Stein Variational Gradient Descent with Multiple Kernels
arxiv(2022)
摘要
Bayesian inference is an important research area in cognitive computation due to its ability to reason under uncertainty in machine learning. As a representative algorithm, Stein variational gradient descent (SVGD) and its variants have shown promising successes in approximate inference for complex distributions. In practice, we notice that the kernel used in SVGD-based methods has a decisive effect on the empirical performance. Radial basis function (RBF) kernel with median heuristics is a common choice in previous approaches, but unfortunately, this has proven to be sub-optimal. Inspired by the paradigm of Multiple Kernel Learning (MKL), our solution to this flaw is using a combination of multiple kernels to approximate the optimal kernel, rather than a single one which may limit the performance and flexibility. Specifically, we first extend Kernelized Stein Discrepancy (KSD) to its multiple kernels view called Multiple Kernelized Stein Discrepancy (MKSD) and then leverage MKSD to construct a general algorithm Multiple Kernel SVGD (MK-SVGD). Further, MK-SVGD can automatically assign a weight to each kernel without any other parameters, which means that our method not only gets rid of optimal kernel dependence but also maintains computational efficiency. Experiments on various tasks and models demonstrate that our proposed method consistently matches or outperforms the competing methods.
更多查看译文
关键词
Bayesian inference,Approximate inference,SVGD,Multiple Kernel Learning,Stein Methods
AI 理解论文
溯源树
样例
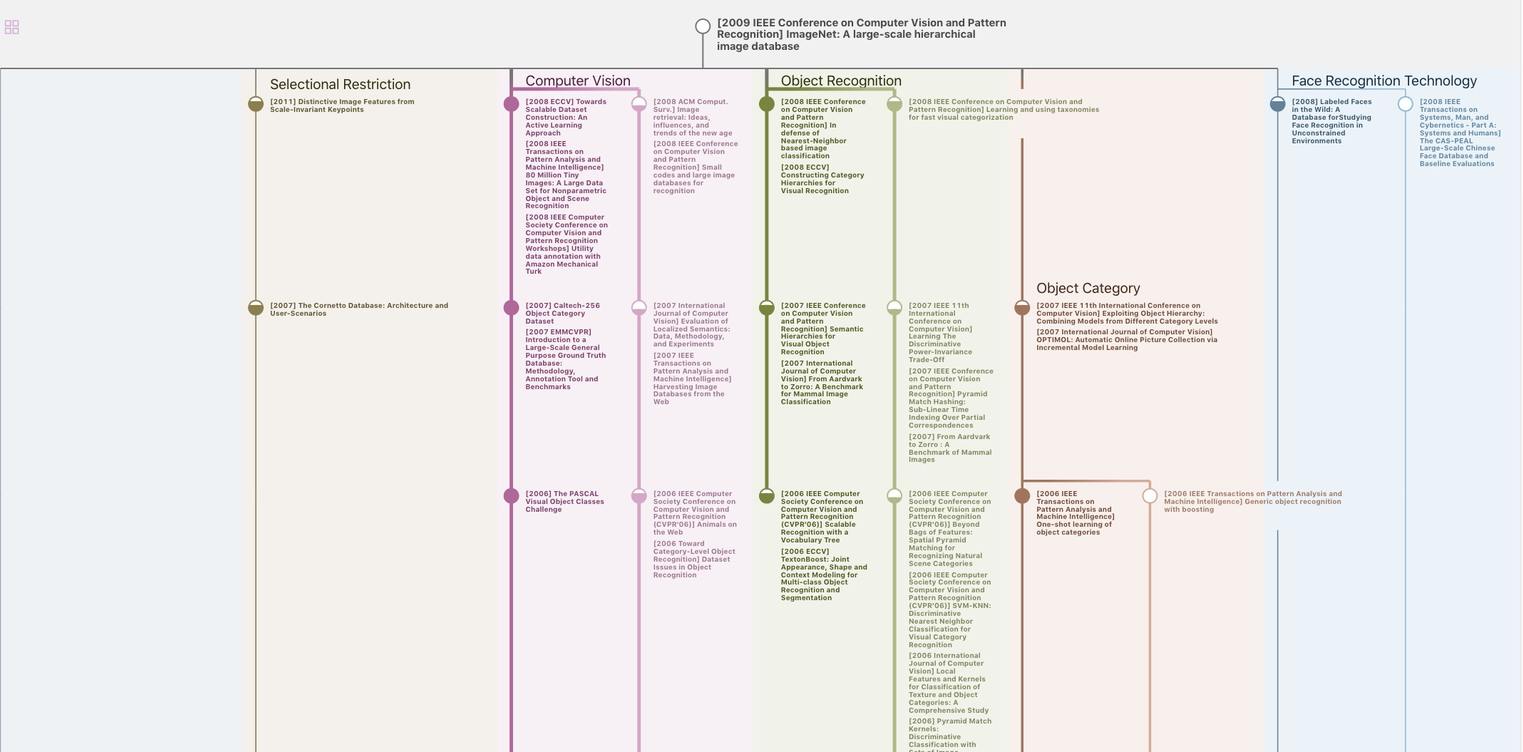
生成溯源树,研究论文发展脉络
Chat Paper
正在生成论文摘要