Spatial Heterogeneity Modeling Of Water Quality Based On Random Forest Regression And Model Interpretation
ENVIRONMENTAL RESEARCH(2021)
摘要
A systematic understanding of the spatial distribution of water quality is critical for successful watershed management; however, the limited number of physical monitoring stations has restricted the evaluation of spatial water quality distribution and the identification of features impacting the water quality. To fill this gap, we developed a modeling process that employed the random forest regression (RFR) to model the water quality distribution for the Taihu Lake basin in Zhejiang Province, China, and adopted the Shapley Additive exPlanations (SHAP) method to interpret the underlying driving forces. We first used RFR to model three water quality parameters: permanganate index (CODMn), total phosphorus (TP), and total nitrogen (TN), based on 16 watershed features. We then applied the built models to generate water quality distribution maps for the basin, with the CODMn ranging from 1.39 to 6.40 mg/L, TP from 0.02 to 0.23 mg/L, and TN from 1.43 to 4.27 mg/L. These maps showed generally consistent patterns among the CODMn, TN, and TP with minor differences in the spatial distribution. The SHAP analysis showed that the TN was mainly affected by agricultural non-point sources, while the CODMn and TP were affected by agricultural and domestic sources. Due to differences in sewage collection and treatment between urban and rural areas, the water quality in highly populated urban areas was better than that in rural areas, which led to an unexpected positive relationship between water quality and population density. Overall, with the RFR models and SHAP interpretation, we obtained a continuous distribution pattern of the water quality and identified its driving forces in the basin. These findings provided important information to assist water quality restoration projects.
更多查看译文
关键词
Water quality assessment, Machine learning, Random forest regression, Driving force analysis, Shapley additive explanations
AI 理解论文
溯源树
样例
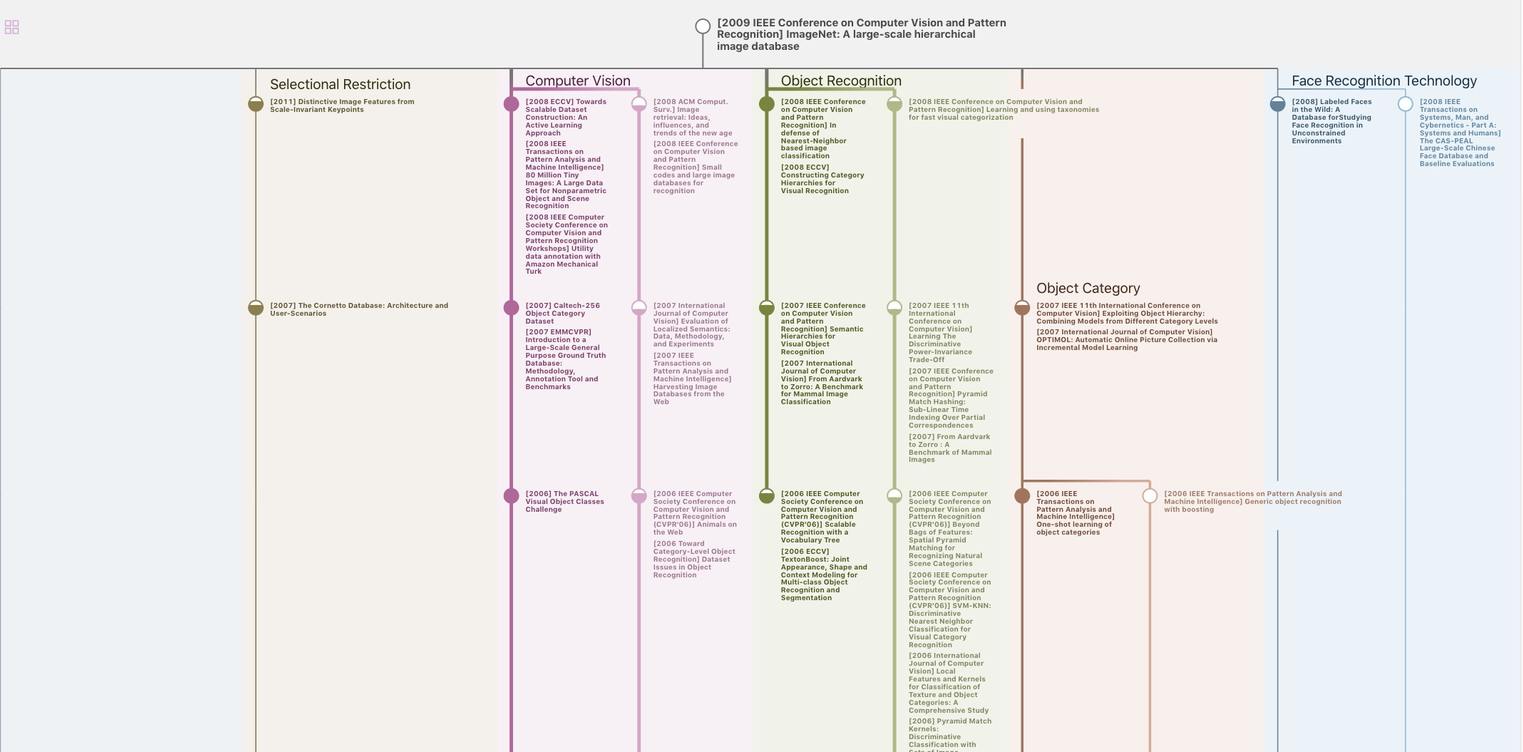
生成溯源树,研究论文发展脉络
Chat Paper
正在生成论文摘要