Robust Online Control with Model Misspecification
arxiv(2021)
摘要
We study online control of an unknown nonlinear dynamical system that is approximated by a time-invariant linear system with model misspecification. Our study focuses on robustness, which measures how much deviation from the assumed linear approximation can be tolerated while maintaining a bounded $\ell_2$-gain compared to the optimal control in hindsight. Some models cannot be stabilized even with perfect knowledge of their coefficients: the robustness is limited by the minimal distance between the assumed dynamics and the set of unstabilizable dynamics. Therefore it is necessary to assume a lower bound on this distance. Under this assumption, and with full observation of the $d$ dimensional state, we describe an efficient controller that attains $\Omega(\frac{1}{\sqrt{d}})$ robustness together with an $\ell_2$-gain whose dimension dependence is near optimal. We also give an inefficient algorithm that attains constant robustness independent of the dimension, with a finite but sub-optimal $\ell_2$-gain.
更多查看译文
关键词
robust online control,model
AI 理解论文
溯源树
样例
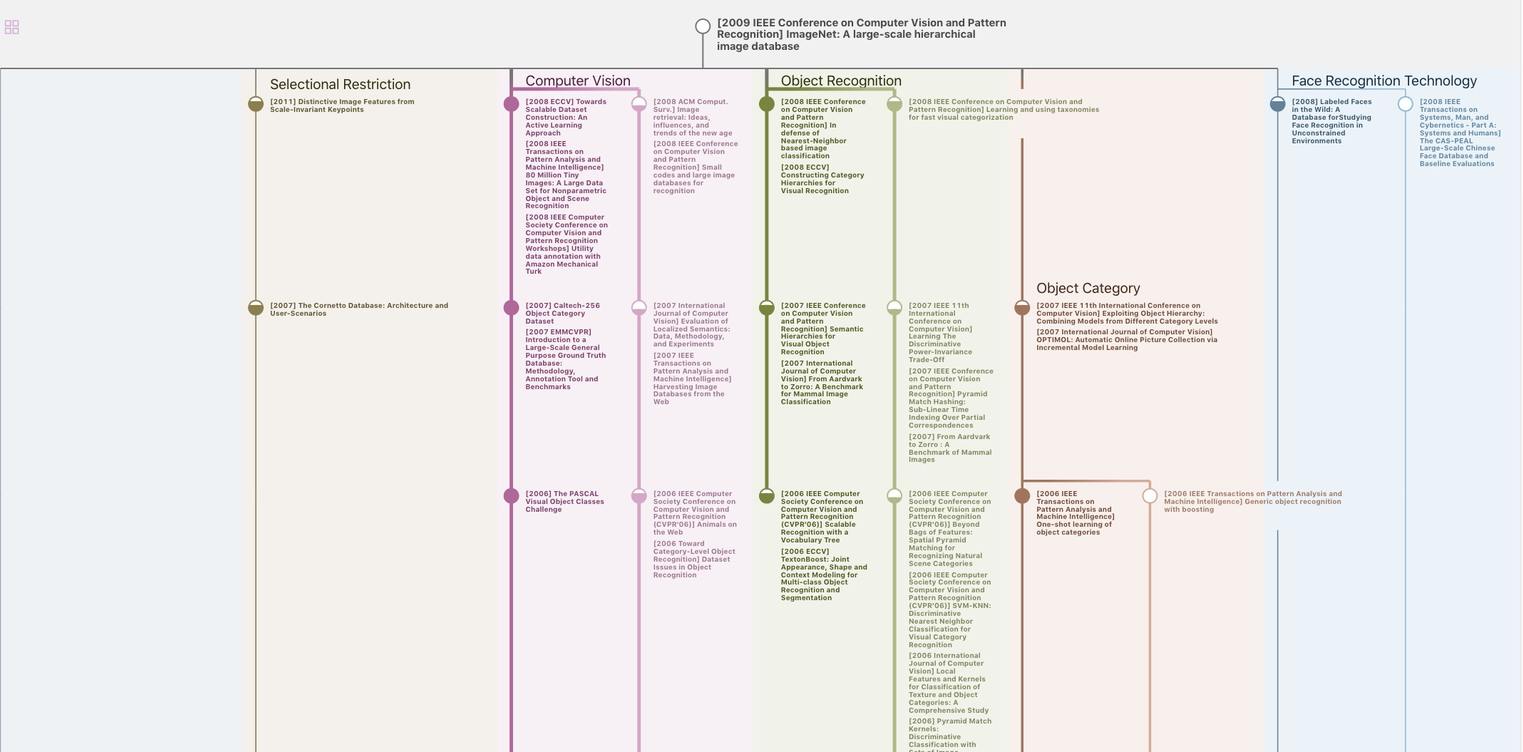
生成溯源树,研究论文发展脉络
Chat Paper
正在生成论文摘要