GopGAN: Gradients Orthogonal Projection Generative Adversarial Network With Continual Learning
IEEE Transactions on Neural Networks and Learning Systems(2023)
摘要
The generative adversarial networks (GANs) in continual learning suffer from catastrophic forgetting. In continual learning, GANs tend to forget about previous generation tasks and only remember the tasks they just learned. In this article, we present a novel conditional GAN, called the gradients orthogonal projection GAN (GopGAN), which updates the weights in the orthogonal subspace of the space spanned by the representations of training examples, and we also mathematically demonstrate its ability to retain the old knowledge about learned tasks in learning a new task. Furthermore, the orthogonal projection matrix for modulating gradients is mathematically derived and its iterative calculation algorithm for continual learning is given so that training examples for learned tasks do not need to be stored when learning a new task. In addition, a task-dependent latent vector construction is presented and the constructed conditional latent vectors are used as the inputs of generator in GopGAN to avoid the disappearance of orthogonal subspace of learned tasks. Extensive experiments on MNIST, EMNIST, SVHN, CIFAR10, and ImageNet-200 generation tasks show that the proposed GopGAN can effectively cope with the issue of catastrophic forgetting and stably retain learned knowledge.
更多查看译文
关键词
Catastrophic forgetting,continual learning,generative adversarial networks (GANs),orthogonal projection matrix
AI 理解论文
溯源树
样例
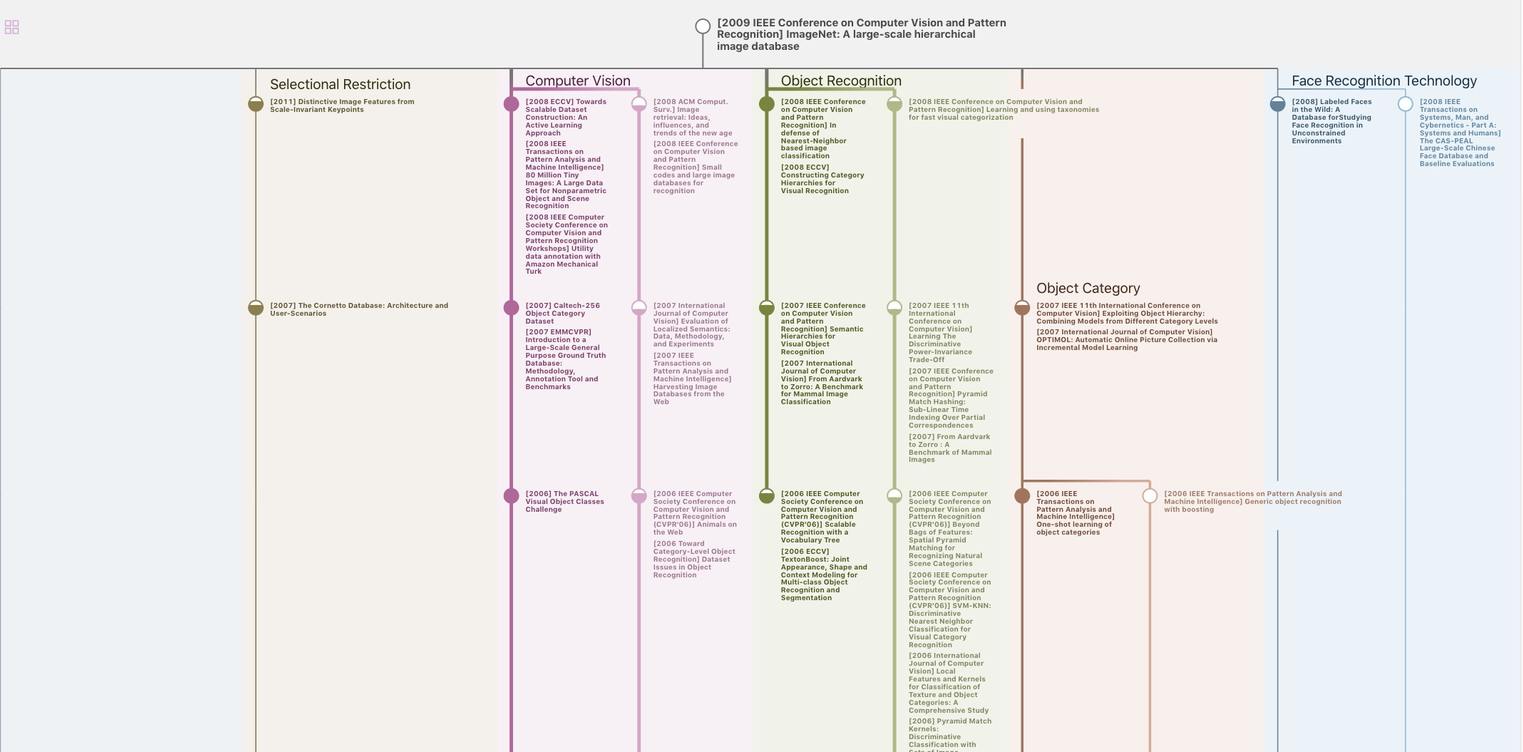
生成溯源树,研究论文发展脉络
Chat Paper
正在生成论文摘要