A meta-inference framework to integrate multiple external models into a current study.
Biostatistics (Oxford, England)(2023)
摘要
It is becoming increasingly common for researchers to consider incorporating external information from large studies to improve the accuracy of statistical inference instead of relying on a modestly sized data set collected internally. With some new predictors only available internally, we aim to build improved regression models based on individual-level data from an "internal" study while incorporating summary-level information from "external" models. We propose a meta-analysis framework along with two weighted estimators as the composite of empirical Bayes estimators, which combines the estimates from different external models. The proposed framework is flexible and robust in the ways that (i) it is capable of incorporating external models that use a slightly different set of covariates; (ii) it is able to identify the most relevant external information and diminish the influence of information that is less compatible with the internal data; and (iii) it nicely balances the bias-variance trade-off while preserving the most efficiency gain. The proposed estimators are more efficient than the naïve analysis of the internal data and other naïve combinations of external estimators.
更多查看译文
关键词
Data integration,Empirical Bayes,Meta-analysis,Prediction models
AI 理解论文
溯源树
样例
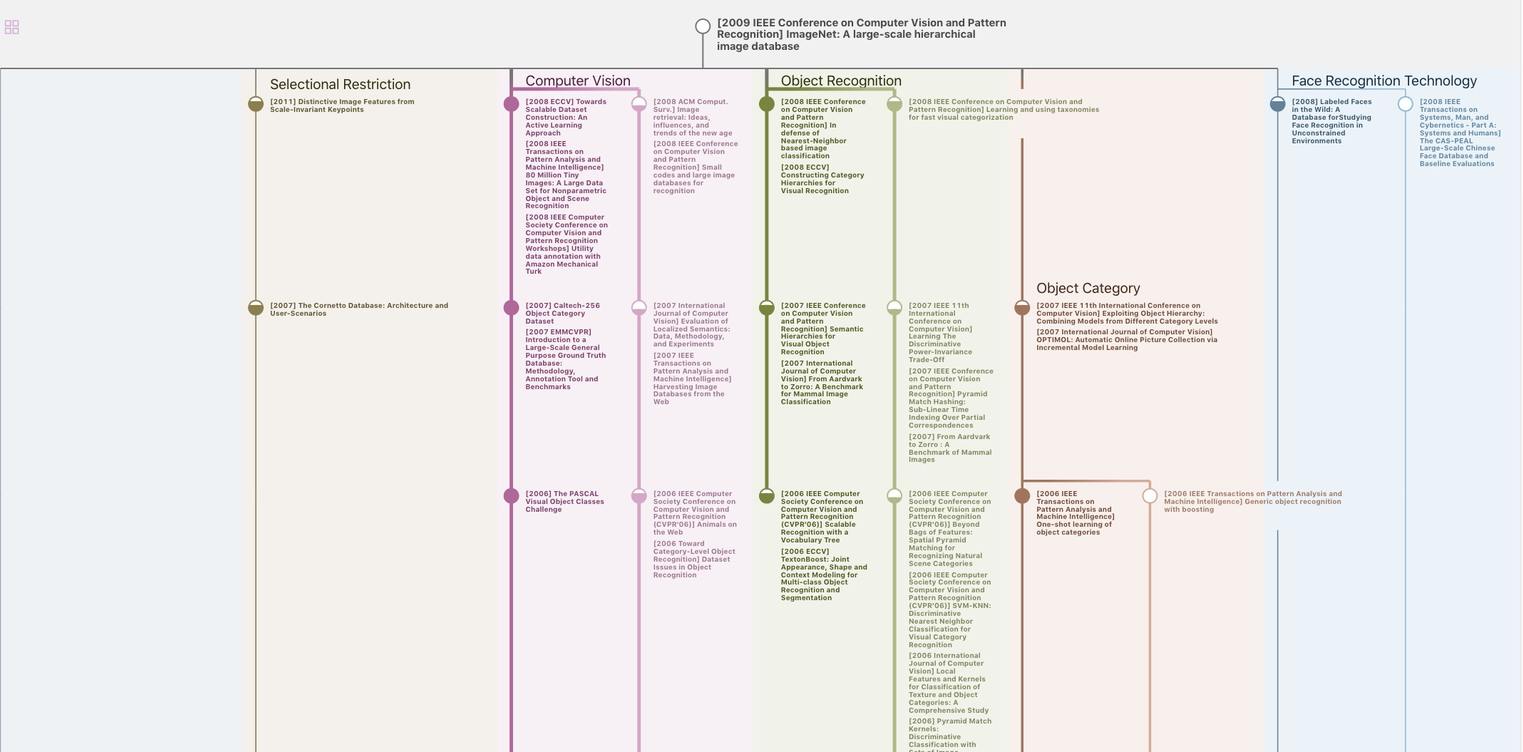
生成溯源树,研究论文发展脉络
Chat Paper
正在生成论文摘要