Predicting Table Beet Root Yield With Multispectral Uas Imagery
REMOTE SENSING(2021)
摘要
Timely and accurate monitoring has the potential to streamline crop management, harvest planning, and processing in the growing table beet industry of New York state. We used unmanned aerial system (UAS) combined with a multispectral imager to monitor table beet (Beta vulgaris ssp. vulgaris) canopies in New York during the 2018 and 2019 growing seasons. We assessed the optimal pairing of a reflectance band or vegetation index with canopy area to predict table beet yield components of small sample plots using leave-one-out cross-validation. The most promising models were for table beet root count and mass using imagery taken during emergence and canopy closure, respectively. We created augmented plots, composed of random combinations of the study plots, to further exploit the importance of early canopy growth area. We achieved a R-2 = 0.70 and root mean squared error (RMSE) of 84 roots (similar to 24%) for root count, using 2018 emergence imagery. The same model resulted in a RMSE of 127 roots (similar to 35%) when tested on the unseen 2019 data. Harvested root mass was best modeled with canopy closing imagery, with a R-2 = 0.89 and RMSE = 6700 kg/ha using 2018 data. We applied the model to the 2019 full-field imagery and found an average yield of 41,000 kg/ha (similar to 40,000 kg/ha average for upstate New York). This study demonstrates the potential for table beet yield models using a combination of radiometric and canopy structure data obtained at early growth stages. Additional imagery of these early growth stages is vital to develop a robust and generalized model of table beet root yield that can handle imagery captured at slightly different growth stages between seasons.
更多查看译文
关键词
multispectral, precision agriculture, table beet, unmanned aerial system, yield prediction
AI 理解论文
溯源树
样例
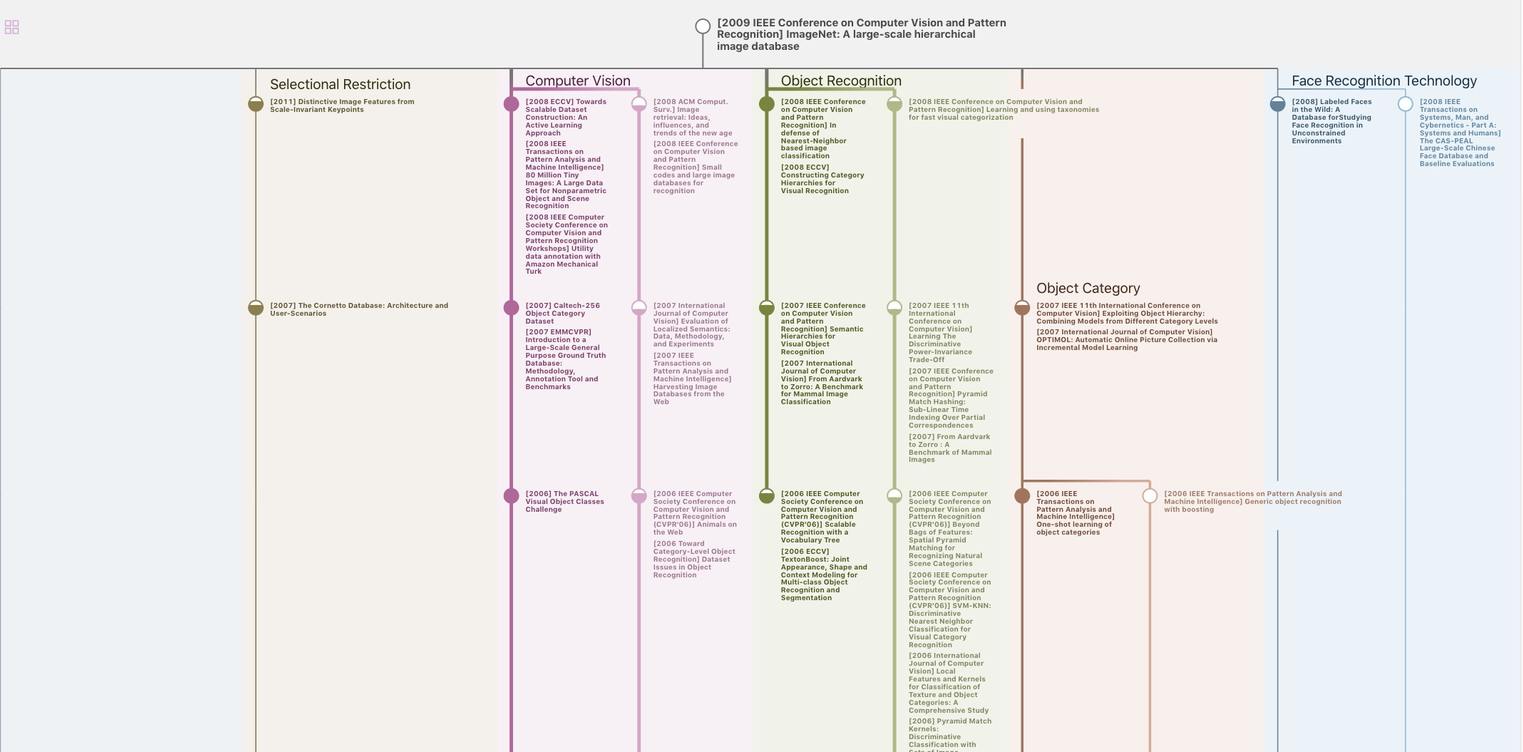
生成溯源树,研究论文发展脉络
Chat Paper
正在生成论文摘要