Machine Learning for Predicting Risk of Drug-Induced Autoimmune Diseases by Structural Alerts and Daily Dose
International journal of environmental research and public health/International journal of environmental research and public health(2021)
摘要
An effective approach for assessing a drug’s potential to induce autoimmune diseases (ADs) is needed in drug development. Here, we aim to develop a workflow to examine the association between structural alerts and drugs-induced ADs to improve toxicological prescreening tools. Considering reactive metabolite (RM) formation as a well-documented mechanism for drug-induced ADs, we investigated whether the presence of certain RM-related structural alerts was predictive for the risk of drug-induced AD. We constructed a database containing 171 RM-related structural alerts, generated a dataset of 407 AD- and non-AD-associated drugs, and performed statistical analysis. The nitrogen-containing benzene substituent alerts were found to be significantly associated with the risk of drug-induced ADs (odds ratio = 2.95, p = 0.0036). Furthermore, we developed a machine-learning-based predictive model by using daily dose and nitrogen-containing benzene substituent alerts as the top inputs and achieved the predictive performance of area under curve (AUC) of 70%. Additionally, we confirmed the reactivity of the nitrogen-containing benzene substituent aniline and related metabolites using quantum chemistry analysis and explored the underlying mechanisms. These identified structural alerts could be helpful in identifying drug candidates that carry a potential risk of drug-induced ADs to improve their safety profiles.
更多查看译文
关键词
drug-induced autoimmune diseases,structural alerts,machine learning,quantum chemistry
AI 理解论文
溯源树
样例
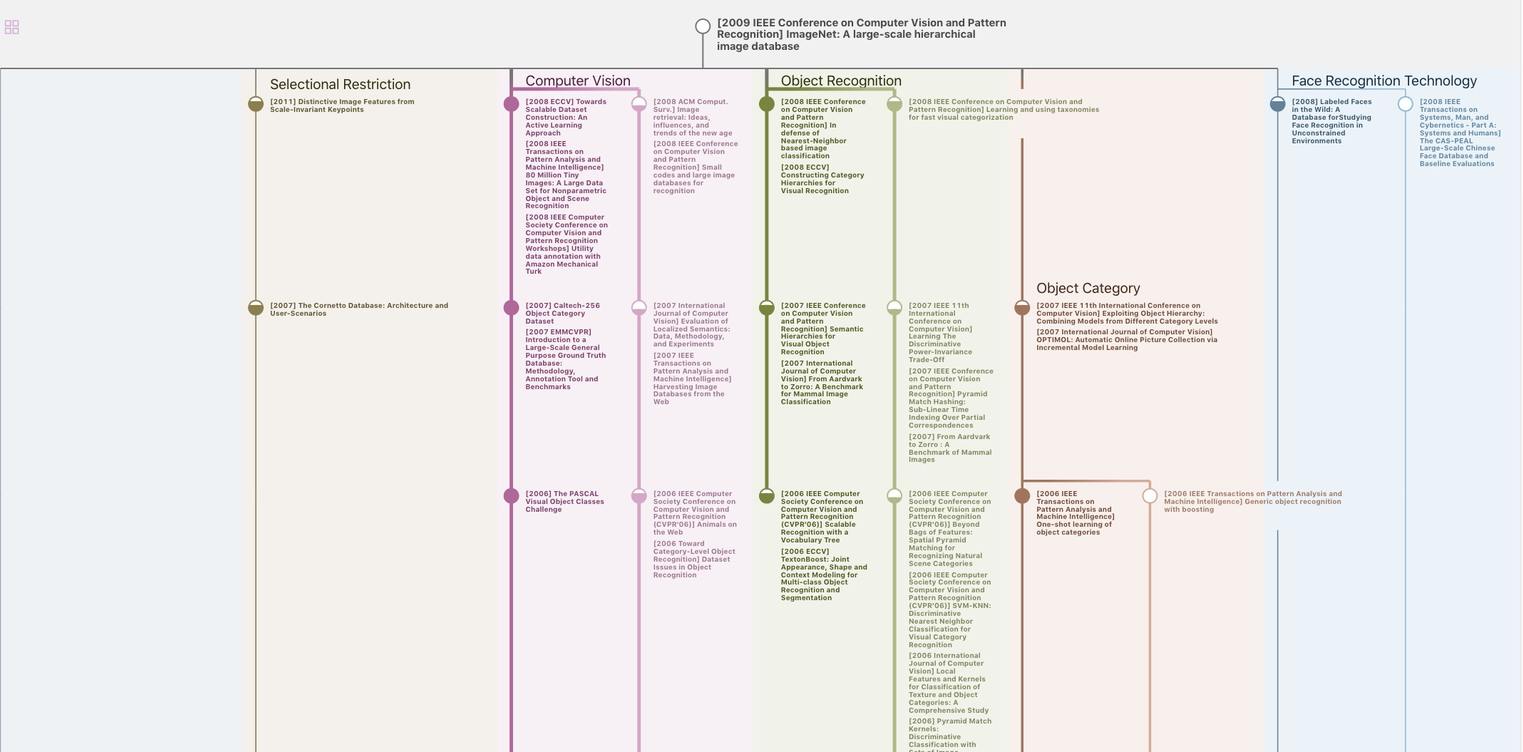
生成溯源树,研究论文发展脉络
Chat Paper
正在生成论文摘要