Learning Sparse Interaction Graphs of Partially Detected Pedestrians for Trajectory Prediction
IEEE Robotics and Automation Letters(2022)
摘要
Multi-pedestrian trajectory prediction is an indispensable element of autonomous systems that safely interact with crowds in unstructured environments. Many recent efforts in trajectory prediction algorithms have focused on understanding social norms behind pedestrian motions. Yet we observe these works usually hold two assumptions, which prevent them from being smoothly applied to robot applications: (1) positions of all pedestrians are consistently tracked, and (2) the target agent pays attention to all pedestrians in the scene. The first assumption leads to biased interaction modeling with incomplete pedestrian data. The second assumption introduces aggregation of redundant surrounding information, and the target agent may be affected by unimportant neighbors or present overly conservative motion. Thus, we propose Gumbel Social Transformer, in which an Edge Gumbel Selector samples a sparse interaction graph of partially detected pedestrians at each time step. A Node Transformer Encoder and a Masked LSTM encode pedestrian features with sampled sparse graphs to predict trajectories. We demonstrate that our model overcomes potential problems caused by the aforementioned assumptions, and our approach outperforms related works in trajectory prediction benchmarks. Code is available at
https://github.com/tedhuang96/gst
.
更多查看译文
关键词
Human-centered robotics,modeling and simulating humans
AI 理解论文
溯源树
样例
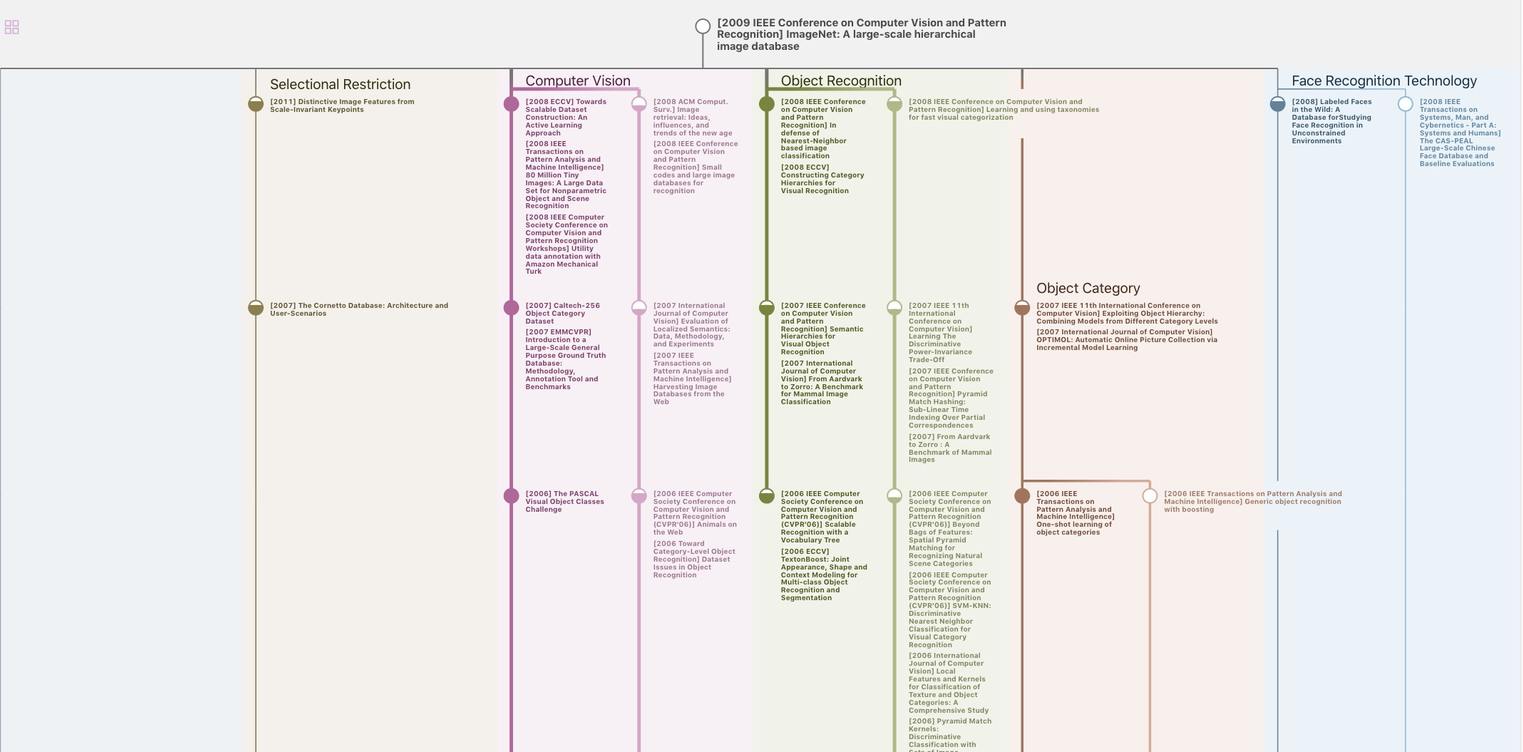
生成溯源树,研究论文发展脉络
Chat Paper
正在生成论文摘要