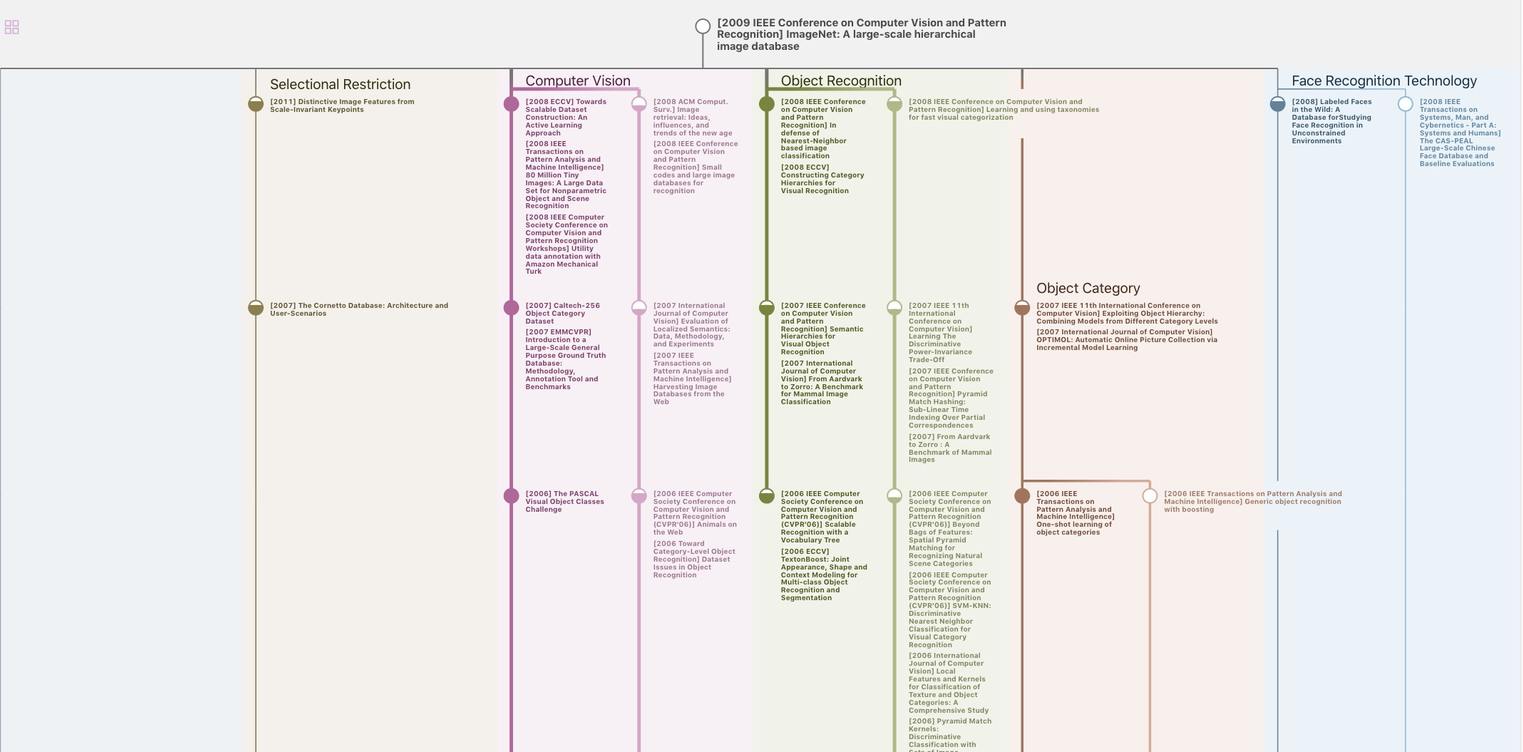
Generate MRT to find the research sequence of this paper
Chat Paper
Summary is being generated by the instructions you defined