Topological Attention for Time Series Forecasting.
Annual Conference on Neural Information Processing Systems(2021)
摘要
The problem of (point) forecasting univariate time series is considered. Most approaches, ranging from traditional statistical methods to recent learning-based techniques with neural networks, directly operate on raw time series observations. As an extension, we study whether local topological properties, as captured via persistent homology, can serve as a reliable signal that provides complementary information for learning to forecast. To this end, we propose topological attention, which allows attending to local topological features within a time horizon of historical data. Our approach easily integrates into existing end-to-end trainable forecasting models, such as N-BEATS, and, in combination with the latter exhibits state-of-the-art performance on the large-scale M4 benchmark dataset of 100,000 diverse time series from different domains. Ablation experiments, as well as a comparison to recent techniques in a setting where only a single time series is available for training, corroborate the beneficial nature of including local topological information through an attention mechanism.
更多查看译文
关键词
time series forecasting,attention
AI 理解论文
溯源树
样例
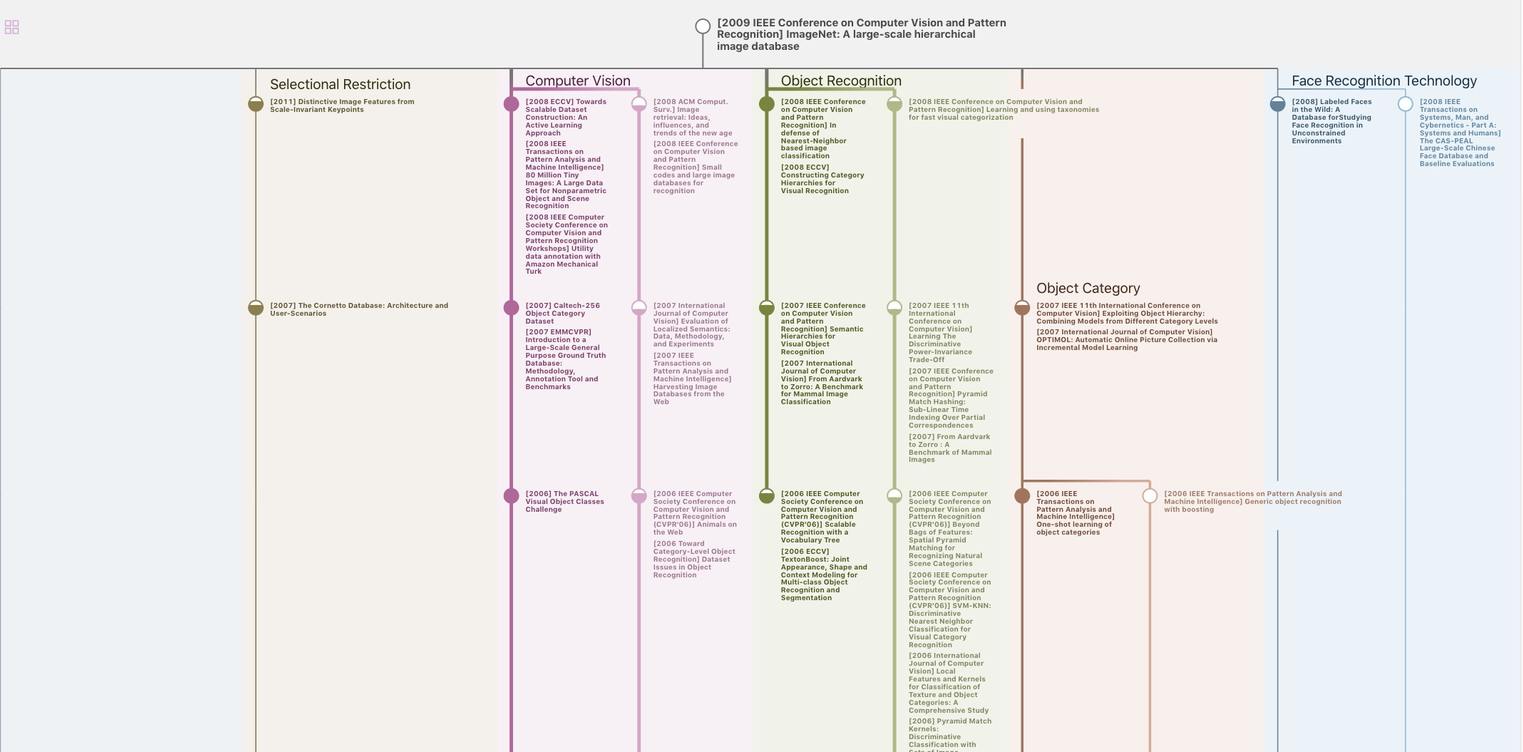
生成溯源树,研究论文发展脉络
Chat Paper
正在生成论文摘要