Soft Layer Selection With Meta-Learning For Zero-Shot Cross-Lingual Transfer
1ST WORKSHOP ON META LEARNING AND ITS APPLICATIONS TO NATURAL LANGUAGE PROCESSING (METANLP 2021)(2021)
摘要
Multilingual pre-trained contextual embedding models (Devlin et al., 2019) have achieved impressive performance on zero-shot cross-lingual transfer tasks. Finding the most effective strategy to fine-tune these models on high-resource languages so that it transfers well to the zero-shot languages is a nontrivial task. In this paper, we propose a novel meta-optimizer to soft-select which layers of the pre-trained model to freeze during fine-tuning. We train the meta-optimizer by simulating the zero-shot transfer scenario. Results on cross-lingual natural language inference show that our approach improves over the simple fine-tuning baseline and X-MAML (Nooralahzadeh et al., 2020).
更多查看译文
关键词
transfer,selection,meta-learning,zero-shot,cross-lingual
AI 理解论文
溯源树
样例
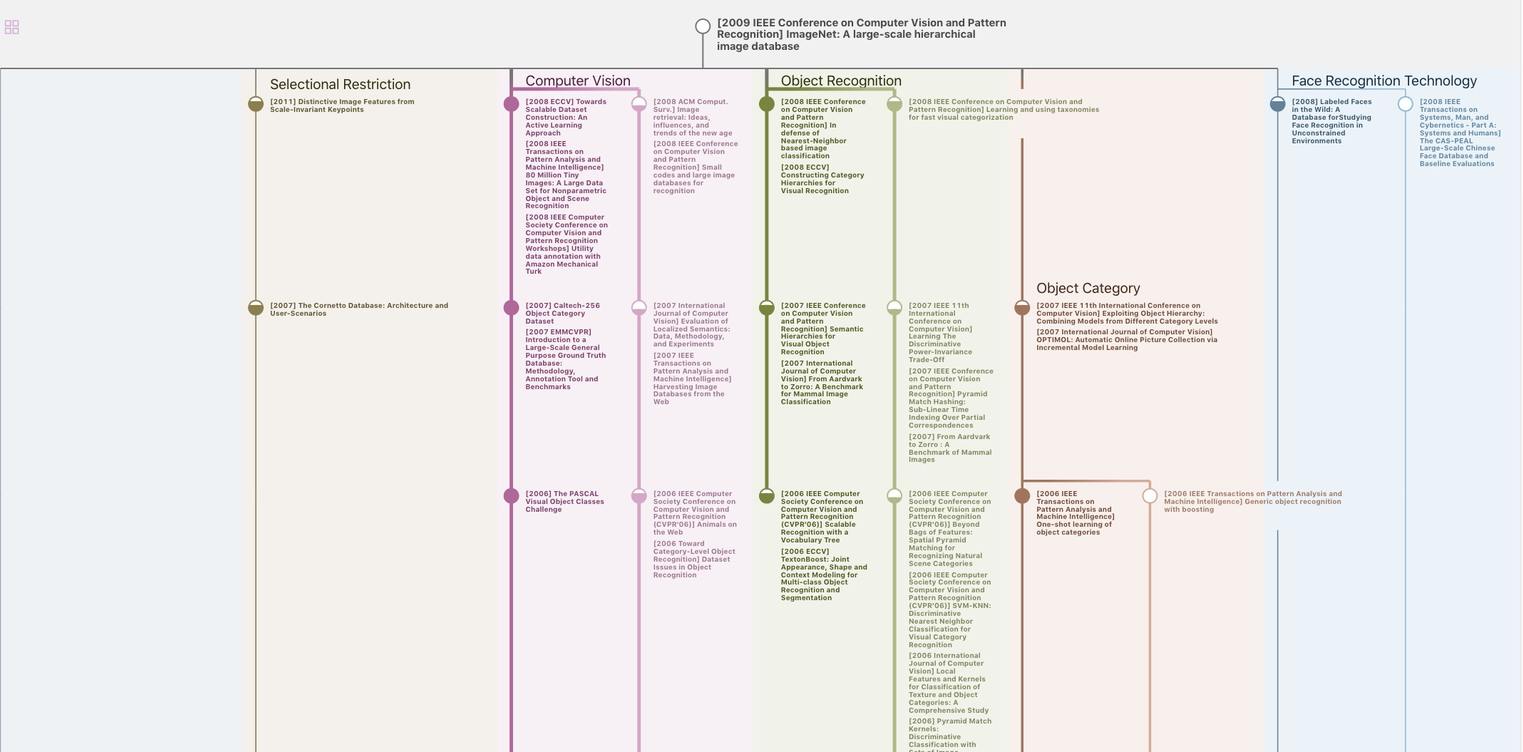
生成溯源树,研究论文发展脉络
Chat Paper
正在生成论文摘要