Inverse Problem of Nonlinear Schrödinger Equation as Learning of Convolutional Neural Network
arxiv(2021)
摘要
In this work, we use an explainable convolutional neural network (NLS-Net) to solve an inverse problem of the nonlinear Schrödinger equation, which is widely used in fiber-optic communications. The landscape and minimizers of the non-convex loss function of the learning problem are studied empirically. It provides a guidance for choosing hyper-parameters of the method. The estimation error of the optimal solution is discussed in terms of expressive power of the NLS-Net and data. Besides, we compare the performance of several training algorithms that are popular in deep learning. It is shown that one can obtain a relatively accurate estimate of the considered parameters using the proposed method. The study provides a natural framework of solving inverse problems of nonlinear partial differential equations with deep learning. Keywords— Inverse Problem, Nonlinear Schrödinger Equation, Operator Splitting, Deep Neural Network, Differentiable Programming, Explainable Artificial Intelligence AMS Mathematics Subject Classifications [2020]: 65M32, 78A46, 68T07, 78M32, 35R30
更多查看译文
关键词
nonlinear schrödinger equation,convolutional neural network,neural network,learning
AI 理解论文
溯源树
样例
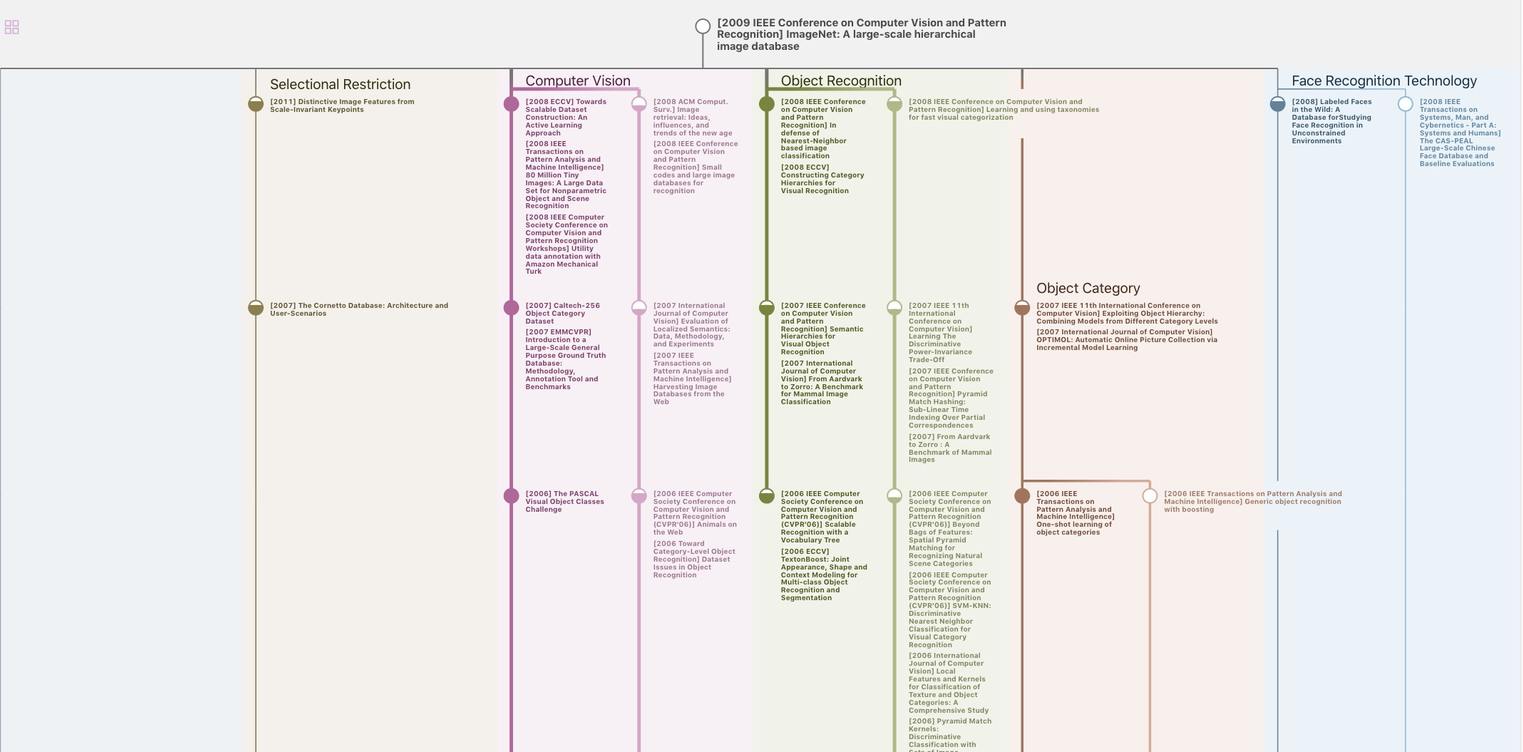
生成溯源树,研究论文发展脉络
Chat Paper
正在生成论文摘要